Locally Differential Private Federated Learning with Controllable Perturbation Domains
2023 International Conference on Networking and Network Applications (NaNA)(2023)
摘要
As a kind of distributed machine learning, federated learning allows each party to upload local model parameters instead of sensitive data to train a more accurate global model collaboratively. However, since it turns out that the original training data can be inferred by intercepting and analyzing the passed model parameters, local differential privacy is introduced into the federated learning to provide robust privacy guarantees and high efficiency. At present, most existing approaches prefer to adjust the perturbation domain range of the model parameters only according to the privacy budget, which is difficult to ensure the accuracy of the model under the condition of limited privacy budget or fewer participating clients, resulting in low usability and practicability of the global model. To tackle this, we propose a locally differential private federated learning with controllable perturbation domains. First, we design a local differential privacy mechanism, which generates three perturbation domains centered on the true value. The range and perturbation probability of each perturbation domain are adjusted by controlling two factors, the privacy budget and the unit size of the perturbation domain. Specially, the true value is mapped to the center perturbation domain with a large probability and to the two-side perturbation domains with a small probability. Second, to ensure the training process of federated learning is protected from inference attacks, we apply the designed mechanism to the transmission of parameters in federated learning. Finally, we analyze and prove the privacy and utility of the mechanism in detail, and conduct comparative experiments in terms of accuracy on three standard datasets. Theoretical and experimental results show that the proposed approach outperforms other state-of-the-art approaches.
更多查看译文
关键词
privacy-preserving,federated learning,local differential privacy,privacy and utility trade-off
AI 理解论文
溯源树
样例
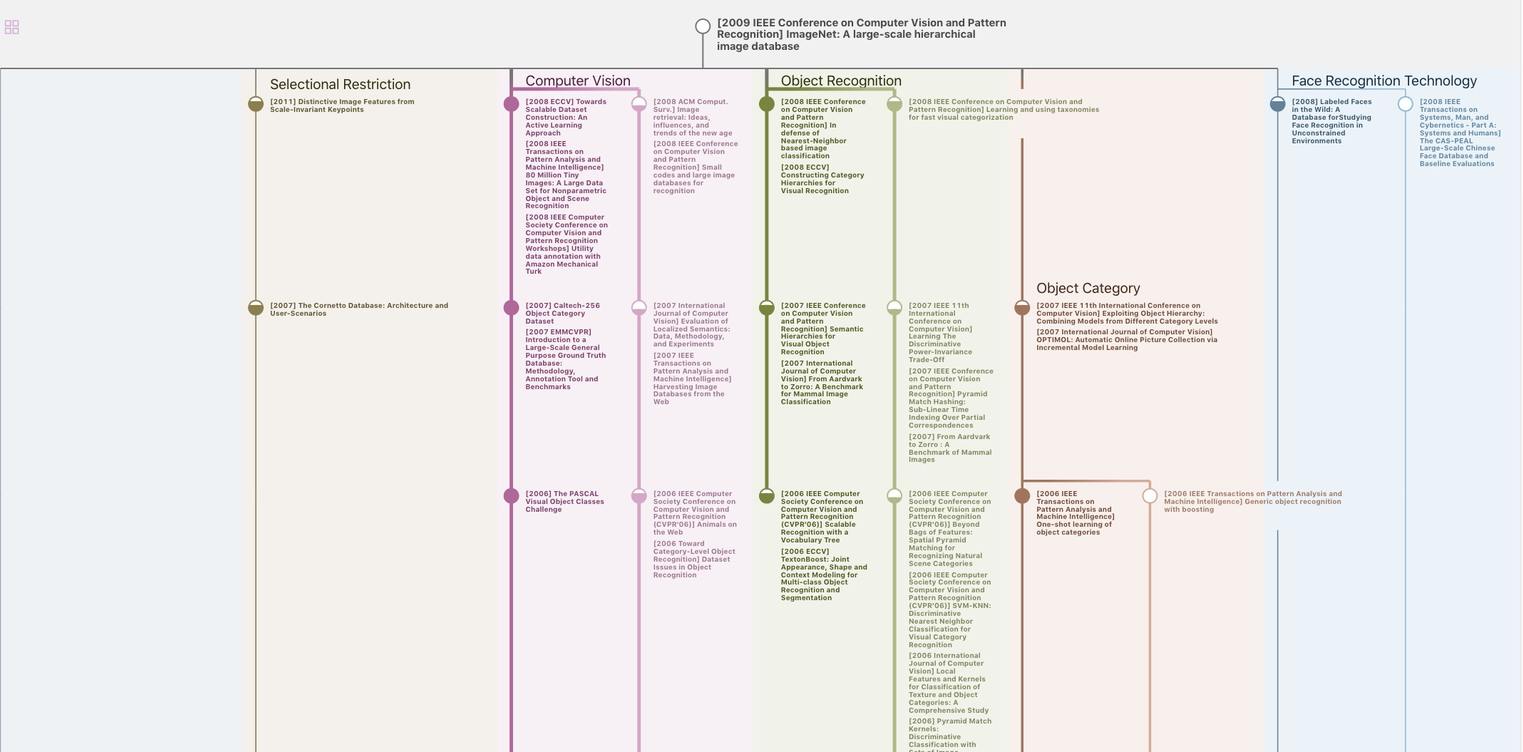
生成溯源树,研究论文发展脉络
Chat Paper
正在生成论文摘要