Independent Vector Analysis with Sparse Inverse Covariance Estimation: An Application to Misinformation Detection
2023 IEEE 33rd International Workshop on Machine Learning for Signal Processing (MLSP)(2023)
摘要
The analysis of multiple sets of data, such as multi-subject or multimodal data, is crucial for many computer science and engineering problems. The primary objective in such problems is to extract relevant features for machine learning tasks, commonly classification or detection tasks. Independent vector analysis (IVA) has emerged as a powerful technique for multi-modal learning and feature extraction due to its minimal model assumptions, i.e., statistical independence and ability to effectively maximize interactions within and across datasets. Despite the appeal of minimizing model assumptions, prior knowledge, such as sparse associations of features, is usually available. Therefore, incorporating sparsity constraints into IVA can mitigate the effects of confounding features promising to significantly improve the utility of the final extracted features. This paper discusses integrating sparsity constraints into the IVA model through the inverse covariance matrix and introduces a new algorithm, IVA with sparse inverse covariance estimation (IVA-SPICE). We present the parallel implementation of IVA-SPICE and demonstrate its efficacy through simulations involving diverse parameters. Finally, we demonstrate the usefulness of IVA-SPICE in multi-modal misinformation detection, highlighting its practical capabilities.
更多查看译文
关键词
Independent Vector Analysis,Inverse Covariance Estimation,Graphical Lasso,Multi-modal Misinformation Detection
AI 理解论文
溯源树
样例
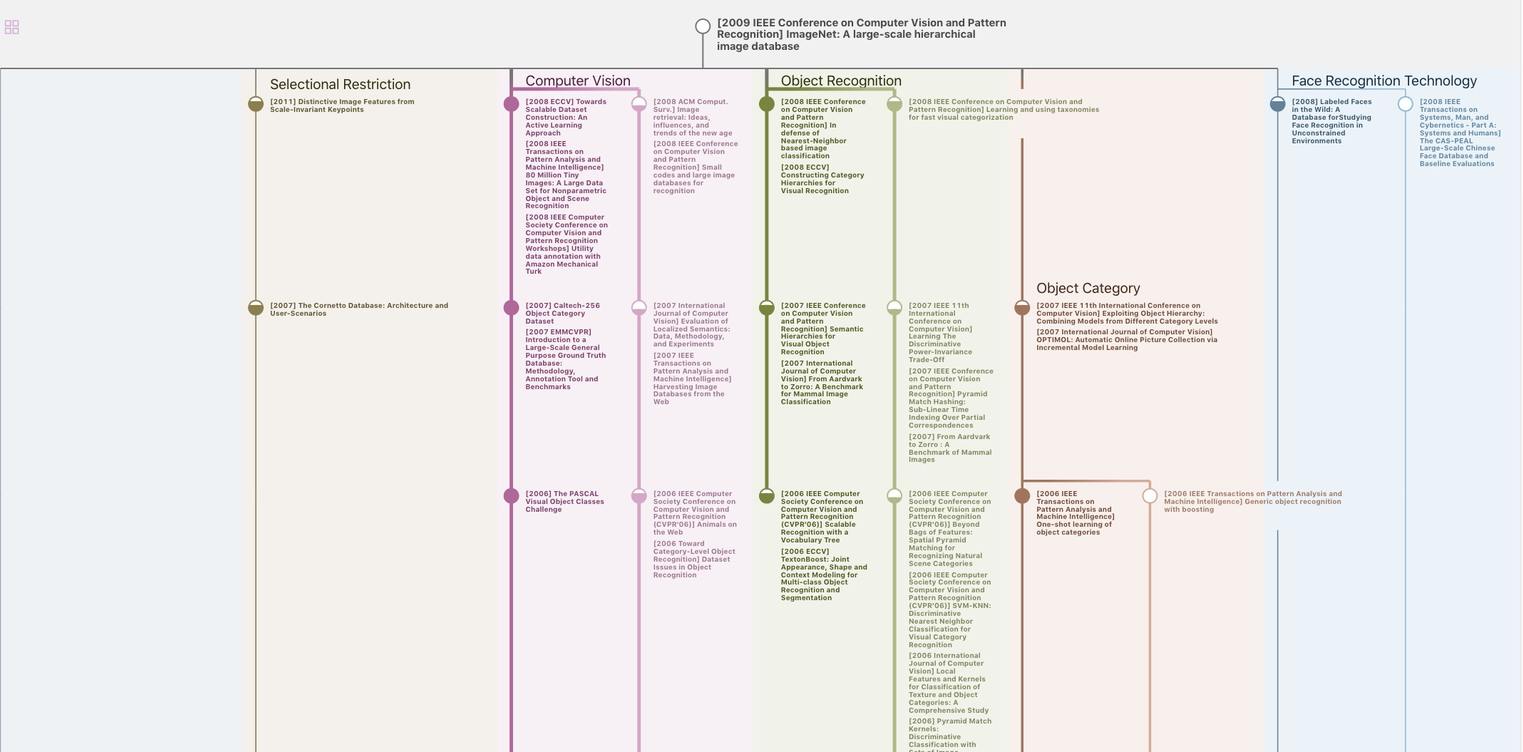
生成溯源树,研究论文发展脉络
Chat Paper
正在生成论文摘要