Relapse Prediction from Long-Term Wearable Data Using Self-Supervised Learning and Survival Analysis
ICASSP 2023 - 2023 IEEE International Conference on Acoustics, Speech and Signal Processing (ICASSP)(2023)
摘要
The introduction of biometric signal analysis in psychiatry could potentially reshape the field by making it more accurate, proactive and personalized. Such biosignals usually acquired from wearables encompass the quantification of human behavior and traits. In this study, we use long-term data acquired from commercial smartwatches, including kinetic and physiological signals, to extract information-thick descriptors that are used for the prediction of subsequent relapses in patients in the psychotic spectrum. Specifically, we propose a novel combination of methods based on Self-Supervised Learning and Survival Analysis that operates on unlabeled and censored data. When combined with other static features that describe the past course of the patient’s health, the proposed methodology yields promising predictive results in terms of two standard survival analysis metrics.
更多查看译文
关键词
Self-Supervised Learning,Psychotic Disorders,Smartwatch Wearables,Survival Analysis,Relapse Prediction
AI 理解论文
溯源树
样例
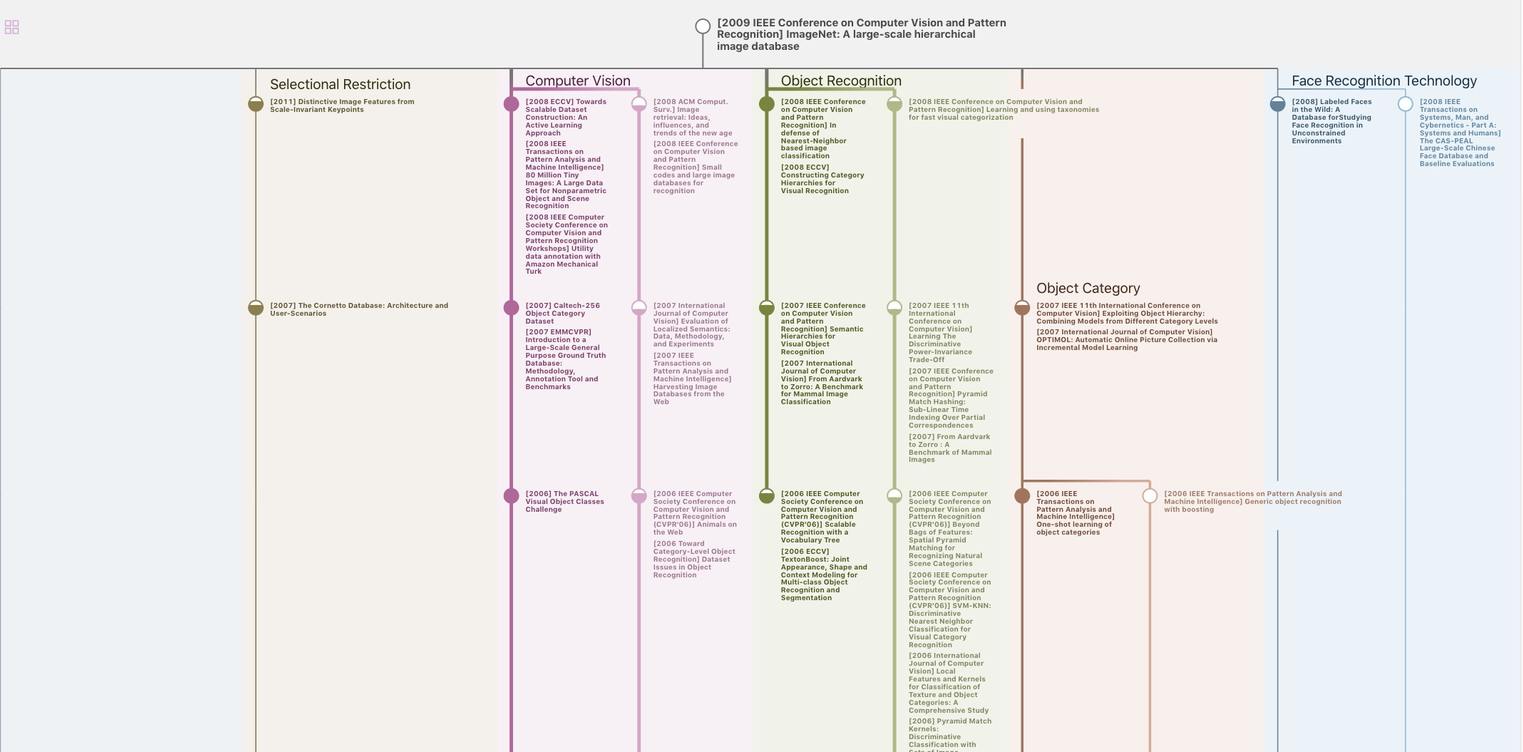
生成溯源树,研究论文发展脉络
Chat Paper
正在生成论文摘要