Impact of permuted spectral neighborhood of high-dimensional msts rs data on crop classification performance with dnn models
IGARSS 2023 - 2023 IEEE INTERNATIONAL GEOSCIENCE AND REMOTE SENSING SYMPOSIUM(2023)
摘要
It is still a challenge for existing DNN based models to synergistically exploit the spatial, temporal, and especially spectral information of a crop present in multi-sensor time series (MSTS) remote sensing (RS) images and provide accurate crop classification while keeping the generalization ability of DNN models high. This imbalance requires investigation and demands novel CNN and RNN model-based approaches that can address the issue. The novel models proposed in this study involve the concepts of permuted localized spectral convolutions, localized spatial convolutions, and bi-directional recurrent units. The permuted spectral band stacking strategy is explored in this study to strengthen the influence of the spectral information. Overall, 6 models are proposed namely; Perm-1D-CNN, Perm-3D-CNN, Perm-RNN, Perm-1D-CRNN, Perm-2D-CRNN, and Perm-3D-CRNN. The qualitative and quantitative assessments reflect the higher generalization ability of the Perm-3D-CRNN along with its high classification accuracy. Also, the impact of spectral band permutations and localized spectral convolutions on the performance of DNN models is significant toward improved generalization.
更多查看译文
关键词
localized spectral information,CNNs,multi-sensor,crop classification,time-series
AI 理解论文
溯源树
样例
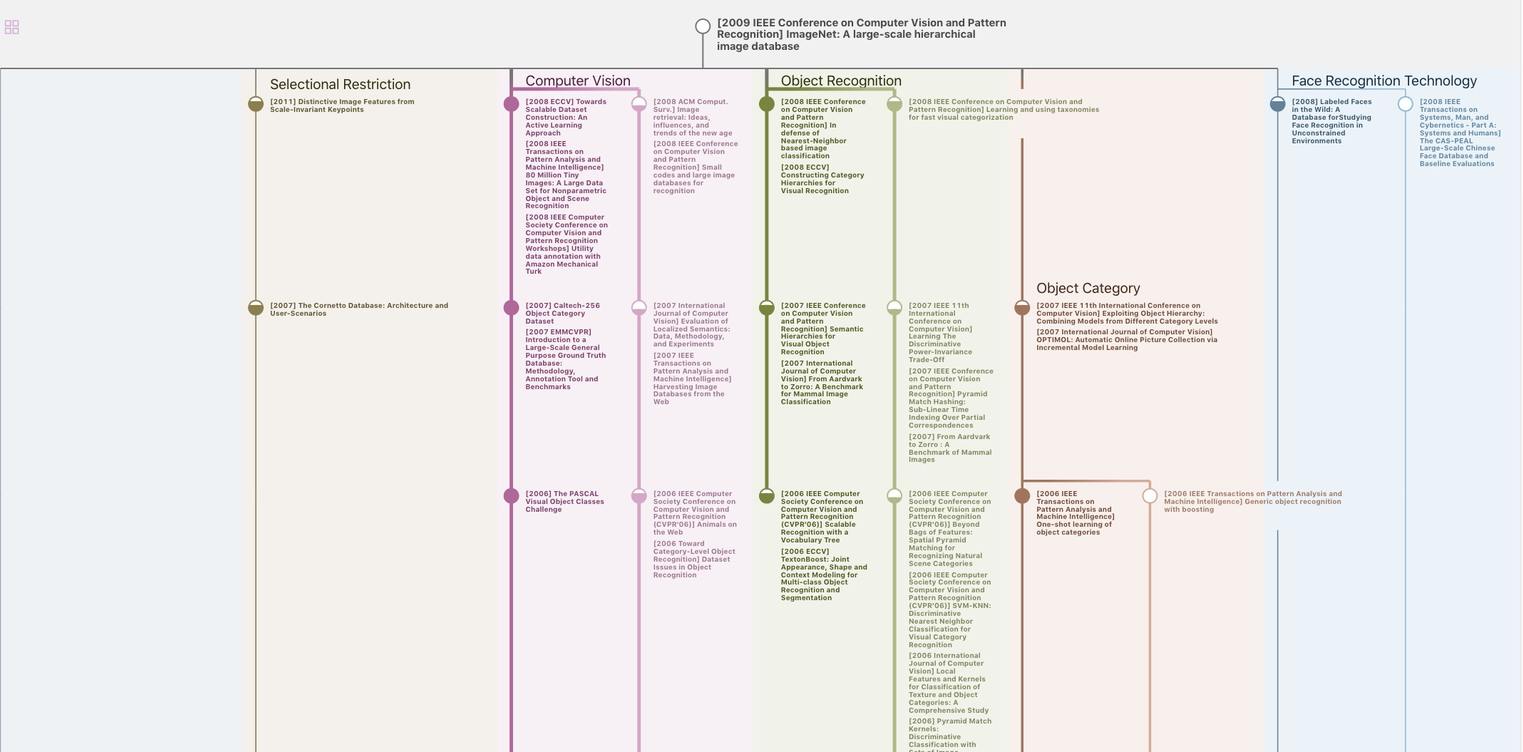
生成溯源树,研究论文发展脉络
Chat Paper
正在生成论文摘要