Graph-Graph Context Dependency Attention for Graph Edit Distance
ICASSP 2023 - 2023 IEEE International Conference on Acoustics, Speech and Signal Processing (ICASSP)(2023)
摘要
As a popular similarity measurement, Graph Edit Distance (GED) can be applied to various downstream tasks. Traditional GED solvers have difficulty in generalization and time efficiency, so deep graph similarity learning models have emerged as a promising trend. However, existing methods lack distinguishing embeddings for cross-dependencies between graphs and intra-graph representations. In this paper, we propose a deep network architecture GED-CDA by introducing a graph-graph context dependency attention module to enhance embeddings. Our graph-graph context dependency attention module consists of a cross-attention layer and a self-attention layer, which can both learn the interactions between graph pairs and capture the connections within graphs. In addition, we also introduce a spectral encoding strategy to bring topological information of graphs into node representations. Extensive experimental results on three real-world graph datasets demonstrate the effectiveness of our proposed GED-CDA in the GED task.
更多查看译文
关键词
Graph Neural Networks,Graph Edit Distance,Graph Similarity Learning,Attention Mechanism
AI 理解论文
溯源树
样例
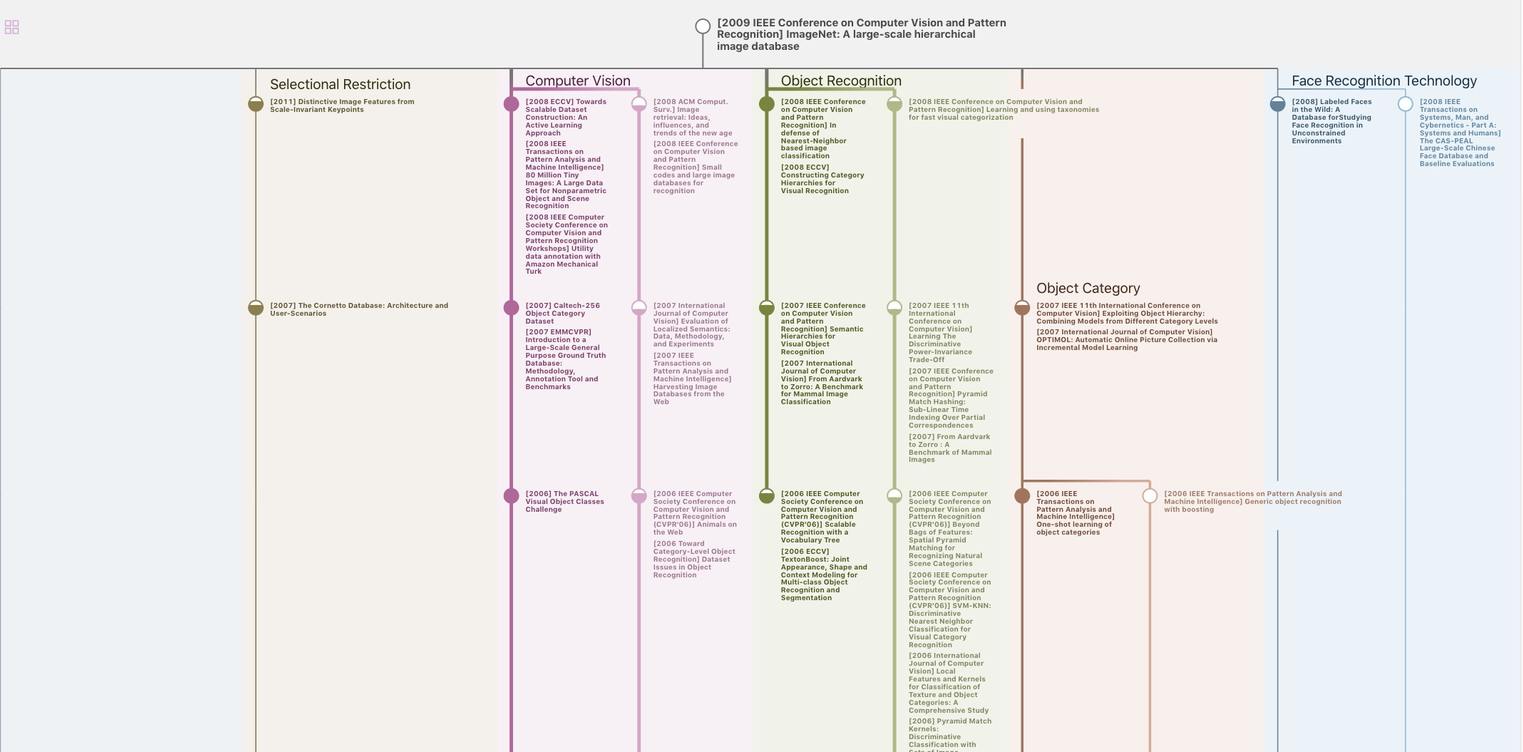
生成溯源树,研究论文发展脉络
Chat Paper
正在生成论文摘要