Graph Neural Network Based Interpretable Spectral Unmixing for Hyperspectral Unmixing Hyperspectral IIRS Data Onboard Chandrayaan-2 Mission
IGARSS 2023 - 2023 IEEE INTERNATIONAL GEOSCIENCE AND REMOTE SENSING SYMPOSIUM(2023)
摘要
Although hyperspectral sensors are highly effective in mapping the minerals, the intimate nonlinear mixing and resolution tradeoff affect their effectiveness. In this regard, this study proposes a graph-based spectral unmixing strategy. The proposed approach leverages the advantages of both graph-based and deep learning based approaches. Additionally, the current study is a pioneer approach of using the graph-based approach for spectral unmixing. The spectral and spatial latent manifolds of the input patches are learned, and this information along with the endmember prior is used to formulate a graph-based representation. Further graph convolution approach is used to soft classify the spectra yielding fractional abundances. The results of the proposed approach on standard, synthetic and real-world data indicates that the proposed approach performs better than the state-of-the-art unmixing approaches. Moreover, the graph-based representations make the approach interpretable and facilitate the consideration of the spatial autocorrelation.
更多查看译文
关键词
Graph Neural Network,Spectral Unmixing,Interpretability
AI 理解论文
溯源树
样例
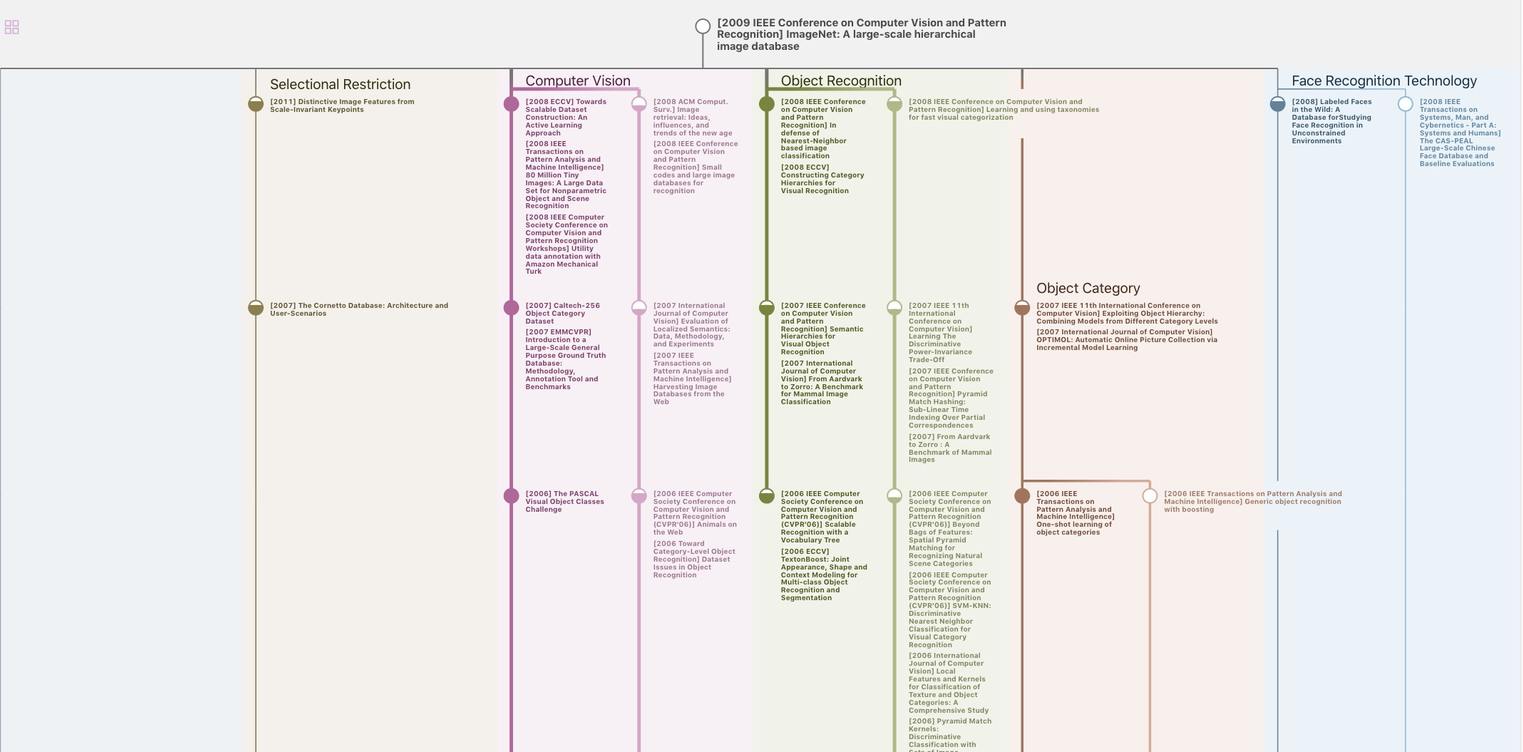
生成溯源树,研究论文发展脉络
Chat Paper
正在生成论文摘要