Toward field level drought and irrigation monitoring using machine learning based high-resolution soil moisture (ml-hrsm) data
IGARSS 2023 - 2023 IEEE INTERNATIONAL GEOSCIENCE AND REMOTE SENSING SYMPOSIUM(2023)
摘要
The field level soil moisture information is critical for crop irrigation, water resource and crop management, crop modeling, and crop yield estimation. USDA National Agricultural Statistics Services (NASS) uses SMAP derived soil moisture data for cropland soil moisture condition monitoring and assessment. However, spatial resolutions of Crop-CASMA soil moisture data are too coarse for field level assessment. Therefore, this paper proposes to use machine learning based high-resolution soil moisture (ML-HRSM) data for field level drought and irrigation monitoring. The preliminary study results show that the ML-HRSM data can accurately delineate field-level soil moisture variations at high spatial (30-m) and temporal (daily) resolutions. It can accurately capture field level soil moisture changes caused by the irrigation activities and vegetation ET processes. The irrigation activities are indirectly confirmed by weekly MODIS NDVI maps. The preliminary results indicate that the surface ML-HRSM is capable for irrigation activity and drought monitoring at field level.
更多查看译文
关键词
ML-HRSM,Crop-CASMA,high resolution soil moisture,irrigation monitoring,cropland
AI 理解论文
溯源树
样例
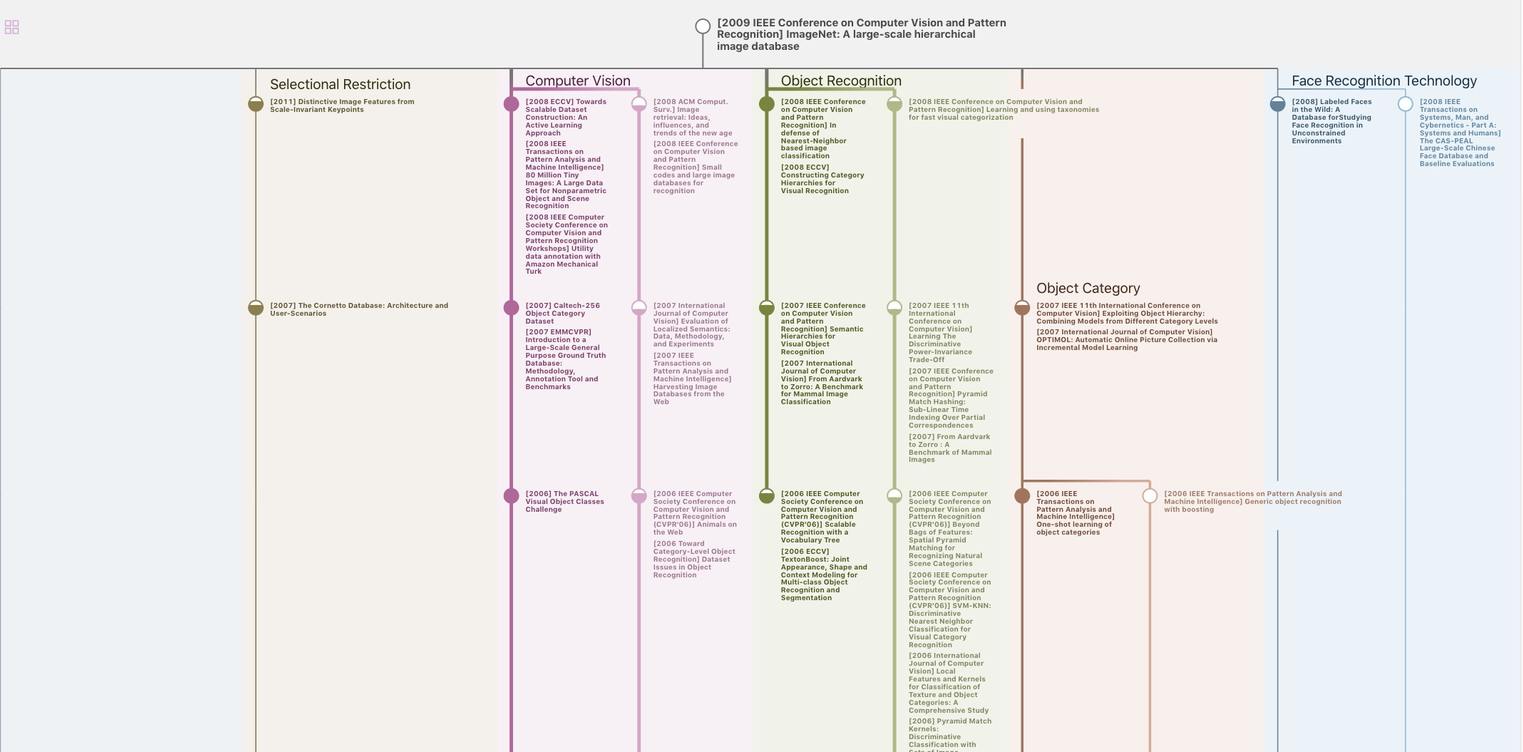
生成溯源树,研究论文发展脉络
Chat Paper
正在生成论文摘要