Dual-Stage Graph Convolution Network With Graph Learning For Traffic Prediction
ICASSP 2023 - 2023 IEEE International Conference on Acoustics, Speech and Signal Processing (ICASSP)(2023)
摘要
Robust and accurate traffic forecasting is a key issue in intelligent transportation systems. Existing studies usually employ pre-defined spatial graph or learned fixed adjacency graph and design models to capture spatial and temporal features. However, pre-defined or fixed graph can not accurately model the complex hidden structure. Moreover, few solutions are satisfied with both long and short-term prediction tasks. In this paper, we propose a novel dual-stage graph convolution network based on graph learning (DSGCN) to address these challenges. To equip the graph convolution network with a flexible and practical graph structure, DSGCN designs a graph learning module to model the varying relations among nodes in the road network. In particular, we first provide a hierarchical graph structure cooperated with the dilated convolution to capture the temporal dependencies. Second, a dual-stage graph convolution layer is proposed to capture the complex spatial dependencies. Experiments on two real-world datasets demonstrate that DSGCN outperforms the state-of-the-art baselines, especially for long-term traffic prediction.
更多查看译文
关键词
Traffic prediction,dynamic graph learning,temporal convolution,graph convolution
AI 理解论文
溯源树
样例
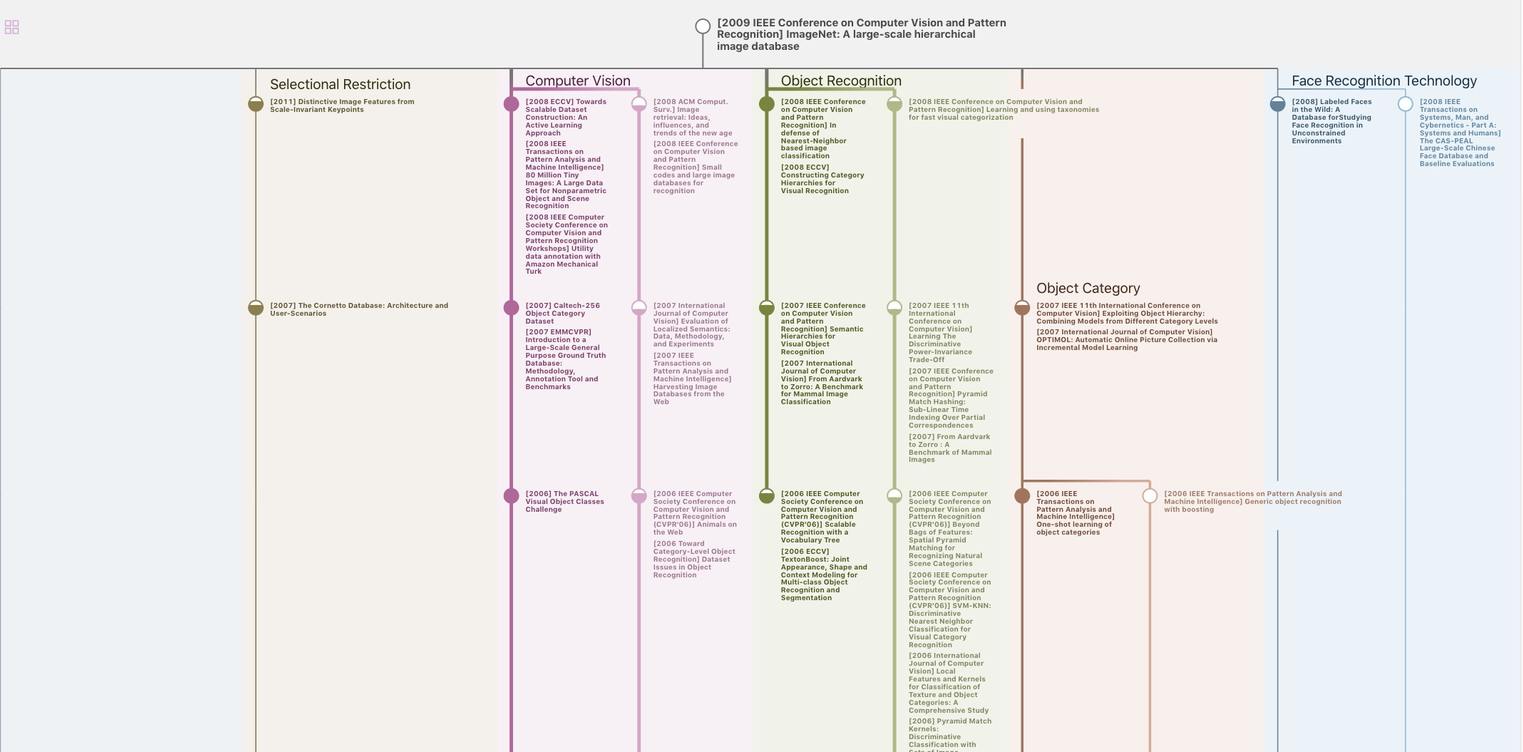
生成溯源树,研究论文发展脉络
Chat Paper
正在生成论文摘要