Neural Network Architectures Evaluation on the Prediction Accuracy of the Ionospheric Total Electron Content
2023 Radiation and Scattering of Electromagnetic Waves (RSEMW)(2023)
摘要
The surge in publications on a machine learning technique and the widespread use of neural networks methods for predicting ionospheric parameters, in particular, the total electron content, due to its huge role in the functioning of various technological systems, indicates the prospects for further development of forecasting methods. This sets the task of choosing the most promising neural network architectures, which is the goal of this work. Attention was paid not only to the statistical characteristics provided by various architectures, but also to the complexity of the implementation of algorithms and computational costs. As a basis for the implementation of architectures, LSTM and GRU are chosen as the most widely used, whose effectiveness has long been confirmed. The paper proposes and considers architectures of multilayer neural networks belonging to the feedforward, bidirectional and convolutional classes. A modification of the TCN architecture is considered in relation to forecasting problems, as well as a comparison with fully connected architectures that act as a baseline. Since there is a relationship between TEC with solar and geomagnetic indices, solar wind parameters, a set of indices were used to increase the accuracy of the forecast. Since terrestrial and satellite systems using TEC are distributed on a global scale, the dependence on the position of GPS receivers is investigated. Statistical characteristics (MAE, MAPE, RMSE) are given for various combinations of architectures for 10 Russian stations. The bidirectional architectures had the highest forecasting accuracy, while the TCN had the highest performance and comparable accuracy in selected cases.
更多查看译文
关键词
deep learning,Recurrent Neural Networks,Bidirectional,BiLSTM,BiGRU,Temporal Convolutional Network,total electron content,Global Positioning System,forecasting
AI 理解论文
溯源树
样例
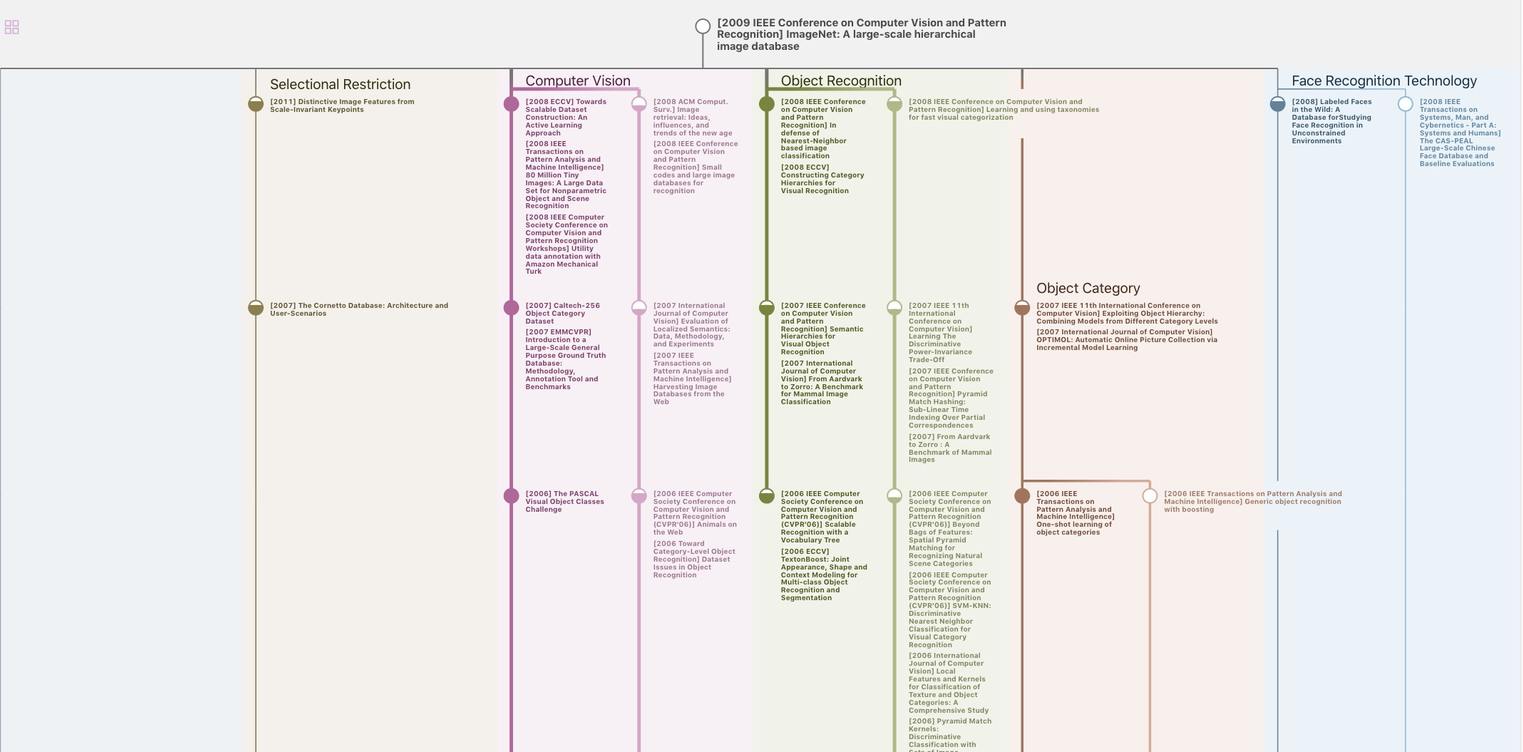
生成溯源树,研究论文发展脉络
Chat Paper
正在生成论文摘要