Influence of Out-of-Distribution Examples on the Quality of Semantic Segmentation in Remote Sensing.
IGARSS 2023 - 2023 IEEE INTERNATIONAL GEOSCIENCE AND REMOTE SENSING SYMPOSIUM(2023)
摘要
Semantic segmentation for land cover maps follows the closed world assumption, where each pixel must be classified into a set of predefined classes. In order to fulfill this assumption, an additional class is usually introduced to describe all areas not covered by the main classes, called "clutter" or "other". Consequently, this class is extremely heterogeneous, and the classification is usually subpar. Using a common approach for uncertainty assessment of land cover classification, we analyze the influence of the clutter class being present or absent during training on the semantic segmentation. We assess the model uncertainties of two different deep learning models, U-Net and DeepLab V3+, and different training configurations by using a Monte-Carlo dropout based uncertainty metric. The corresponding uncertainty maps and histograms show a correlation between clutter class and the uncertainty metric.
更多查看译文
关键词
Semantic segmentation,Monte-Carlo dropout,out-of-distribution,quality assessment,uncertainty
AI 理解论文
溯源树
样例
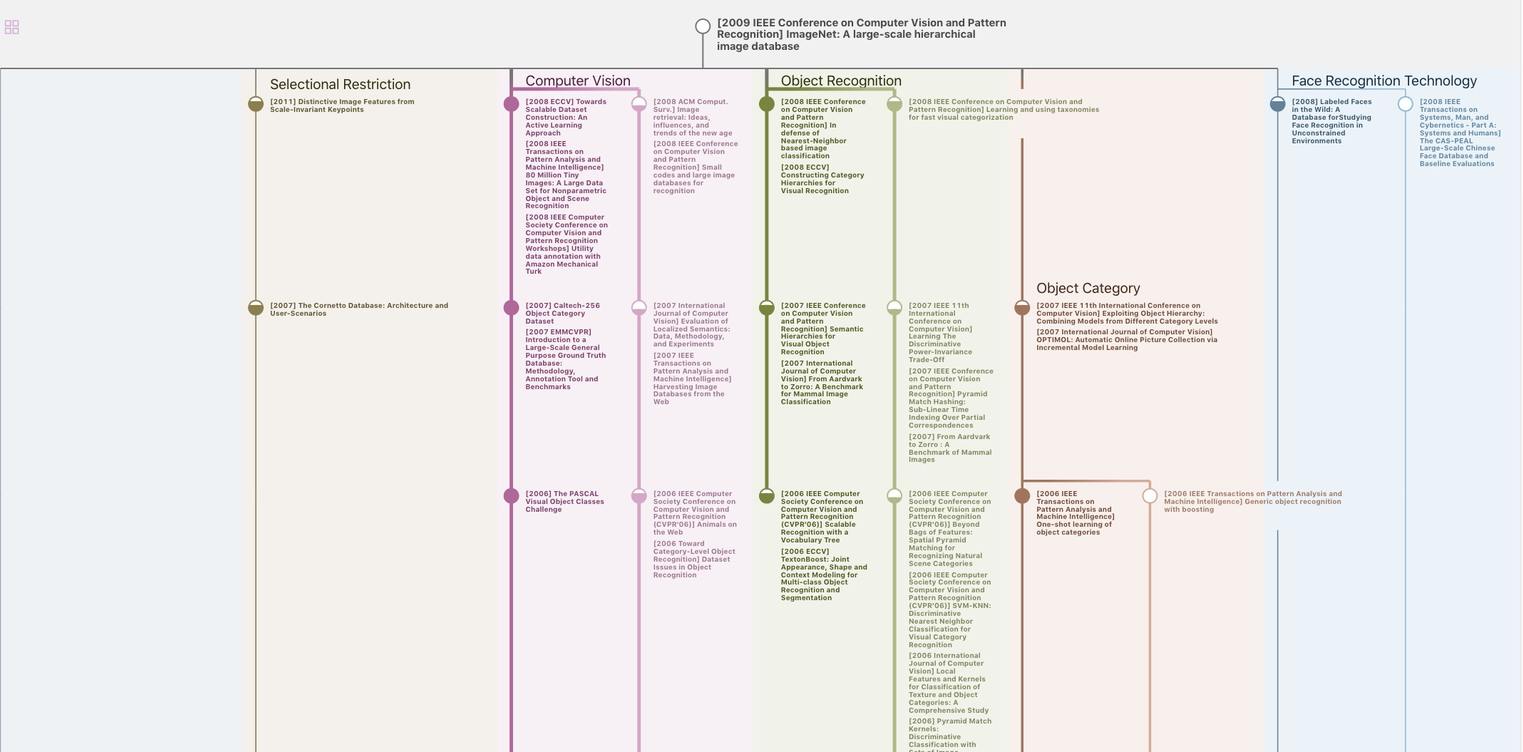
生成溯源树,研究论文发展脉络
Chat Paper
正在生成论文摘要