Machine Learning-Based Approach for Landslide Susceptibility Mapping Using Multimodal Data
IGARSS 2023 - 2023 IEEE International Geoscience and Remote Sensing Symposium(2023)
关键词
conventional ML-based methods,debris flow,Global Deforestation Detection Algorithm,hazard map,inventory Landslide,Landslide Susceptibility Mapping,Machine Learning-based approach,method fuses only three carefully selected factors,multimodal data,multiple data sources,practical Machine,rainfall data,rainfall forecast data,real-world landslide,substantial forest changes,SVM-based classifier,Synthetic Aperture Radar
AI 理解论文
溯源树
样例
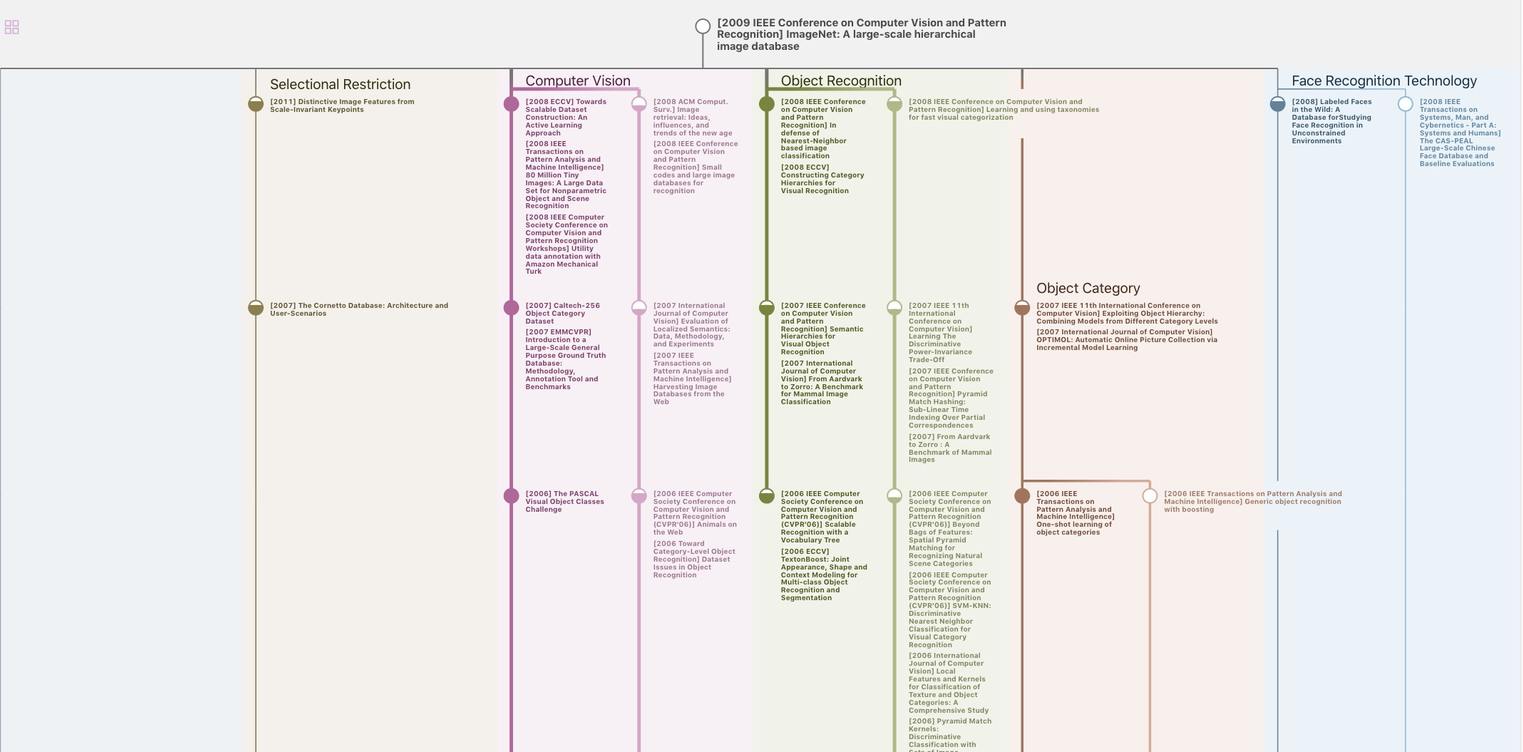
生成溯源树,研究论文发展脉络
Chat Paper
正在生成论文摘要