Potato Leaf Disease Identification using Hybrid Deep Learning Model
2023 International Conference on Network, Multimedia and Information Technology (NMITCON)(2023)
摘要
The potato is one of the most significant crops in the world. However, it is prone to several leaf diseases that can result in significant productivity losses, leading to economic challenges. Early and precise disease identification is essential for sensitive crops like potatoes. Deep learning approaches have demonstrated excellent potential in image-based disease classification tasks in recent years. This paper presents a hybrid strategy for classifying potato leaf image diseases by integrating Optimised Convolutional Neural Network (OCNN) and Long Short-Term Memory (LSTM) networks. The Adaptive Shark Smell Optimisation (ASSO) technique is used to optimize the weights of CNN models. The CNN component is initially used to extract pertinent characteristics from Potato leaves, capturing significant visual patterns related to various diseases. These extracted features are then fed into the LSTM model, which utilizes its sequential learning capability to model the temporal dependencies among the extracted features. The model performance is analyzed based on Accuracy, Precision, Recall, and F1-score criteria. Experimental results showed that the hybrid OCNN-LSTM model outperforms the individual CNN, LSTM, and MobileNet models. The proposed model results are compared with existing state-of-the-art work, and it was found that the OCNN-LSTM model performed better and received 99.02% accuracy.
更多查看译文
关键词
Potato,leaf disease,OCNN-LSTM,CNN,LSTM
AI 理解论文
溯源树
样例
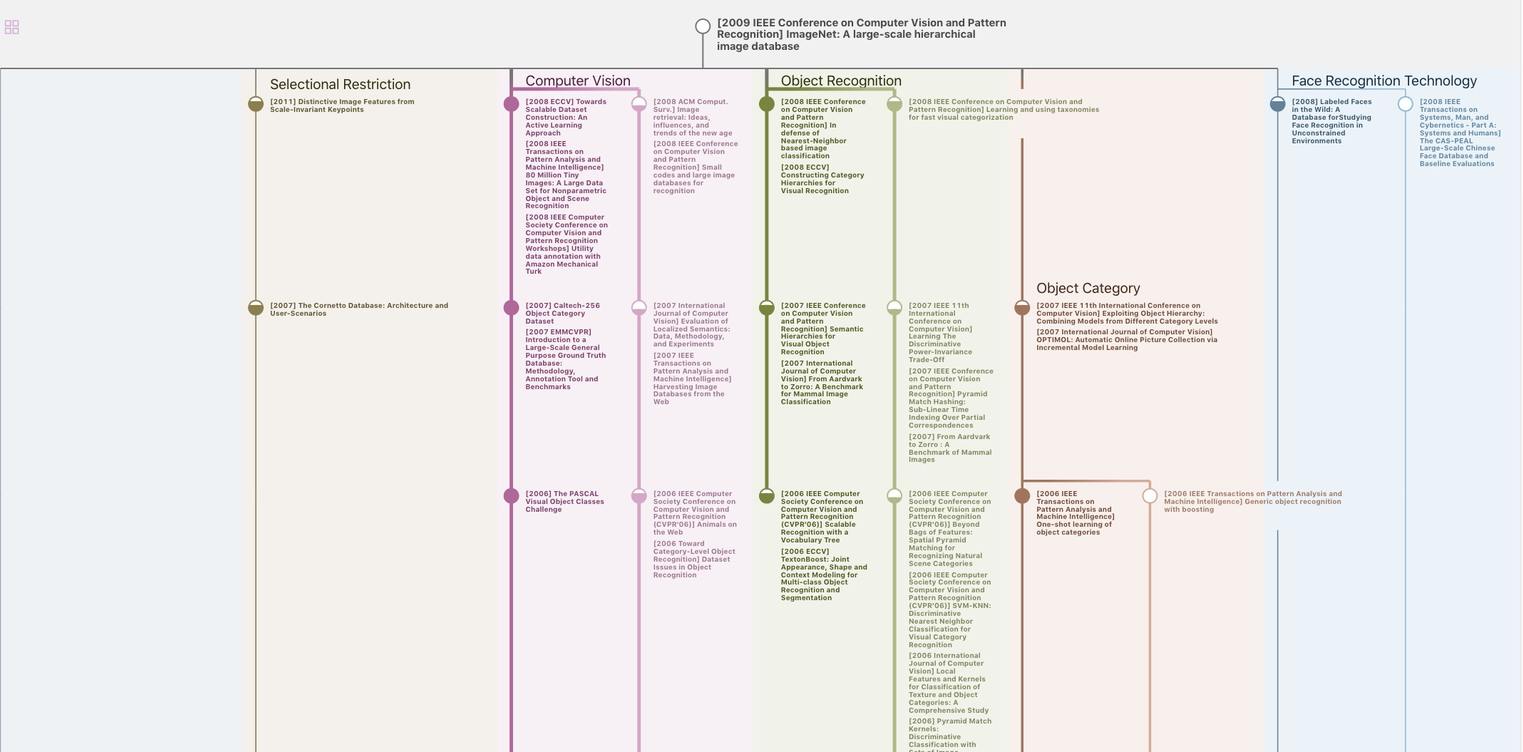
生成溯源树,研究论文发展脉络
Chat Paper
正在生成论文摘要