SimGCL: graph contrastive learning by finding homophily in heterophily
Knowledge and Information Systems(2024)
摘要
Graph Contrastive learning (GCL) has been widely studied in unsupervised graph representation learning. Most existing GCL methods focus on modeling the invariances of identical instances in different augmented views of a graph and using the Graph Neural Network (GNN) as the underlying encoder to generate node representations. GNNs generally learn node representations by aggregating information from their neighbors, where homophily and heterophily in the graph can strongly affect the performance of GNNs. Existing GCL methods neglect the effect of homophily/heterophily in graphs, resulting in sub-optimal learned representations of graphs with more complex patterns, especially in the case of high heterophily. We propose a novel Similarity-based Graph Contrastive Learning model (SimGCL), which generates augmented views with a higher homophily ratio at the topology level by adding or removing edges. We treat dimension-wise features as weak labels and introduce a new similarity metric based on feature and feature dimension-wise distribution patterns as a guide to improving homophily in an unsupervised manner. To preserve node diversity in augmented views, we retain feature dimensions with higher heterophily to amplify the differences between nodes in augmented views at the feature level. We also use the proposed similarity in the negative sampling process to eliminate possible false negative samples. We conduct extensive experiments comparing our model with ten baseline methods on seven benchmark datasets. Experimental results show that SimGCL significantly outperforms the state-of-the-art GCL methods on both homophilic and heterophilic graphs and brings more than 10% improvement on heterophilic graphs.
更多查看译文
关键词
Graph neural network,Heterophilic graph,Local assortativity,Contrastive learning
AI 理解论文
溯源树
样例
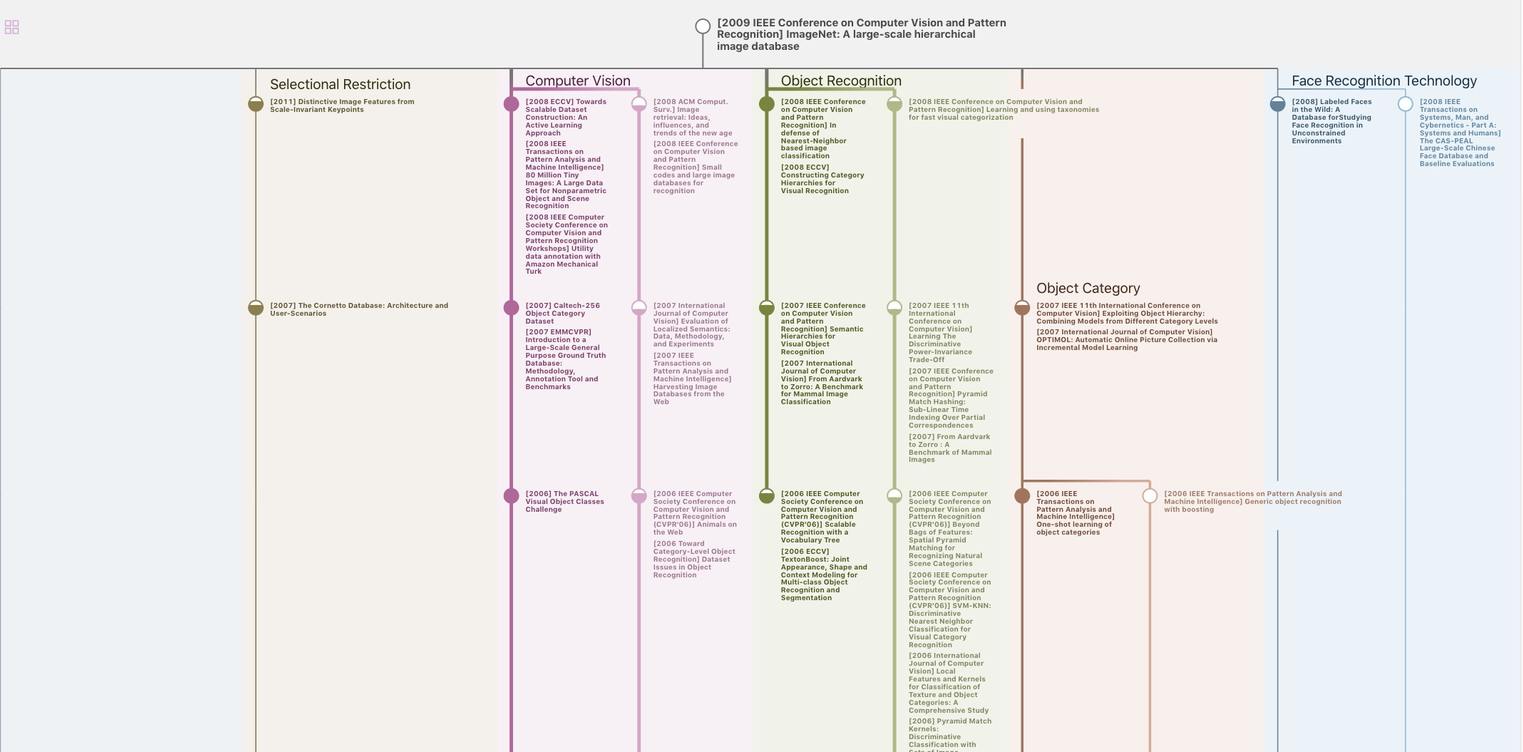
生成溯源树,研究论文发展脉络
Chat Paper
正在生成论文摘要