Efficient zeroth-order proximal stochastic method for nonconvex nonsmooth black-box problems
Machine Learning(2024)
摘要
Proximal gradient method has a major role in solving nonsmooth composite optimization problems. However, in some machine learning problems related to black-box optimization models, the proximal gradient method could not be leveraged as the derivation of explicit gradients are difficult or entirely infeasible. Several variants of zeroth-order (ZO) stochastic variance reduced such as ZO-SVRG and ZO-SPIDER algorithms have recently been studied for nonconvex optimization problems. However, almost all the existing ZO-type algorithms suffer from a slowdown and increase in function query complexities up to a small-degree polynomial of the problem size. In order to fill this void, we propose a new analysis for the stochastic gradient algorithm for optimizing nonconvex, nonsmooth finite-sum problems, called ZO-PSVRG+ and ZO-PSPIDER+. The main goal of this work is to present an analysis that brings the convergence analysis for ZO-PSVRG+ and ZO-PSPIDER+ into uniformity, recovering several existing convergence results for arbitrary minibatch sizes while improving the complexity of their ZO oracle and proximal oracle calls. We prove that the studied ZO algorithms under Polyak-Łojasiewicz condition in contrast to the existent ZO-type methods obtain a global linear convergence for a wide range of minibatch sizes when the iterate enters into a local PL region without restart and algorithmic modification. The current analysis in the literature is mainly limited to large minibatch sizes, rendering the existing methods unpractical for real-world problems due to limited computational capacity. In the empirical experiments for black-box models, we show that the new analysis provides superior performance and faster convergence to a solution of nonconvex nonsmooth problems compared to the existing ZO-type methods as they suffer from small-level stepsizes. As a byproduct, the proposed analysis is generic and can be exploited to the other variants of gradient-free variance reduction methods aiming to make them more efficient.
更多查看译文
关键词
Nonconvex optimization,Zeroth-order methods,Polyak-Łojasiewicz condition,Nonsmooth optimization,Query efficient methods,Adversarial attacks
AI 理解论文
溯源树
样例
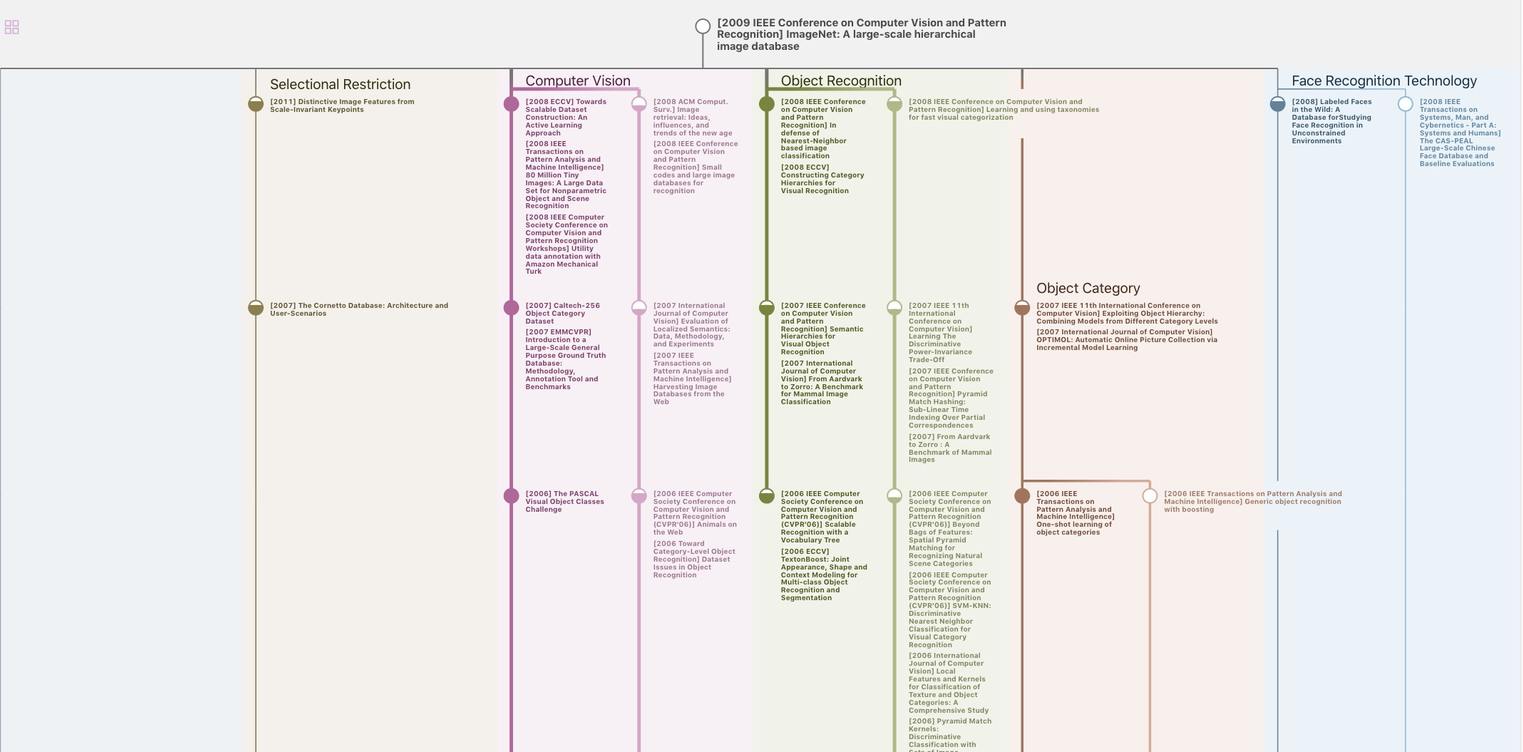
生成溯源树,研究论文发展脉络
Chat Paper
正在生成论文摘要