Fast Multi-ANN Composite Models for Repeater Optimization in Presence of Parametric Uncertainty for On-Chip Hybrid Copper-Graphene Interconnects
IEEE ACCESS(2023)
摘要
In this paper, composite models are developed to predict the statistics of the optimal number and size of repeaters required to minimize the power delay product (PDP) of on-chip hybrid copper-graphene interconnects when subject to parametric uncertainty. Specifically, two distinct artificial neural network (ANN) based composite models are developed in this paper. Each composite model is made up of three individual ANNs that are interconnected. Depending on the way in which the ANNs are interconnected, the total number of full-wave electromagnetic (EM) simulations and SPICE circuit simulations required for training the composite models are reduced. Overall, the composite models enable the use of analytic expressions instead of expensive and repeated full-wave EM and SPICE simulations to solve the repeater optimization problem within a Monte Carlo framework for efficient statistical analysis.
更多查看译文
关键词
Artificial neural networks (ANNs),copper-graphene interconnects,fin field effect transistors (FinFETs),parametric uncertainty,repeaters,statistical analysis
AI 理解论文
溯源树
样例
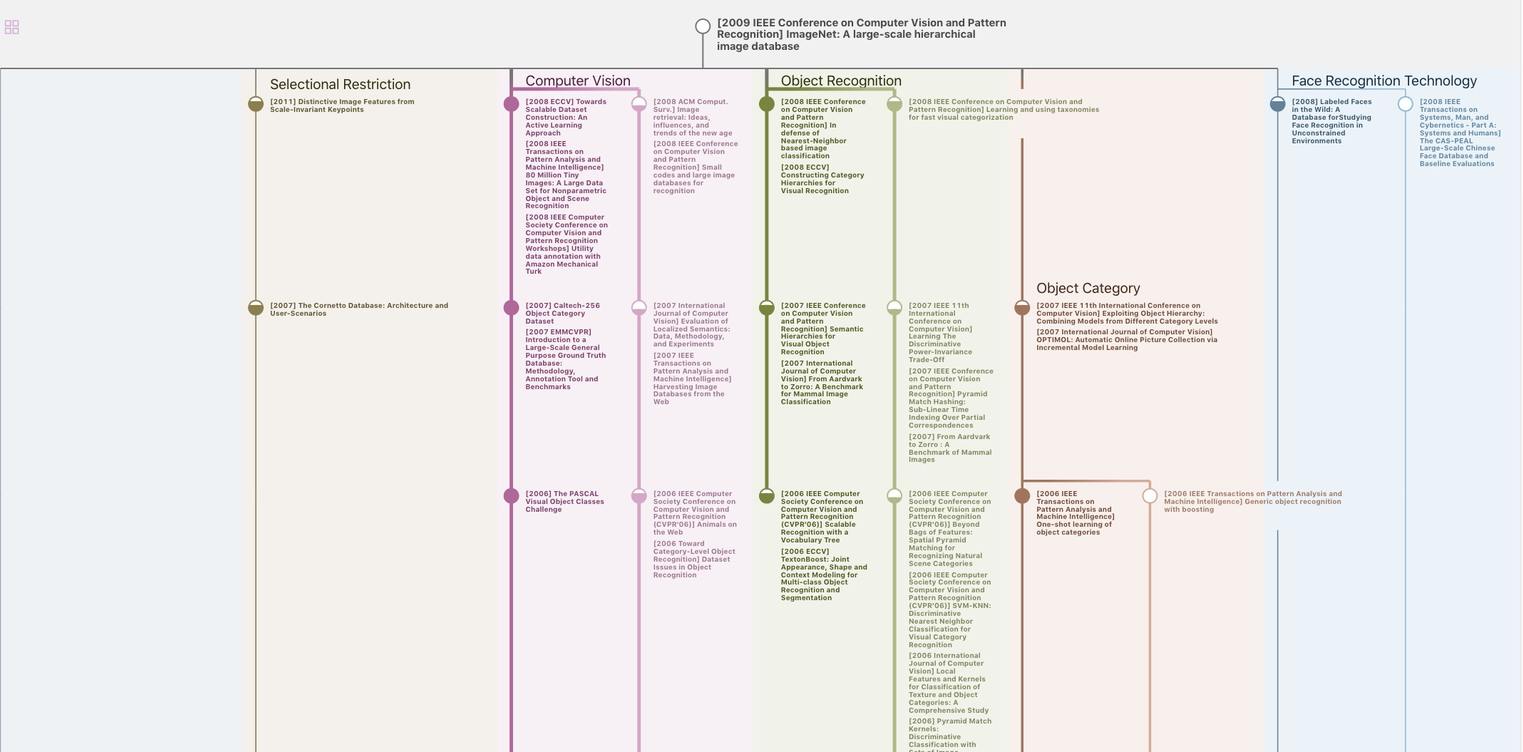
生成溯源树,研究论文发展脉络
Chat Paper
正在生成论文摘要