Utilizing Latent Dirichlet Allocation for Analyzing Topics in Undergraduate Theses
2023 10th International Conference on Information Technology, Computer, and Electrical Engineering (ICITACEE)(2023)
摘要
Topic modeling is an important and interesting research area that can assist in discovering patterns and underlying themes in large datasets. This research aims to identify commonly used topics in previous undergraduate thesis research through text mining using Latent Dirichlet Allocation (LDA) as the topic modeling method. The study utilizes abstracts as the primary source to identify the content of the research documents accurately and quickly. We collected a dataset of 666 abstract theses and utilized LDA to identify the dominant themes and topics within the corpus. Our results indicate that the LDA model achieved a coherence score of up to 0.448, indicating a reasonable level of coherence in the identified topics. We identified six main topics within the dataset, including system analysis and design, data mining, computer networks, decision support systems, software testing, and computer security. The result of this study is expected to serve as a useful reference for students and thesis supervisors in selecting future thesis topics and identifying novel and underexplored topics.
更多查看译文
关键词
latent dirichlet allocation,LDA,topic modeling,topic discovery,topic exploration,topic analysis
AI 理解论文
溯源树
样例
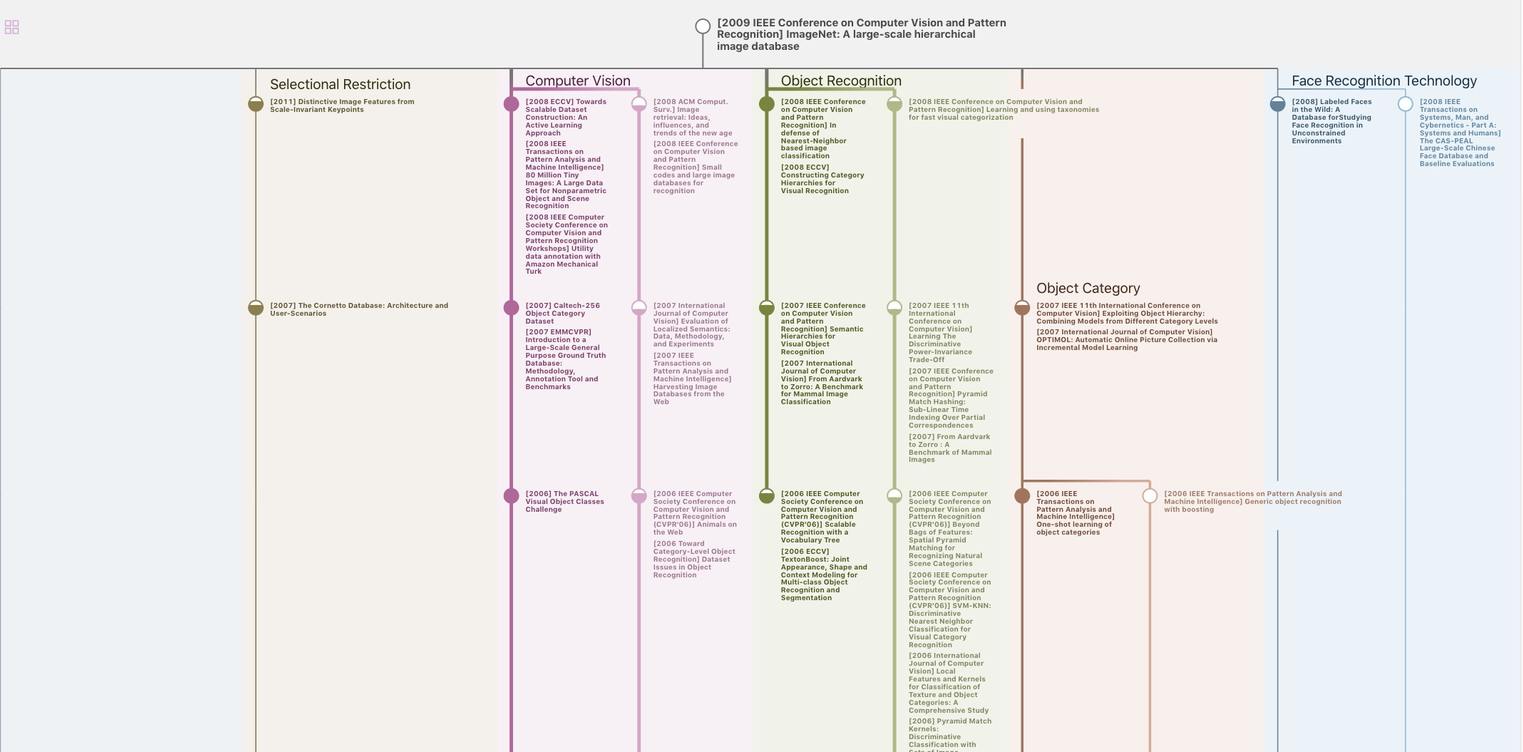
生成溯源树,研究论文发展脉络
Chat Paper
正在生成论文摘要