HandyPriors: Physically Consistent Perception of Hand-Object Interactions with Differentiable Priors
ICRA 2024(2024)
摘要
Various heuristic objectives for modeling hand-object interaction have been proposed in past work. However, due to the lack of a cohesive framework, these objectives often possess a narrow scope of applicability and are limited by their efficiency or accuracy. In this paper, we propose HandyPriors, a unified and general pipeline for pose estimation in human-object interaction scenes by leveraging recent advances in differentiable physics and rendering. Our approach employs rendering priors to align with input images and segmentation masks along with physics priors to mitigate penetration and relative-sliding across frames. Furthermore, we present two alternatives for hand and object pose estimation. The optimization-based pose estimation achieves higher accuracy, while the filtering-based tracking, which utilizes the differentiable priors as dynamics and observation models, executes faster. We demonstrate that HandyPriors attains comparable or superior results in the pose estimation task, and that the differentiable physics module can predict contact information for pose refinement. We also show that our approach generalizes to perception tasks, including robotic hand manipulation and human-object pose estimation in the wild.
更多查看译文
关键词
Object Detection, Segmentation and Categorization,Multifingered Hands
AI 理解论文
溯源树
样例
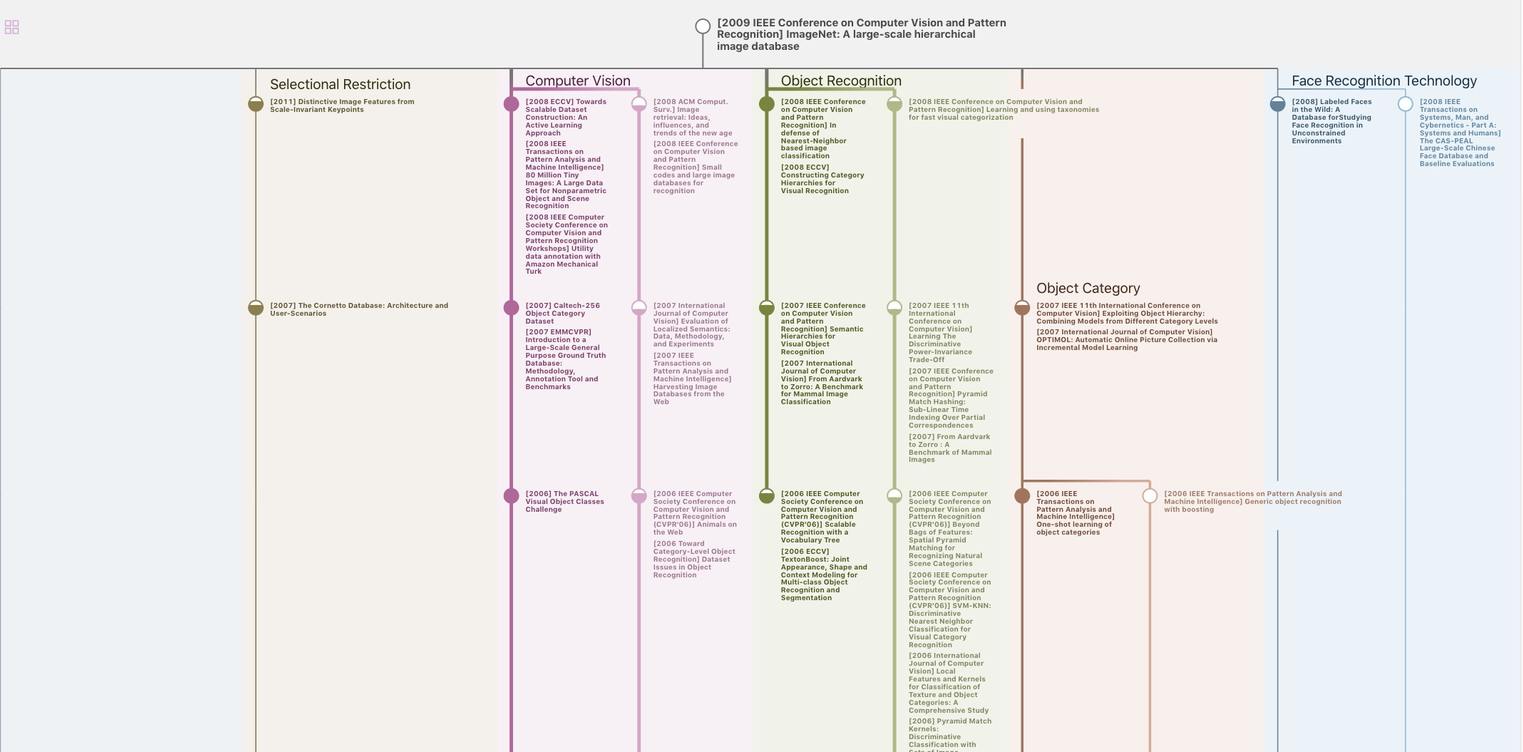
生成溯源树,研究论文发展脉络
Chat Paper
正在生成论文摘要