Estimation of Probability Density of Potential Fire Intensity Using Quantile Regression and Bi-Directional Long Short-Term Memory.
IGARSS 2023 - 2023 IEEE INTERNATIONAL GEOSCIENCE AND REMOTE SENSING SYMPOSIUM(2023)
摘要
Accurate estimation of potential fire intensity (PFI) can improve wildfire management. The PFI can be simulated by fire spread models, but with immeasurable uncertainties. There are also some difficulties in estimating PFI with multi-source drivers, since the fire spread is limited by fire suppression. This study aimed to estimate the probability density of PFI over southwestern China, using time-series fuel and weather data as well as topographic data. The Quantile Regression and Bi-directional Long Short-Term Memory were selected to establish the prediction model of PFI. The results showed that the QR-BiLSTM performed best at the 90% confidence level. The modal PFI values extracted from the estimated probability density were closer to the observed values. This study suggests the potential of probability density estimation of PFI with artificial intelligence, for which improves wildfire risk assessment.
更多查看译文
关键词
Wildfire,potential fire intensity,probability density,quantile regression,Bi-directional Long Short-Term Memory
AI 理解论文
溯源树
样例
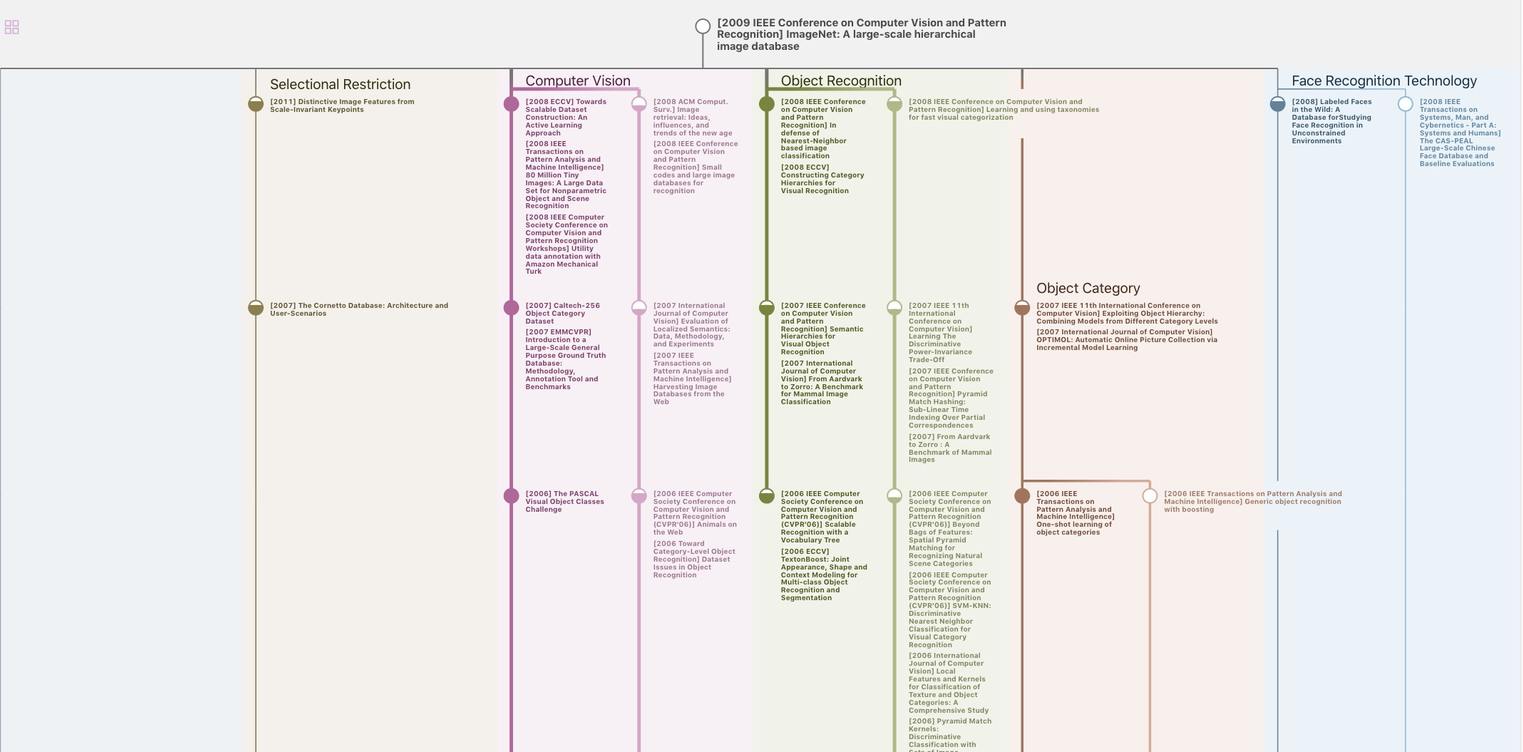
生成溯源树,研究论文发展脉络
Chat Paper
正在生成论文摘要