On the Quantization of Recurrent Neural Networks for Smiles Generation
ICASSP 2023 - 2023 IEEE International Conference on Acoustics, Speech and Signal Processing (ICASSP)(2023)
摘要
This paper focuses on the effects of applying quantization during training to Recurrent Neural Networks (RNNs) used in Simplified Molecular-Input Line-Entry System (SMILES) generation, a form of line notation for molecular information used in the development of pharmaceutical drugs, from the PubChem database. It offers the flexibility to choose the precision used by the model, by defining the number of bits at each layer. The RNNs are the focus of the current study, by comparing the performance of three of the most used algorithms, Simple RNN, Long Short-Term Memory (LSTM), and Gated Recurrent Unit (GRU). The models were trained on a selection of SMILES. By exploiting the QK-eras library, quantization performance was compared to their floating-point equivalent for several combinations of parameters. The goal of the testing program developed is to generate a large number of novel SMILES, facilitating the process of Drug Discovery which is traditionally long, and thus very expensive and difficult. By understanding how the behavior of quantized networks deviates from the regular model, in relation to the parameters used, we are able to control the process of choosing whether to quantize a model and to which degree it becomes more or less efficient. In this study, we observed good performance even for 4-bit models making use of LSTM and GRU layers, the same way we concluded that Simple RNN quantization does not compensate the effort.
更多查看译文
关键词
Recurrent Neural Networks,Long Short-Term Memory,Gated Recurrent Unit,Drug Discovery,Quantization
AI 理解论文
溯源树
样例
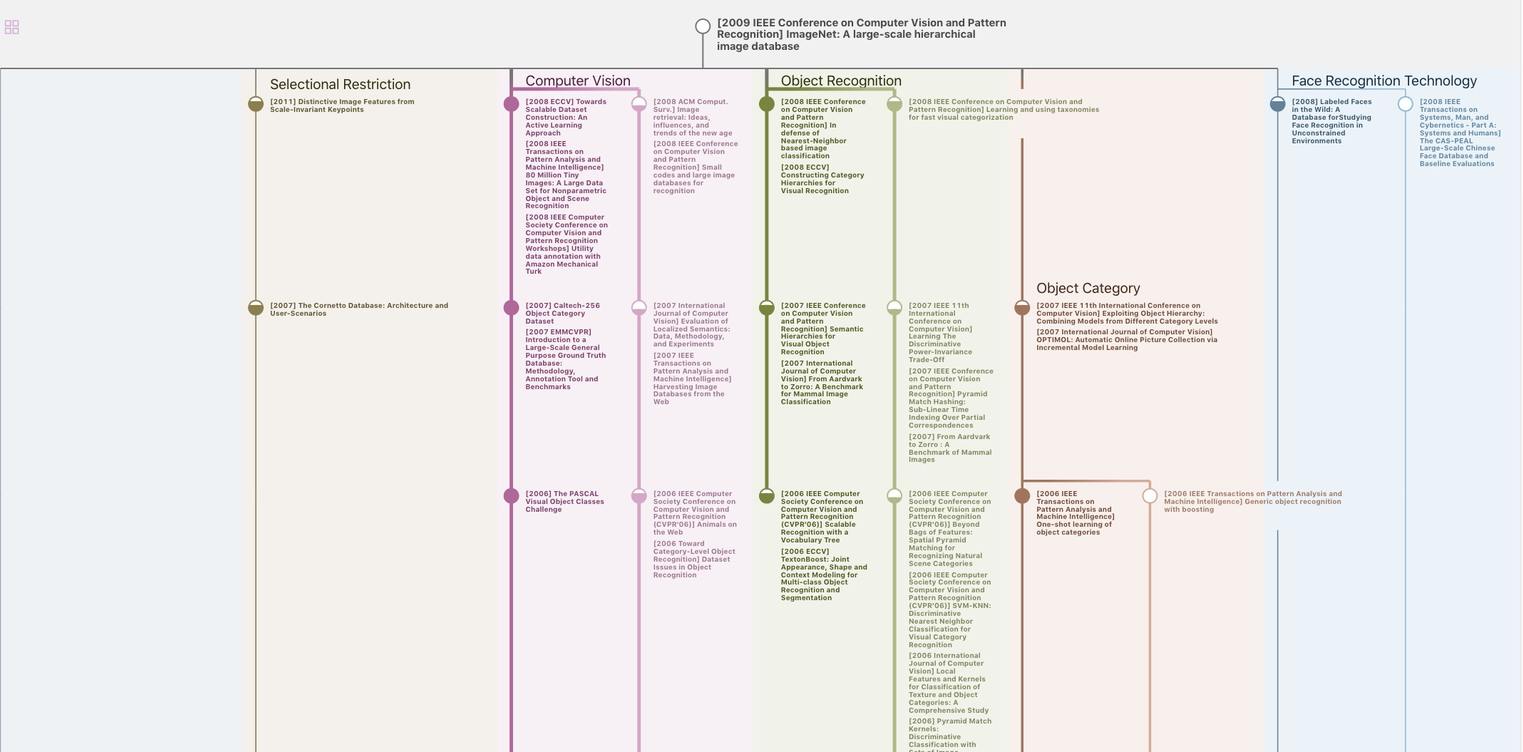
生成溯源树,研究论文发展脉络
Chat Paper
正在生成论文摘要