Cross-Subject Mental Fatigue Detection based on Separable Spatio-Temporal Feature Aggregation
ICASSP 2023 - 2023 IEEE International Conference on Acoustics, Speech and Signal Processing (ICASSP)(2023)
摘要
Cross-subject mental fatigue detection via Electroencephalography (EEG) is challenging because EEG from different individuals varies greatly. Existing works have exploited domain adaption to alleviate the individual discrepancy due to personality, gender and so on. However, the distributions of data from new subjects and old ones are aligned by deceiving the domain discriminator. An inevitable issue of such a paradigm is that the samples near the decision boundary are easy to be misclassified. To address this issue, we propose a Separable Spatio-temporal Feature Aggregation (SSFA) that consists of a Spatio-temporal Feature Extractor (SFE) and a Separable Feature Aggregation mechanism (SFA). Specifically, SFE utilizes the spatio-temporal information in EEG and automatically tune the weights of temporal and spatial features, so as to update the model along the optimal direction and obtain more discriminative features. In addition, SFA employs two classifiers combined with sliced Wasserstein Discrepancy to aggregate each separate class together, facilitating the mapping of the new subjects to the support region of the old subjects. Leave-one-subject-out experiments conducted on a public fatigue dataset show that the proposed method performs better than state-of-the-art on many evaluation metrics especially with an accuracy of 85.91%.
更多查看译文
关键词
Mental fatigue detection,Domain adaptation,EEG,Wasserstein discrepancy
AI 理解论文
溯源树
样例
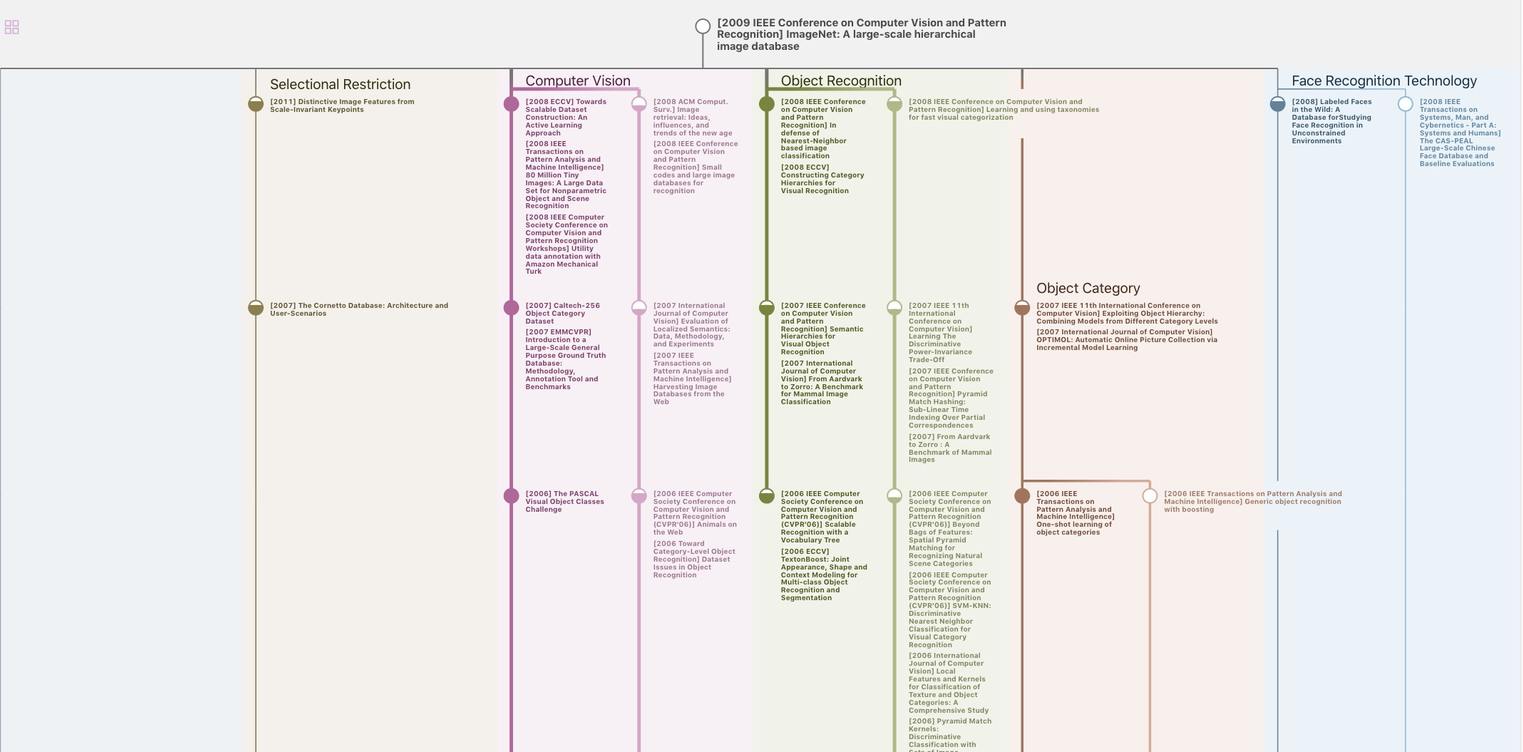
生成溯源树,研究论文发展脉络
Chat Paper
正在生成论文摘要