Meta++ Network for Few-Shot Aerospace Crack Segmentation
ICASSP 2023 - 2023 IEEE International Conference on Acoustics, Speech and Signal Processing (ICASSP)(2023)
摘要
The fatigue of riveted joints and bolts problem is extremely important in aircraft safety. In order to solve the problem of sample scarcity in the industrial field, this paper proposes a UNet++ based few-shot segmentation network Meta++ for aerospace metal structural component fatigue crack. The Meta++ consists of a base learner UNet++, a proxy extracting module, and a meta learner. Firstly, the base learner is trained by the semantic segmentation method. Secondly, we use the base learner predictions and ground truth masks to extract proxies. Thirdly, the meta learner is trained by the few-shot learning episode method, which uses the proxies and base learner intermediate features as inputs. The fatigue cracks are generated by a servohydraulic fatigue testing system and collated into a new aerospace crack dataset. Experiments on our proposed dataset demonstrate the superiority of Meta++ for the few-shot segmentation problem of aerostructures.
更多查看译文
关键词
Fatigue crack,few-shot,semantic segmentation,prototype learning
AI 理解论文
溯源树
样例
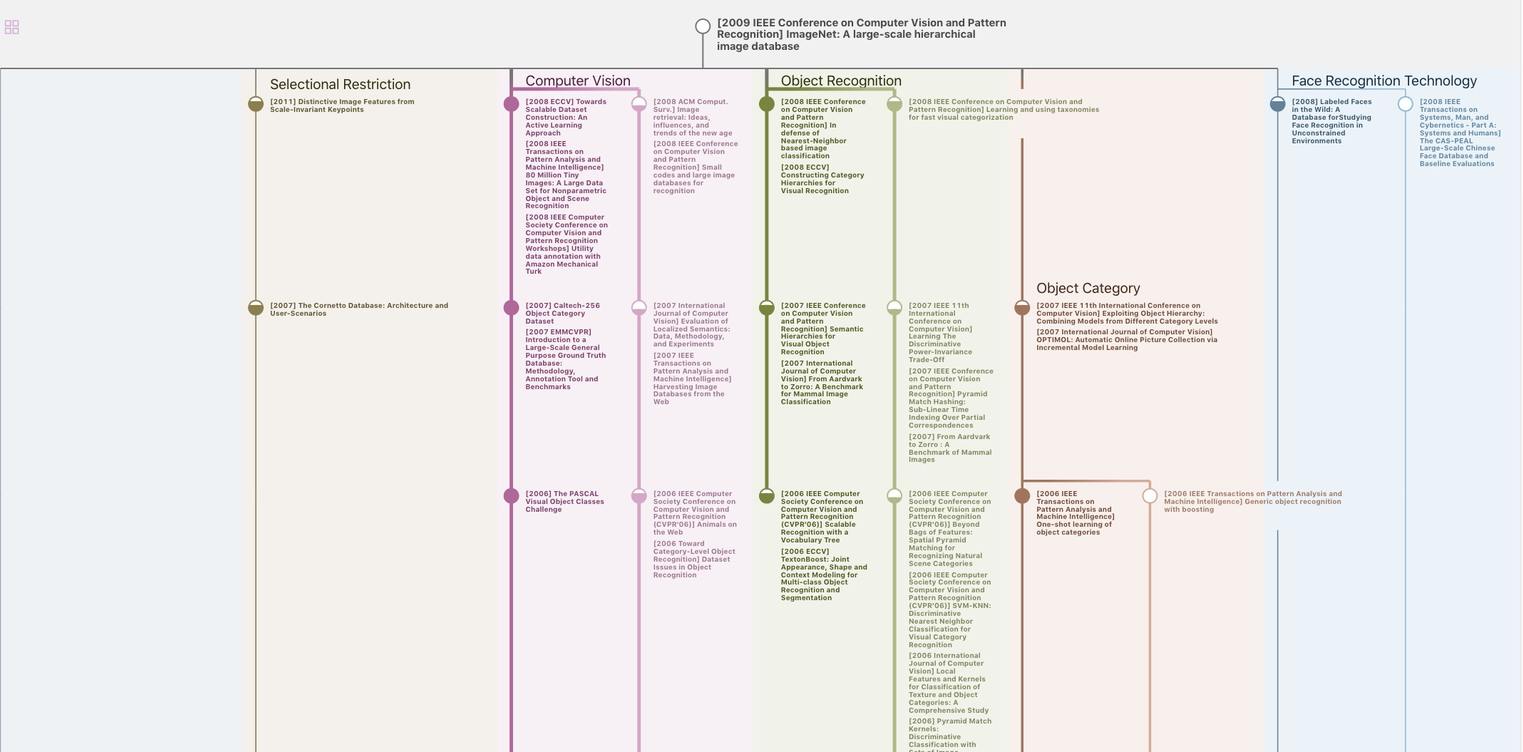
生成溯源树,研究论文发展脉络
Chat Paper
正在生成论文摘要