Improved Small Sample Hypothesis Testing Using the Uncertain Likelihood Ratio
ICASSP 2023 - 2023 IEEE International Conference on Acoustics, Speech and Signal Processing (ICASSP)(2023)
摘要
This paper studies the problem of event detection in highly dynamic and uncertain environments where training data is limited and the number of observations is small. The objective is to determine if the training data and observations are drawn from the same or different distributions. The traditional approach is to use the Generalized Likelihood Ratio (GLR) test statistic. However, with limited training data and observations, the GLR is suboptimal. To overcome this, we propose to use the Uncertain Likelihood Ratio (ULR) test statistic to accurately account for the uncertainty. We show that the ULR is the mean most powerful test and that it is asymptotically equivalent to the GLR under a Jeffreys Prior. Furthermore, a simulation study validates the theory that the performance of the ULR is superior to the GLR when testing in the small sample regime.
更多查看译文
关键词
generalized likelihood ratio test statistic,GLR,small sample hypothesis,test statistic,training data,ULR,uncertain environments,uncertain likelihood ratio test
AI 理解论文
溯源树
样例
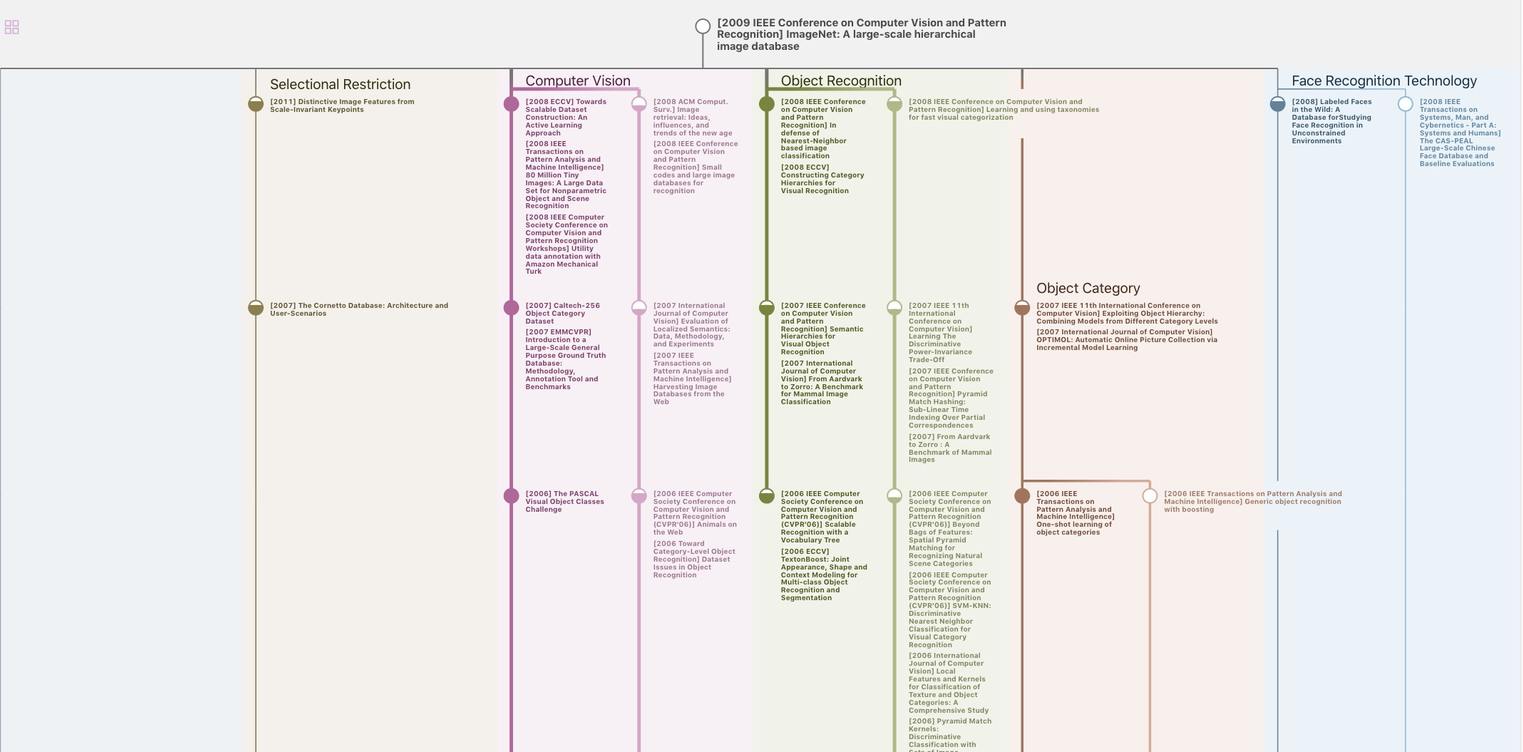
生成溯源树,研究论文发展脉络
Chat Paper
正在生成论文摘要