Semi-supervised learning by domain adaptation for hyperspectral image classification
IGARSS 2023 - 2023 IEEE INTERNATIONAL GEOSCIENCE AND REMOTE SENSING SYMPOSIUM(2023)
摘要
Classification of remotely sensed data is the mainstay of analysis methods for generating actionable insights. Classification of remotely sensed data is inherently a semi-supervised classification problem. Often, the labeled pixels and the unlabeled pixels in the image may have different distribution. Hence classification accuracy of such images is affected. We propose a umbrella framework for semi-supervised learning that considers the domains shifts in labeled and unlabeled pixels (called Domain Aware Semi-supervised learning-DASSL). The method learns the deep features in a such a that they are invariant of the pixel source, i.e, labeled or unlabeled. We employed DASSL for classification of hypersepctral image of Pavia University. We compared DASSL with self-training iterations performed using SVM and Convolutional Neural Network. We used spectral features, spatial features, and fused spectral-spatial features. The results are encouraging. We observed the reasonable improvement in classification by DASSL over self-training iterations.
更多查看译文
关键词
Hyperspectral data,Semi-supervised learning,Domain adaptation,Remote sensing
AI 理解论文
溯源树
样例
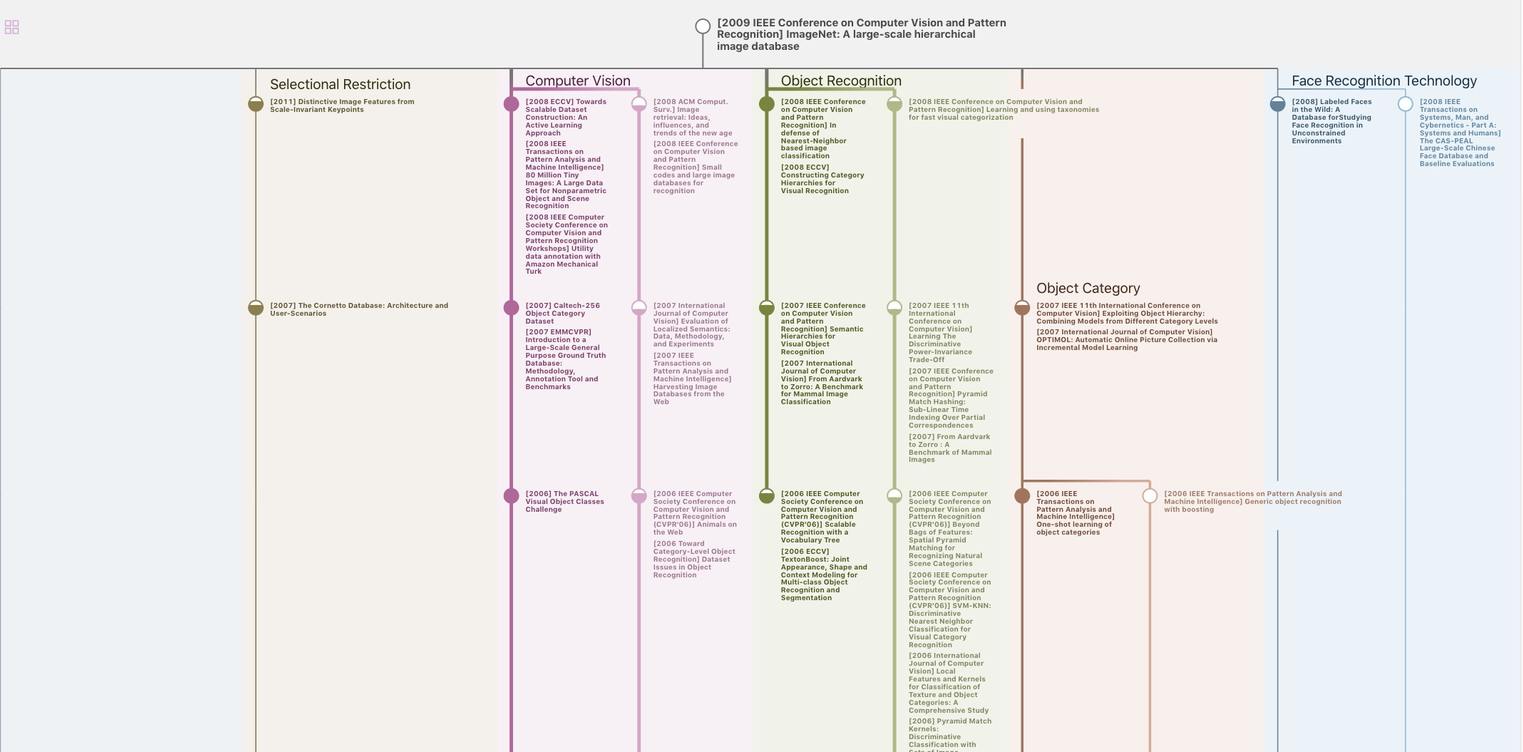
生成溯源树,研究论文发展脉络
Chat Paper
正在生成论文摘要