A hyperspectral change detection method based on active learning strategy
IGARSS 2023 - 2023 IEEE INTERNATIONAL GEOSCIENCE AND REMOTE SENSING SYMPOSIUM(2023)
摘要
In recent years, deep learning has demonstrated its transformative potential in the field of hyperspectral image (HSI) processing but is notoriously data-hungry. However, wanting to obtain a large number of labels is labor-intensive and time-consuming. To reduce the dependence of the model on the label samples while maintaining high detection accuracy, a hyperspectral image change detection algorithm based on active learning strategy (ALCD) is proposed. First, the active learning strategy is employed to select high-value labeled samples from the test set as additional training data, gradually enhancing the model's detection performance. Second, the self-attention module MOAT is introduced to enable effective interaction of local information during the feature extraction process and enhance the network's feature expression capability. Then, the feature interaction and the mixing block are used to blend the features of the bitemporal images, so that the feature distribution of the bitemporal images is more similar, which is conducive to subsequent feature extraction and classification. Experiments on two HIS datasets show that the proposed method can obtain better change detection results than the four comparison algorithms.
更多查看译文
关键词
Hyperspectral image,change detection,active learning
AI 理解论文
溯源树
样例
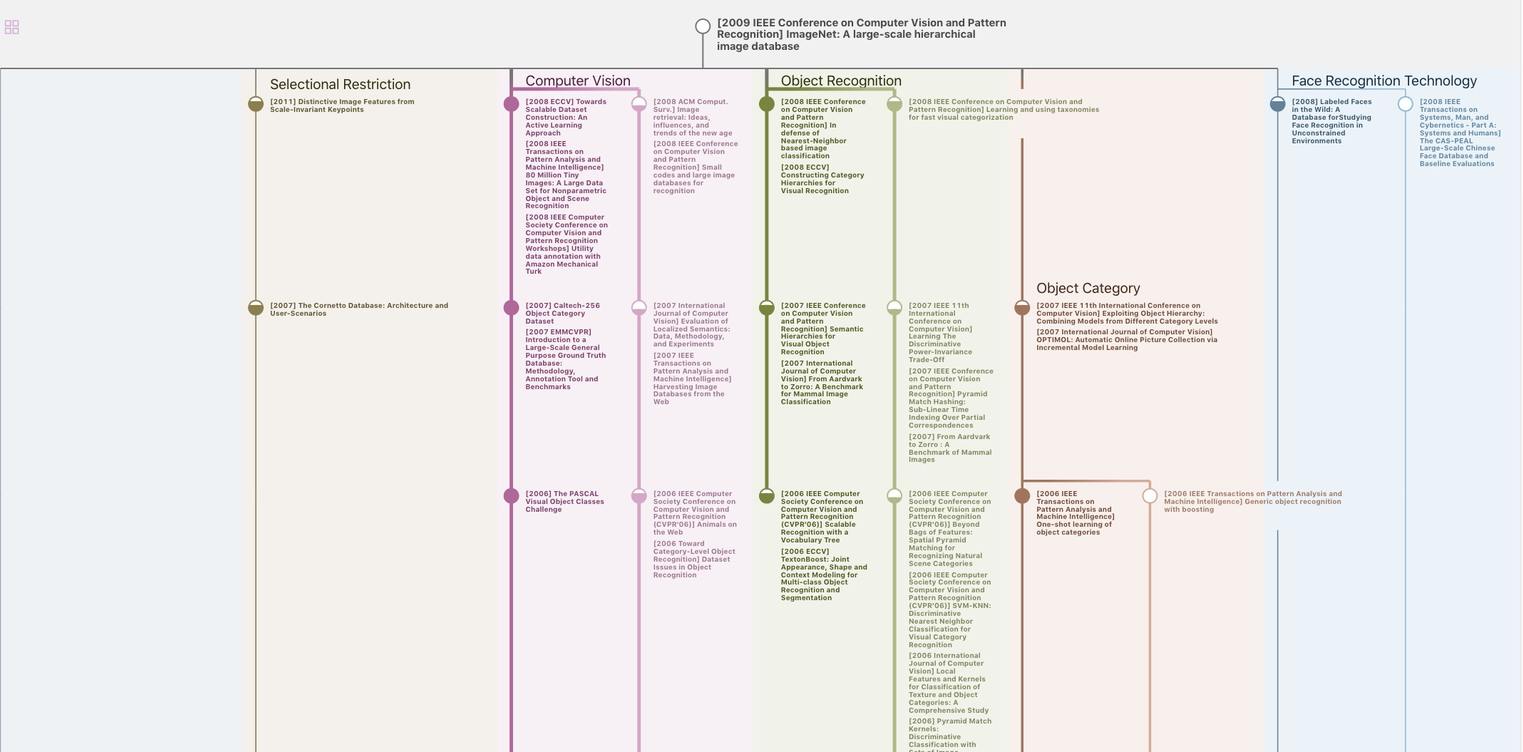
生成溯源树,研究论文发展脉络
Chat Paper
正在生成论文摘要