Gradient Boosting Approach to Predict Zero Carbon Achievement of Households in Kitakyushu
2023 9th International Conference on Control, Decision and Information Technologies (CoDIT)(2023)
摘要
The objective of this paper is to develop a gradient-boosting algorithm based machine-learning model that predicts the zero-carbon achievement of households using 11 months of energy data and questionnaire survey results, obtained from 198 smart houses located in Kitakyushu, Japan. In order to predict the zero-carbon achievement of households, we use LightGBM (light gradient boosting machine) classifier for feature selection and demonstrate that the most important features are the mean absolute error (MAE) of housing type, floor heating, average monthly electricity bill, and average monthly gas bill. This light GBM-based model can also predict the zero carbon achievement of households that did not participate in the questionnaire survey. This model helps us determine households with low carbon emissions and factors that influence zero carbon achievement. The work is currently ongoing, and the results presented in this paper are encouraging and provide valuable insights for developing effective household energy policies, which is a big step towards achieving nearly zero-carbon houses.
更多查看译文
关键词
average monthly electricity bill,average monthly gas bill,effective household energy policies,gradient-boosting algorithm,Japan,Kitakyushu,light GBM-based model,light gradient,low carbon emissions,machine-learning model,MAE,mean absolute error,time 11.0 month,zero carbon achievement prediction,zero-carbon houses
AI 理解论文
溯源树
样例
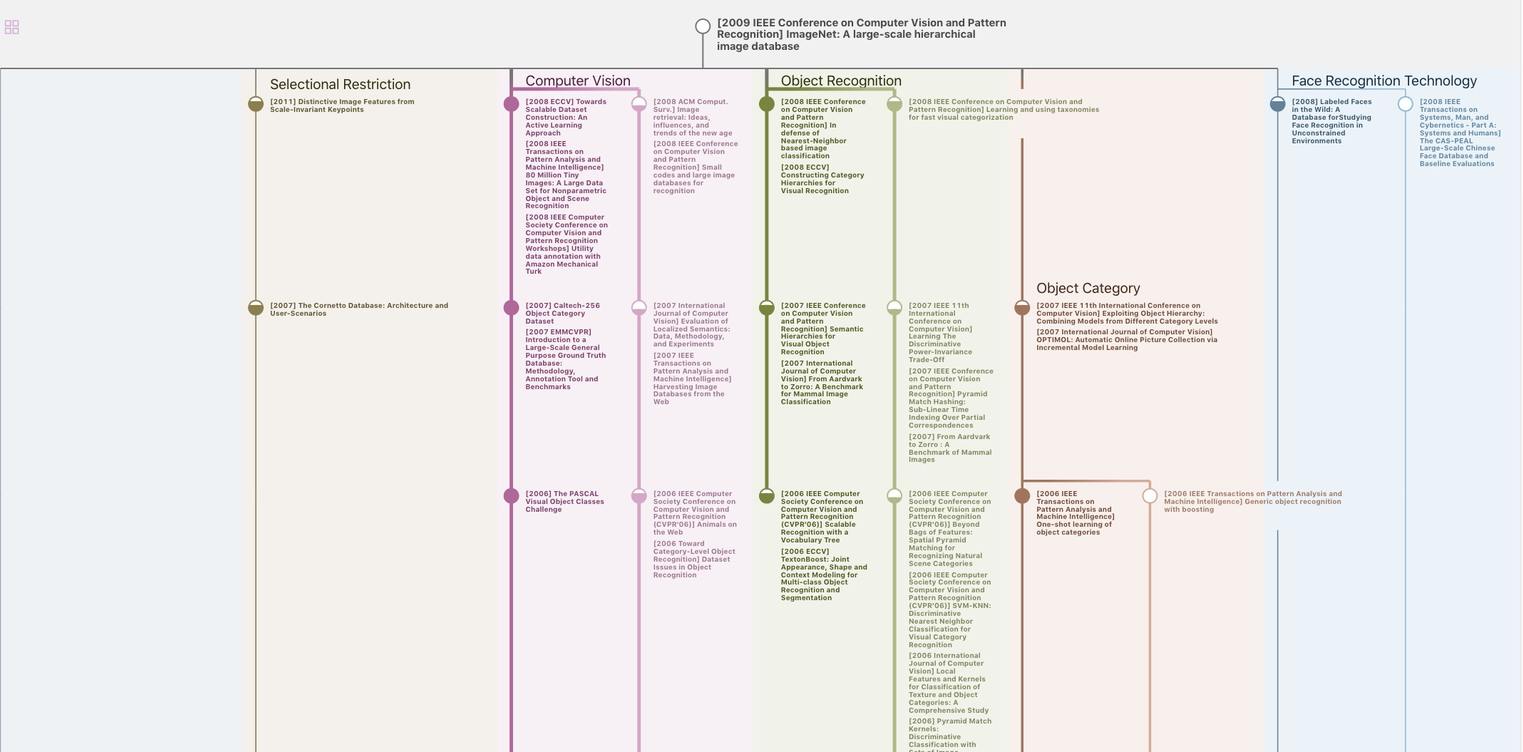
生成溯源树,研究论文发展脉络
Chat Paper
正在生成论文摘要