Light-weighted explainable dual transformer network for hyperspectral image classification
IGARSS 2023 - 2023 IEEE INTERNATIONAL GEOSCIENCE AND REMOTE SENSING SYMPOSIUM(2023)
摘要
Although light-weighted explainable deep learning techniques are critical for operational hyperspectral image (HSI) classification, it is very challenging to achieve these techniques due to difficulties to deal with the spatial-spectral complexity and coupling effect in HSI. Leveraging the excellent feature learning capability of the attention mechanism, this paper presents a spatial-spectral dual transformer (SSDT) network that decomposes the conventional spatial-spectral transformer operation into a spatial transformer and a spectral transformer, which not only reduce the model complexity, but also allows the use of self-attention to explain feature relevance. The proposed approach is tested on some benchmark HSI scenes and the results demonstrate that the proposed dual transformer network not only achieves new state-of-the-art performance due to its excellent feature extraction capability, but also enables the analysis and visualization of feature importance and decision making process.
更多查看译文
关键词
Hyperspectral image classification,transformer,spatial-spectral analysis,explainable neural network,feature importance evaluation,light-weighted models
AI 理解论文
溯源树
样例
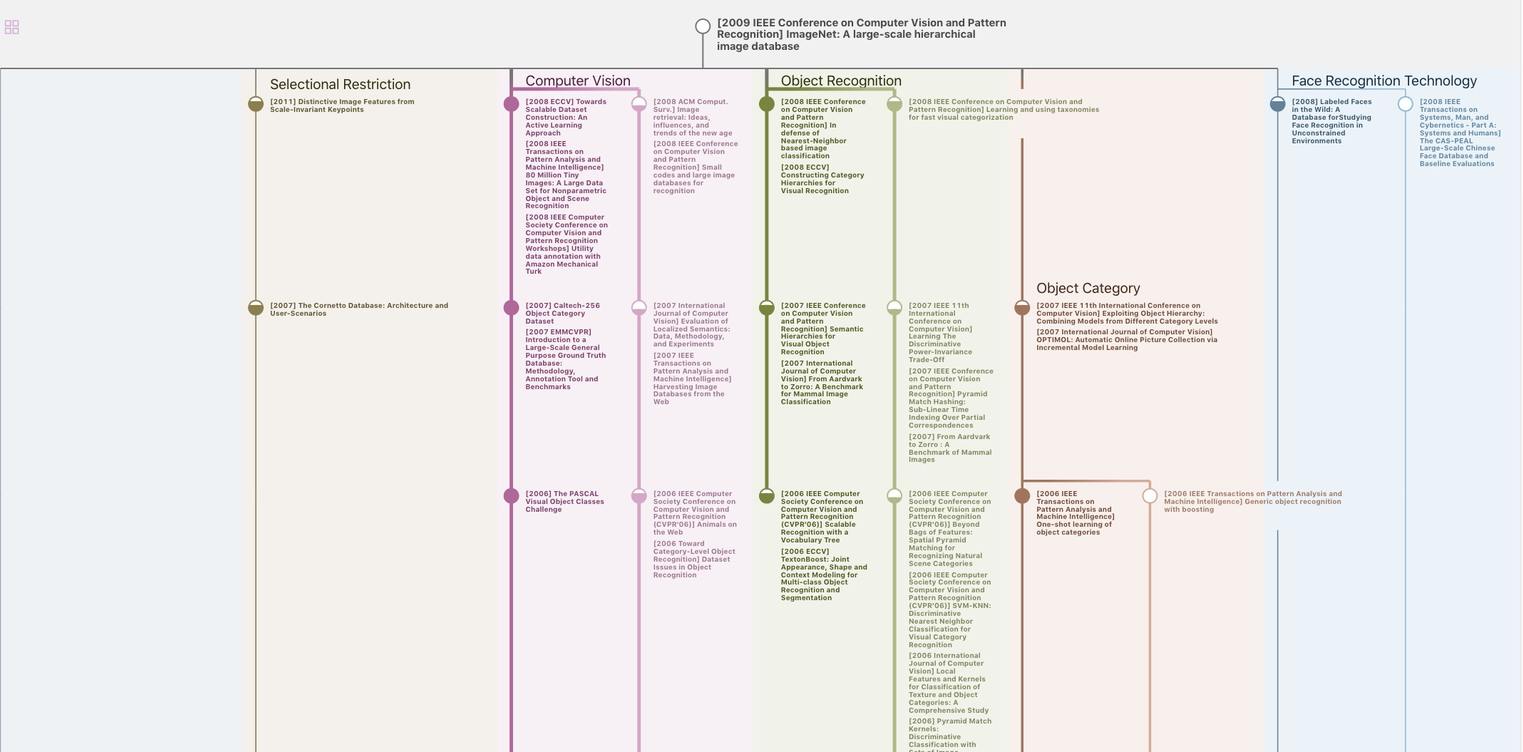
生成溯源树,研究论文发展脉络
Chat Paper
正在生成论文摘要