Spectral masked autoencoder for few-shot hyperspectral image classification
IGARSS 2023 - 2023 IEEE INTERNATIONAL GEOSCIENCE AND REMOTE SENSING SYMPOSIUM(2023)
摘要
Though deep learning methods have achieved the state-of-the-art performance for hyperspectral image (HSI) classification, they often highly rely on large amount of samples for training, and introduce few-shot challenge due to the lack of labeled samples. In this paper, a self-supervised method is presented for HSI classification in the few-shot scenario, where masked autoencoder is employed to reconstruct the masked bands in spectral domain for model pretraining with limited labeled sample, namely Spectral-MAE. The proposed method not only avoids the overfitting via the pretraining, but also provides the model's ability for effective feature extraction while avoiding the high spatial redundancy. Experiments conducted verify the effectiveness of the proposed method for HSI classification in few-shot situation as compared with other methods.
更多查看译文
关键词
Hyperspectral Image Classification,MAE,Few-Shot,Self-Supervised Learning
AI 理解论文
溯源树
样例
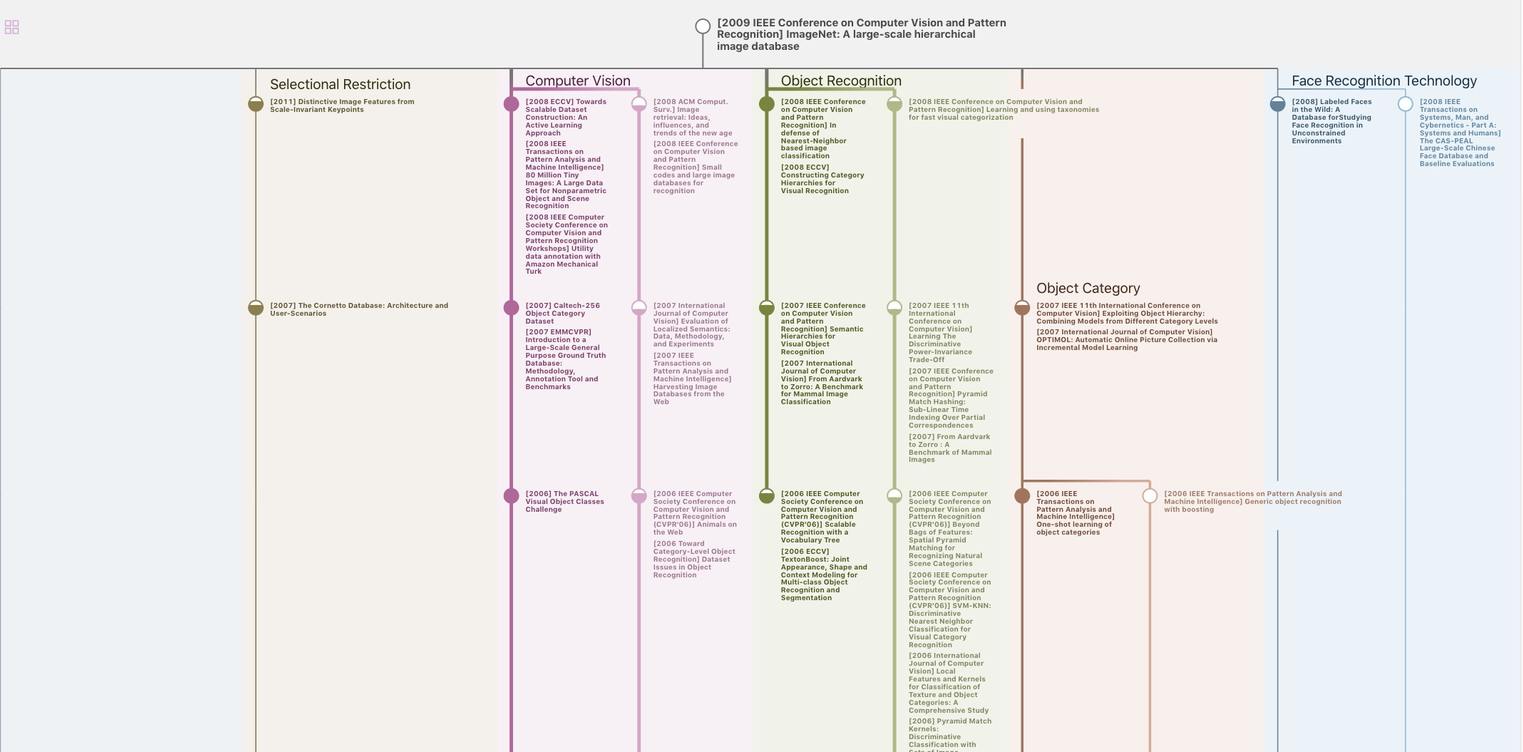
生成溯源树,研究论文发展脉络
Chat Paper
正在生成论文摘要