Deep Learning Architecture with an Optimized Convolutional Processing for the Segmentation of Retinal Blood Vessels
2023 9th International Conference on Control, Decision and Information Technologies (CoDIT)(2023)
摘要
The retinal vascular tree (RVT) is crucial for the diagnosis of various ophthalmological diseases. Efficient segmentation of the RVT with reduced runtime is essential for clinical purposes. Recently, convolutional neural networks (CNNs) have been used for RVT segmentation. However, these architectures typically apply fixed and standard size of convolution kernels for all blocks, which may be unsuitable for accurately capturing vessel scales. In addition, these kernels are applied using 3D convolution layers across all channel depths, leading to higher computational complexity. In this work, we propose a novel deep learning architecture. The main contribution consists of performing a convolution processing where kernel size is chosen with respect to vessel scale variation, in order to enhance the quality of the segmentation of vascular trees. In addition, the convolution processing is insured through several layers with 2D kernels, to reduce the computational complexity. The proposed architecture is evaluated on DRIVE database reaching an average accuracy and sensitivity respectively in the order of 97.69
%
and 91.69% in 0.75 second per fundus image.
更多查看译文
关键词
3D convolution layers,convolution kernels,convolution processing,convolutional neural networks,convolutional processing,deep learning architecture,kernel size,ophthalmological diseases,retinal blood vessels,retinal vascular tree,RVT segmentation,vascular trees,vessel scale variation,vessel scales
AI 理解论文
溯源树
样例
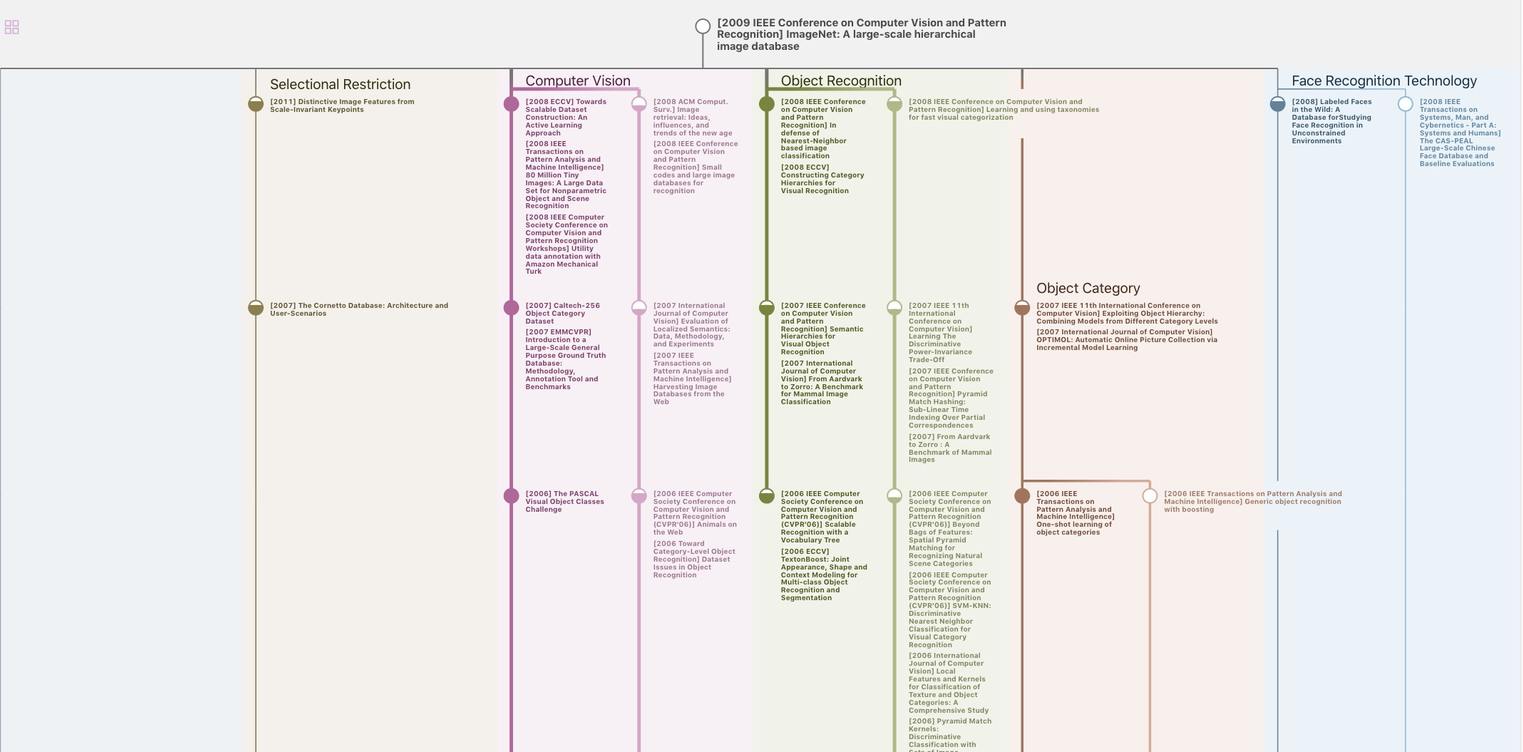
生成溯源树,研究论文发展脉络
Chat Paper
正在生成论文摘要