Enhancing IoT Intrusion Detection System Performance with the Diversity Measure As a Novel Drift Detection Method
2023 9th International Conference on Information Technology Trends (ITT)(2023)
摘要
The emergence of the Internet of Things (IoT) has revolutionized various sectors, such as healthcare, intelligent homes, agriculture, transportation, and manufacturing. Nevertheless, the rapid growth of IoT networks has introduced new security challenges, making them susceptible to a variety of attacks. In response, machine learning-driven intrusion detection approaches have been developed, which analyze IoT devices' behavior and communication patterns to detect and counteract suspicious activities. While these approaches exhibit high accuracy and low false alarm rates in static contexts, their performance stability in dynamic, evolving environments is yet to be determined. Model drift, the decline in a machine learning model's predictive capacity over time, is a crucial issue that can considerably affect machine learning-based intrusion detection systems if not identified and addressed promptly. Our work presents an innovative IoT Intrusion Detection System that incorporates the Diversity measure as a drift detection method to address model drift in IoT networks. The proposed concept can detect unknown attacks in IoT networks by employing a cutting-edge drift detection technique.
更多查看译文
关键词
Concept Drift,IoT,Intrusion Detection System,Data Drift,Machine Learning
AI 理解论文
溯源树
样例
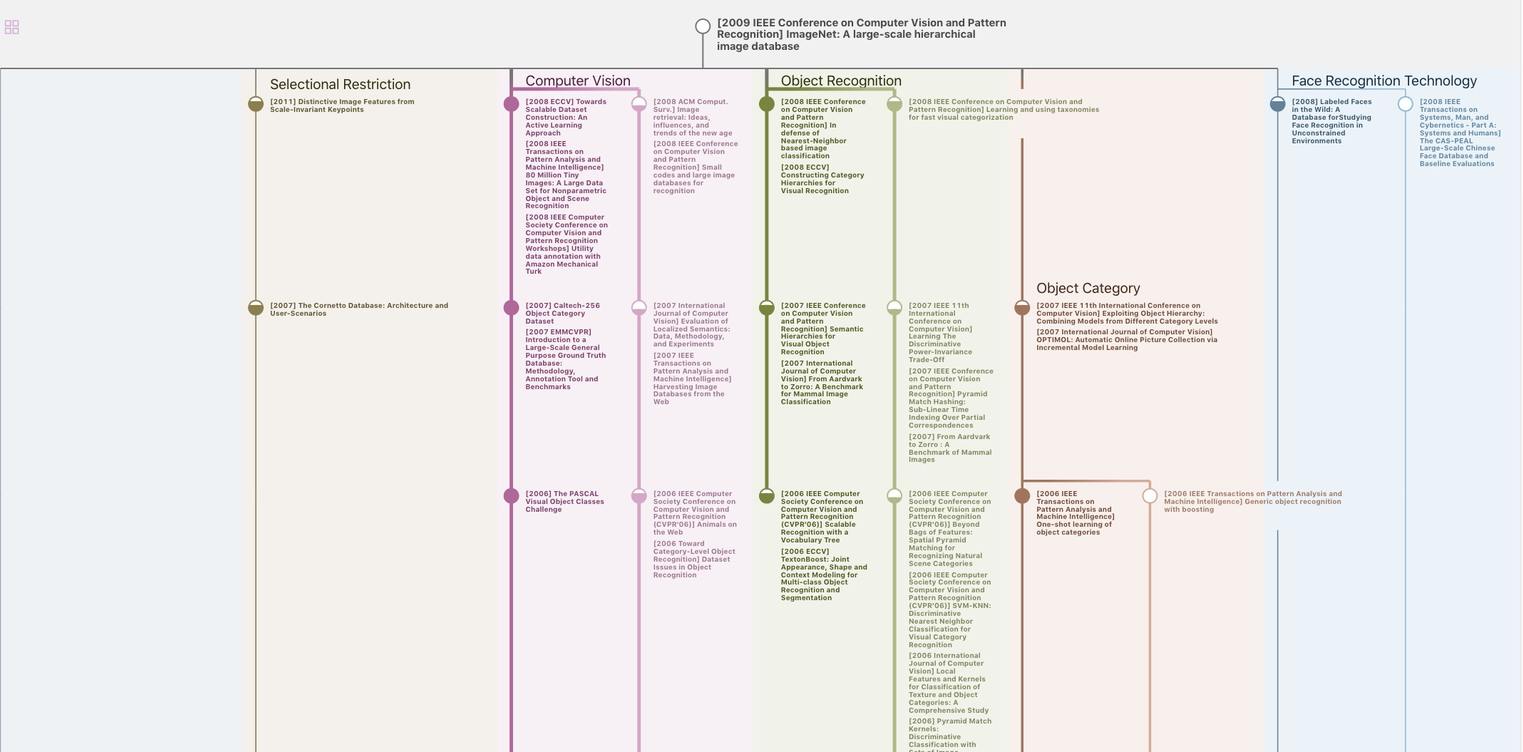
生成溯源树,研究论文发展脉络
Chat Paper
正在生成论文摘要