Improving Semantic Segmentation Performance of Deep Networks for Autonomous Driving through Loss Functions
2023 IEEE Guwahati Subsection Conference (GCON)(2023)
摘要
Semantic segmentation of images for autonomous driving is a dense pixel-level prediction task. Present-day algorithms make extensive use of deep learning models for parsing the image. A large number of classes and class imbalance in the autonomous driving dataset make accurate labeling of pixels challenging. Cross- Entropy(Ce)loss function is mainly used to optimize the network parameters during training. The overall performance gain resulting from CE loss comes from large object classes. In contrast, the segmentation accuracy of smaller classes and object boundaries remains inferior, even with increased overall accuracy. To address this limitation, task- specific custom loss functions have been developed and used for various applications like biomedical image processing. However, they have rarely been used for road scene segmentation in an autonomous driving context. To fill this gap, we develop a composite loss function by linearly combining the CE loss and boundary/region-based loss to improve the existing state- of-the-art model. We give empirical evidence that our custom loss function improves the segmentation scores on smaller object categories and object boundaries. Through extensive experiments on the Cityscapes dataset, we show that Deep networks trained with our loss function see a gain of more than 2 % on the overall
$m$
IoU score. In some smaller classes, these scores are increased by more than 20%.
更多查看译文
关键词
Computer vision,Autonomous driving,Semantic segmentation,Deep learning,Loss function
AI 理解论文
溯源树
样例
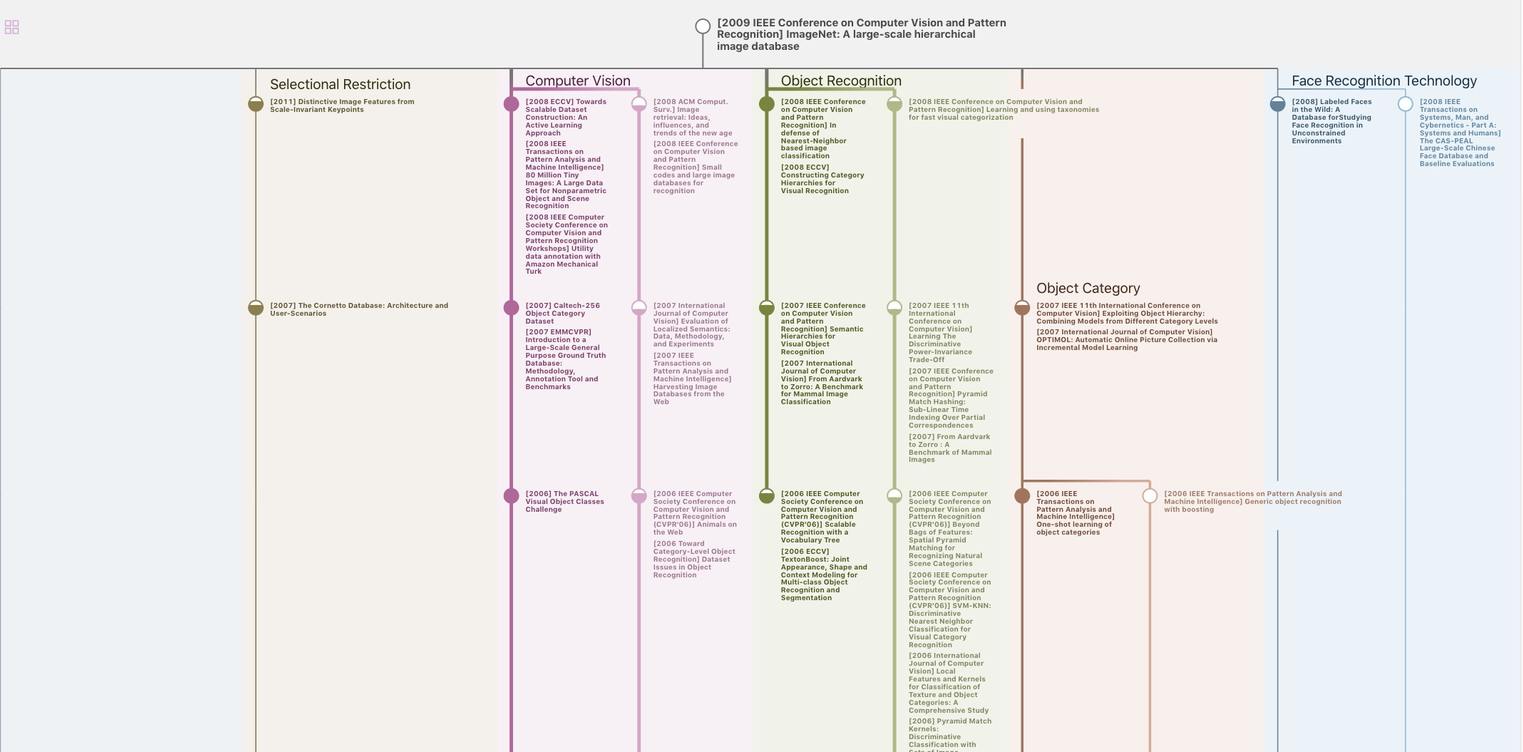
生成溯源树,研究论文发展脉络
Chat Paper
正在生成论文摘要