Multi-parameter retrieval of water quality indicators from bayesian and mixture density networks
IGARSS 2023 - 2023 IEEE INTERNATIONAL GEOSCIENCE AND REMOTE SENSING SYMPOSIUM(2023)
摘要
Machine Learning (ML) models have emerged as powerful and accurate tools for the estimation of the properties of the water column from optical remote sensing data. In particular, machine learning models with a probabilistic output like Mixture Density Networks (MDNs) and Bayesian Neural Network with MC-Dropout (BNN-MC) have emerged as the front-runners for the task of Chlorophyll-a estimation. Another advantage of these models is that they also provide a method to capture the confidence associated with the prediction of the machine learning model for each sample. This paper extends the analysis of the performance of such models to the task of simultaneous estimation of a set of water quality indicators, to provide the water resource managers with a more complete picture on the state of the water column. The paper compares and contrasts the performance of the BNN-MC and MDN in terms of both parameter and uncertainty estimation. Further, the effect of the spectral resolution on the model performance is also analyzed by verifying model performance at both multispectral and hyperspectral sensor-resolutions.
更多查看译文
关键词
Aquatic remote sensing,water quality monitoring,machine learning,multispectral,hyperspectral
AI 理解论文
溯源树
样例
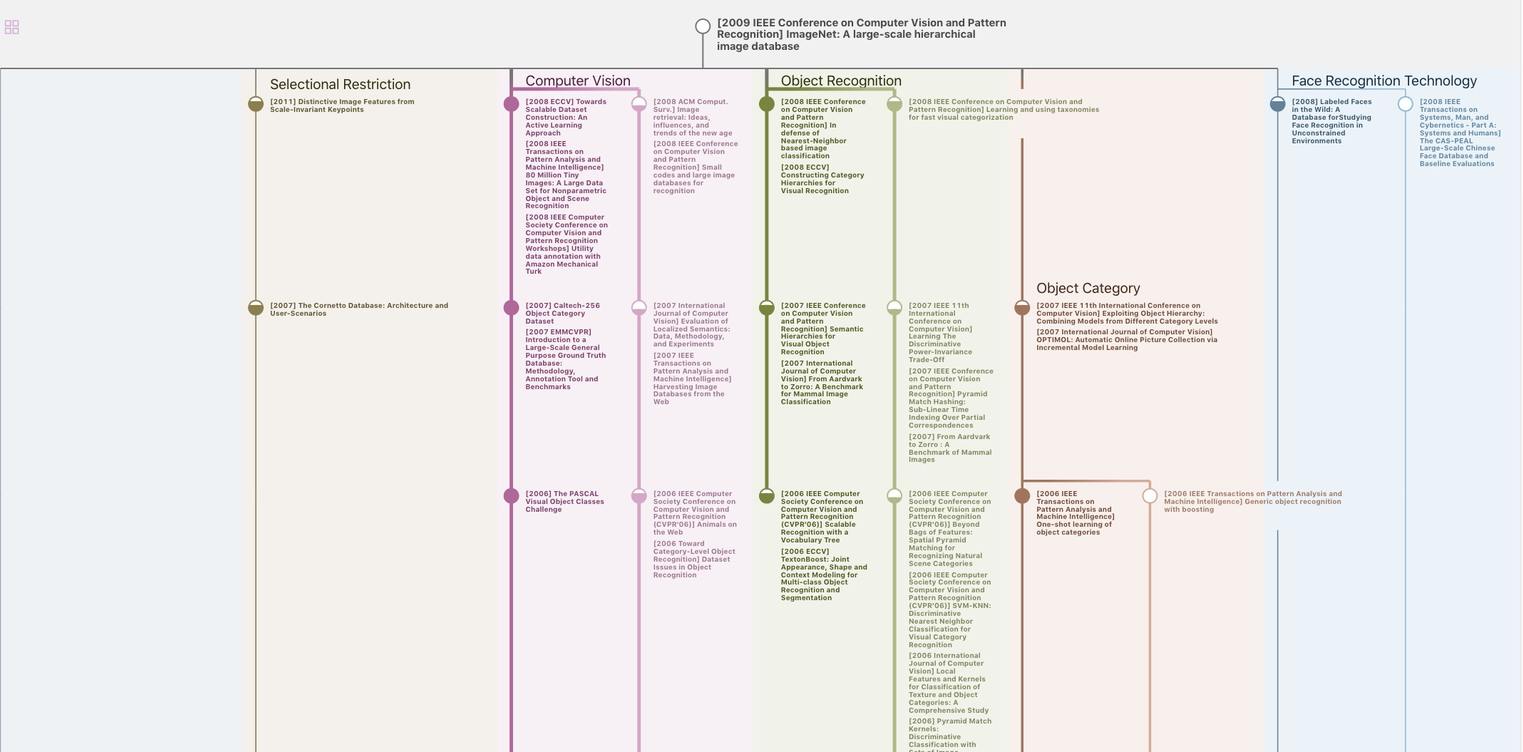
生成溯源树,研究论文发展脉络
Chat Paper
正在生成论文摘要