Scaling Political Texts with Large Language Models: Asking a Chatbot Might Be All You Need
arxiv(2023)
摘要
We use instruction-tuned Large Language Models (LLMs) such as GPT-4, MiXtral,
and Llama 3 to position political texts within policy and ideological spaces.
We directly ask the LLMs where a text document or its author stand on the focal
policy dimension. We illustrate and validate the approach by scaling British
party manifestos on the economic, social, and immigration policy dimensions;
speeches from a European Parliament debate in 10 languages on the anti- to
pro-subsidy dimension; Senators of the 117th US Congress based on their tweets
on the left-right ideological spectrum; and tweets published by US
Representatives and Senators after the training cutoff date of GPT-4. The
correlation between the position estimates obtained with the best LLMs and
benchmarks based on coding by experts, crowdworkers or roll call votes exceeds
.90. This training-free approach also outperforms supervised classifiers
trained on large amounts of data. Using instruction-tuned LLMs to scale texts
in policy and ideological spaces is fast, cost-efficient, reliable, and
reproducible (in the case of open LLMs) even if the texts are short and written
in different languages. We conclude with cautionary notes about the need for
empirical validation.
更多查看译文
AI 理解论文
溯源树
样例
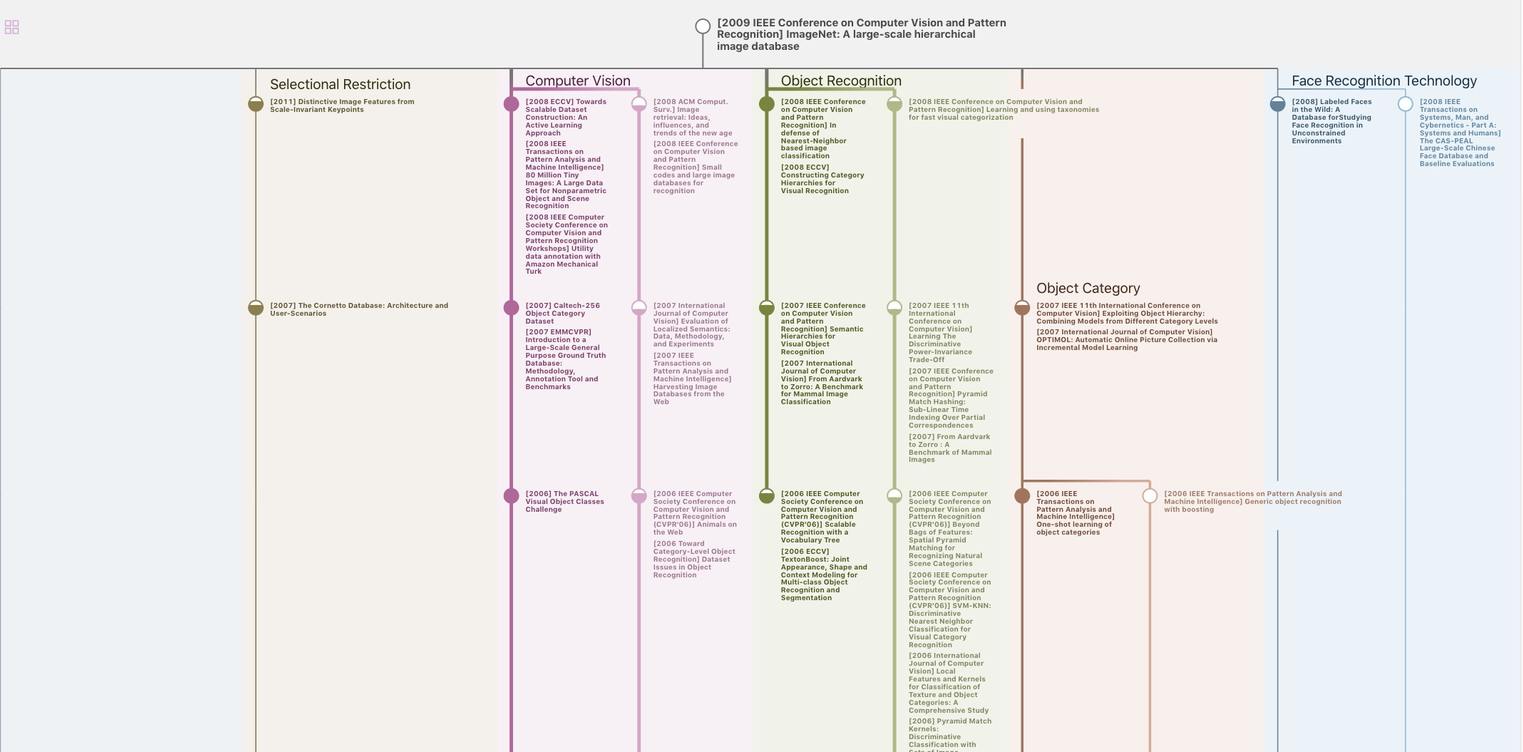
生成溯源树,研究论文发展脉络
Chat Paper
正在生成论文摘要