Hyper-spectral image classification using adiabatic quantum computation
IGARSS 2023 - 2023 IEEE INTERNATIONAL GEOSCIENCE AND REMOTE SENSING SYMPOSIUM(2023)
摘要
Supervised machine learning techniques are widely used for hyper-spectral images segmentation. A typical simple scheme of classification of such images probabilistically assigns a label to each individual pixel omitting information about pixel surroundings. In order to achieve better classification results for real world images one has to agree the local label obtained from the classifier with the classes of pixel neighborhood. A popular way to do it is through a probabilistic graphical model, where label distributions for individual pixels are mapped into a graph of neighborhood relations. One way to realize this approach is to use Ising models, where class probability is mapped to spin energy and class-class interaction is mapped to the spins coupling. By finding low energy states of such an Ising model we can perform post-processing of segmented images. In this work we present how this post-processing can be implemented using a quantum annealer.
更多查看译文
关键词
hyper-spectral image segmentation,energy-based models,quantum annealing
AI 理解论文
溯源树
样例
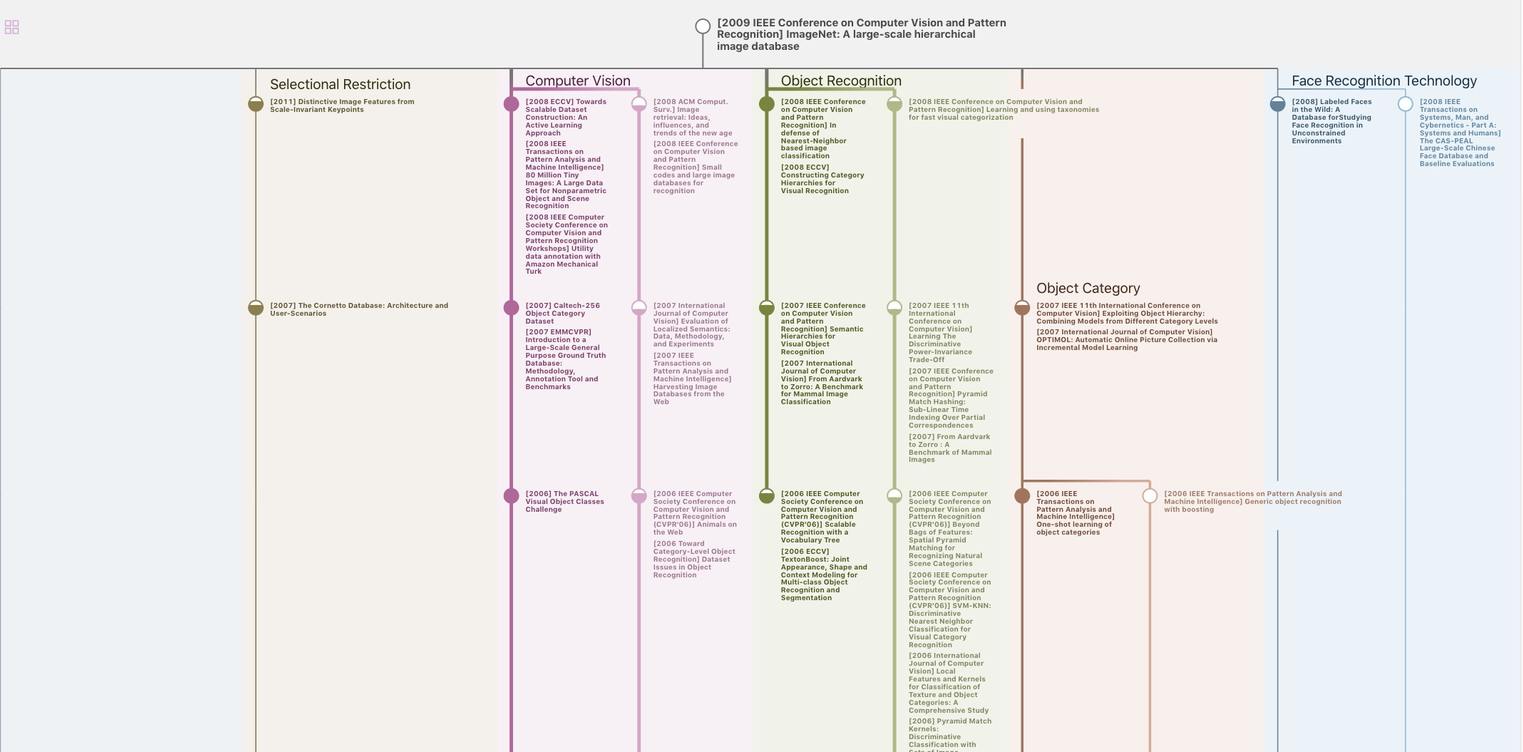
生成溯源树,研究论文发展脉络
Chat Paper
正在生成论文摘要