M22: Rate-Distortion Inspired Gradient Compression
ICASSP 2023 - 2023 IEEE International Conference on Acoustics, Speech and Signal Processing (ICASSP)(2023)
Key words
Federated learning,Gradient compression,Gradient sparsification,DNN gradient modeling
AI Read Science
Must-Reading Tree
Example
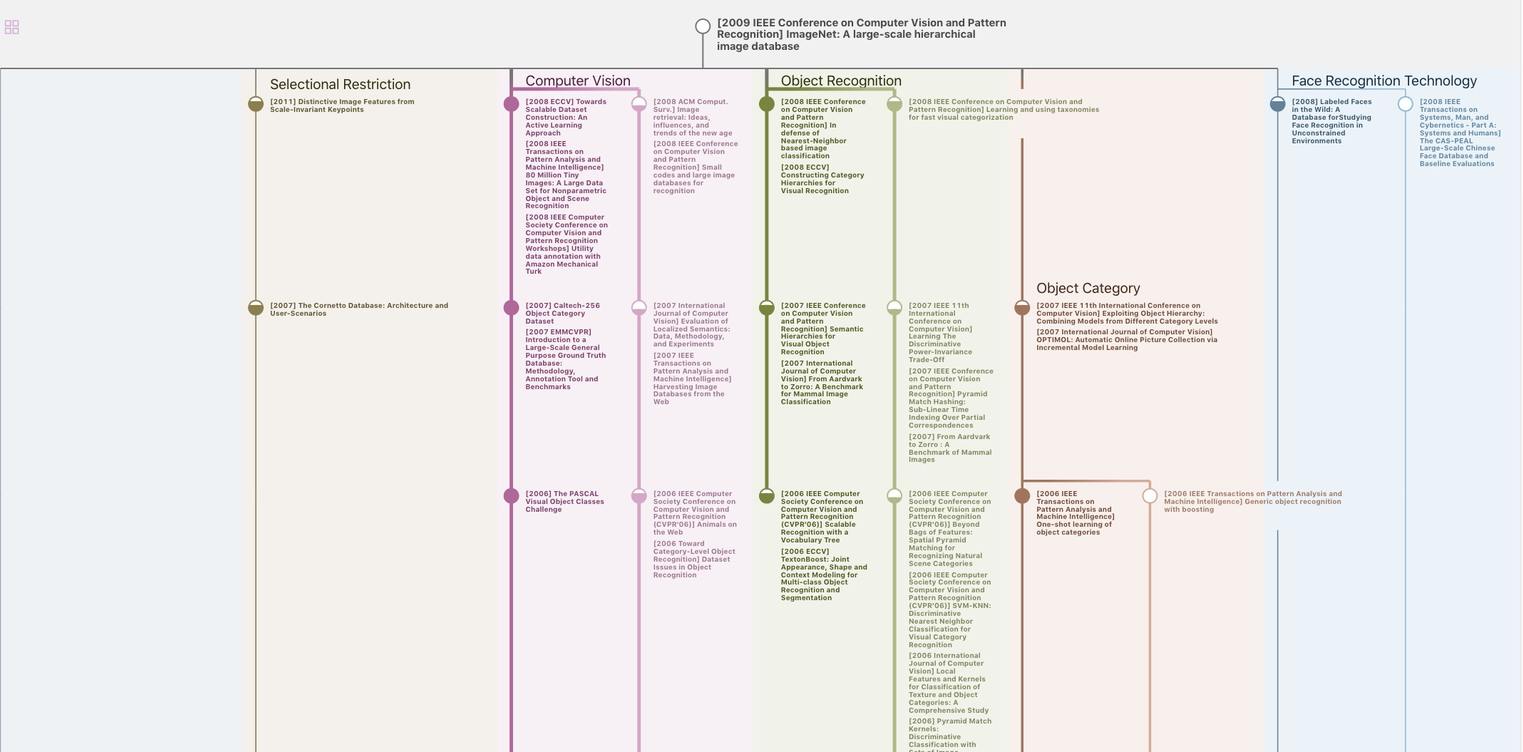
Generate MRT to find the research sequence of this paper
Chat Paper
Summary is being generated by the instructions you defined