A modified U-Net with dilated convolution and CBAM
2023 2nd International Conference on Robotics, Artificial Intelligence and Intelligent Control (RAIIC)(2023)
摘要
Interstitial pneumonia is a lung disease with a high incidence rate and high mortality. Its Medical imaging is complex and diverse, and the diagnostic effectiveness depends on the clinician's ability to read CT. Traditional methods are difficult to diagnose interstitial pneumonia, and there is misdiagnosis or missed diagnosis. Therefore, timely and accurate diagnosis of interstitial pneumonia is of great significance to modern medicine. This paper proposes an improved image segmentation algorithm based on U-Net to improve the accuracy of lesion segmentation in interstitial pneumonia. First, replacing standard convolution with dilated convolution can expand the receptive field without introducing additional parameters, extract higher dimensional semantic features and enlarge the model's perception of global information; Then, combined with the Convolutional Block Attention Module can infer the attention coefficient moment matrix along the two dimensions of channel and space, adaptively modify the input feature map and pay more attention to the visualization features of interstitial pneumonia to improve segmentation performance. This paper conducted extensive experiments on both the interstitial dataset and the COVID-19 public dataset. Compared with mainstream image segmentation methods, the proposed method has improved segmentation PA, Recall, IoU, and F1 in interstitial pneumonia, reaching 84.97%, 86.86%, 87.25%, and 85.90%, respectively. The method proposed in this paper can effectively achieve lesion area segmentation in interstitial pneumonia.
更多查看译文
关键词
Interstitial Pneumonia,Image Segmentation,U-Net,Dilated Convolution,Convolutional Block Attention Module
AI 理解论文
溯源树
样例
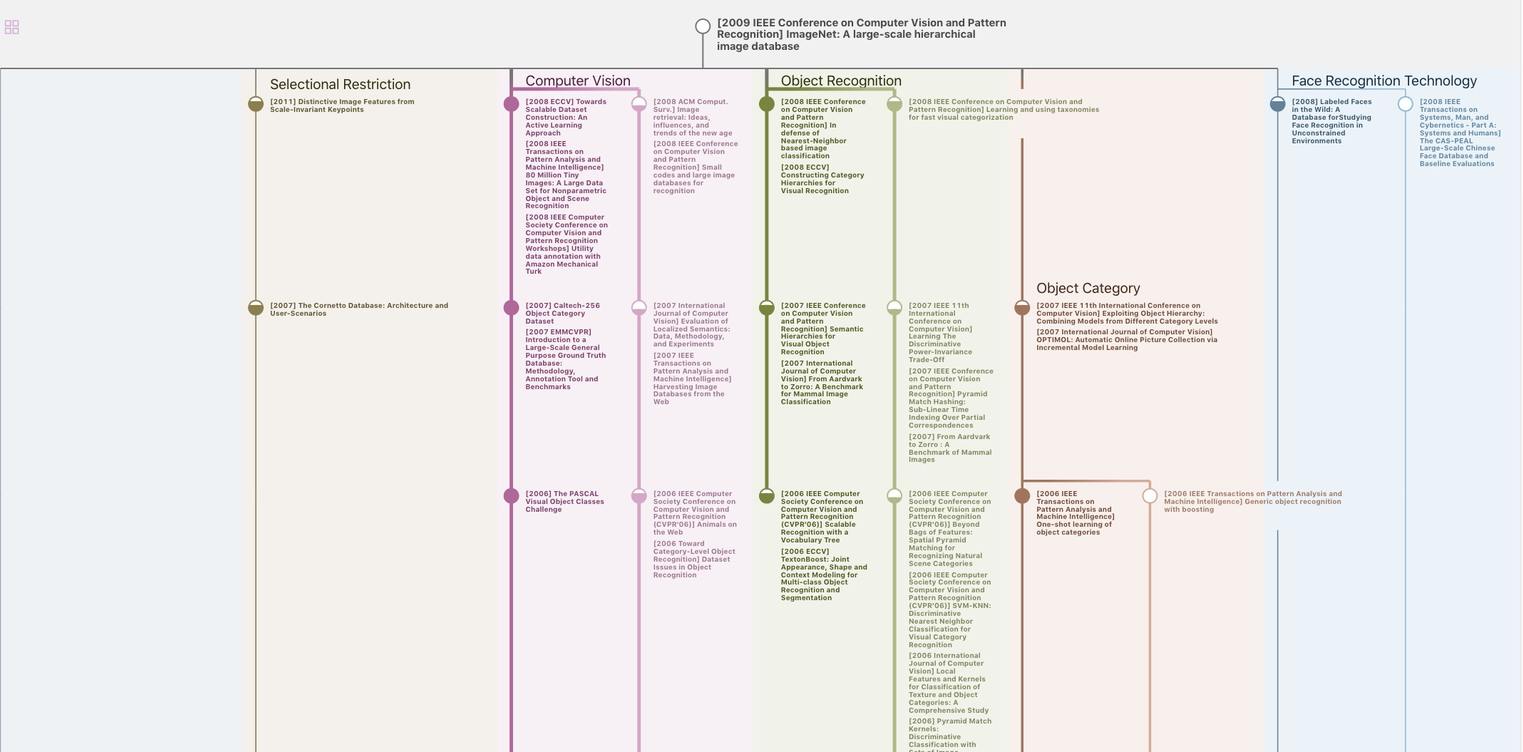
生成溯源树,研究论文发展脉络
Chat Paper
正在生成论文摘要