On the Impact of Sampling on Deep Sequential State Estimation
2023 57th Asilomar Conference on Signals, Systems, and Computers(2023)
摘要
State inference and parameter learning in sequential models can be
successfully performed with approximation techniques that maximize the evidence
lower bound to the marginal log-likelihood of the data distribution. These
methods may be referred to as Dynamical Variational Autoencoders, and our
specific focus lies on the deep Kalman filter. It has been shown that the ELBO
objective can oversimplify data representations, potentially compromising
estimation quality. Tighter Monte Carlo objectives have been proposed in the
literature to enhance generative modeling performance. For instance, the IWAE
objective uses importance weights to reduce the variance of marginal
log-likelihood estimates. In this paper, importance sampling is applied to the
DKF framework for learning deep Markov models, resulting in the IW-DKF, which
shows an improvement in terms of log-likelihood estimates and KL divergence
between the variational distribution and the transition model. The framework
using the sampled DKF update rule is also accommodated to address sequential
state and parameter estimation when working with highly non-linear
physics-based models. An experiment with the 3-space Lorenz attractor shows an
enhanced generative modeling performance and also a decrease in RMSE when
estimating the model parameters and latent states, indicating that tighter MCOs
lead to improved state inference performance.
更多查看译文
关键词
Importance sampling,variational inference,sequential state estimation,generative modeling
AI 理解论文
溯源树
样例
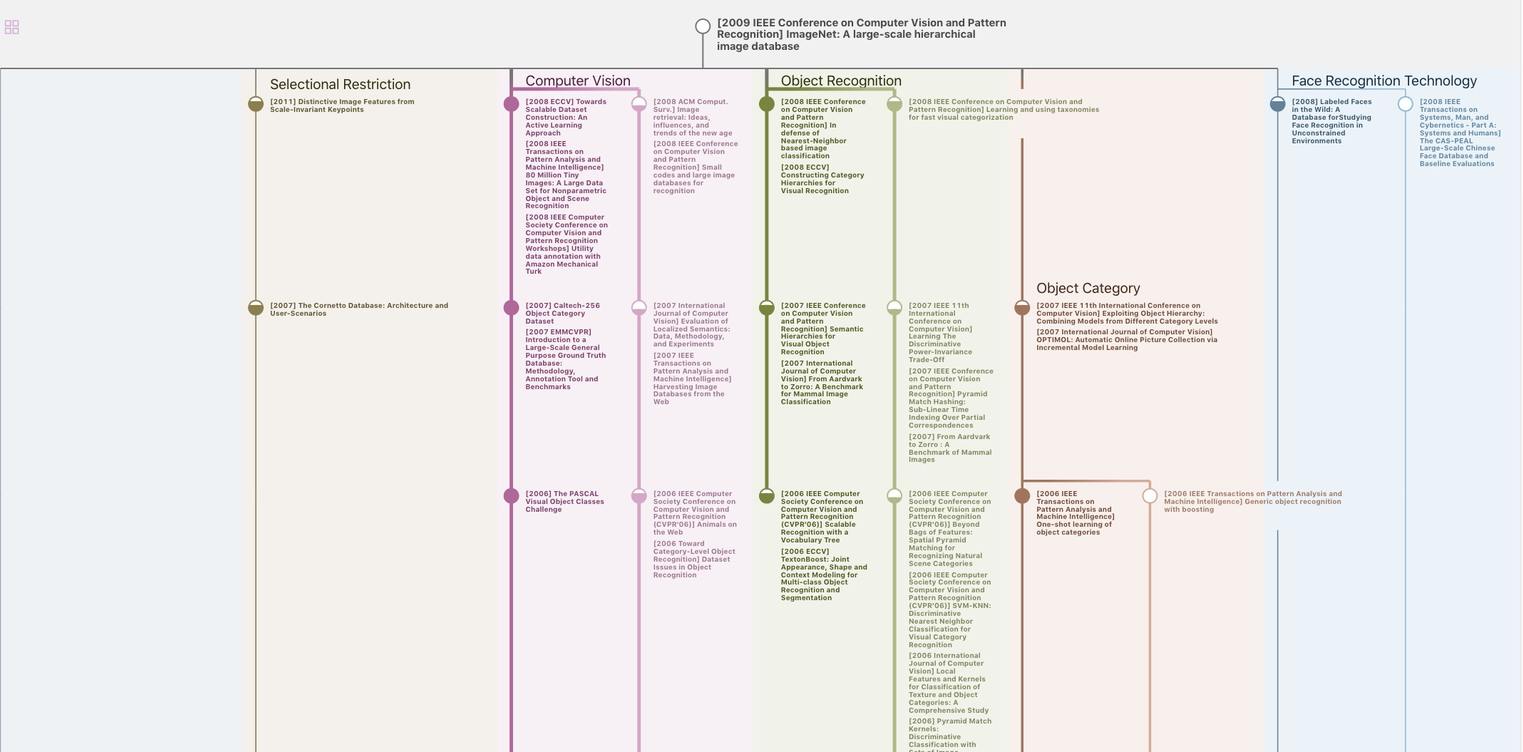
生成溯源树,研究论文发展脉络
Chat Paper
正在生成论文摘要