Edge-guided feature dense fusion network for remote sensing image change detection
IGARSS 2023 - 2023 IEEE INTERNATIONAL GEOSCIENCE AND REMOTE SENSING SYMPOSIUM(2023)
摘要
Remote sensing change detection (CD) is of great importance to Earth observation. Recently, Deep Learning (DL) has been increasingly used to extract useful features and make accurate decisions in a large number of remote sensing images, due to its ability to automatically learn semantic features. However, insufficient fusion of bitemporal images and the lack of prior knowledge of edge structures in current DL methods will result in inaccurate CD results, especially for building boundaries. To alleviate these problems, an edge-guided feature-densely-fused network (EGFDFN) is proposed in this paper. In contrast to conventional Siamese networks, EGFDFN extracts bitemporal features from an extra dual decoder instead of a dual encoder to obtain more accurate change features. In addition, an attention and dense fusion module (ADFM) and an edge guidance module (EGM) are used to enhance features and make full use of edge information. Experimental results demonstrate that the proposed method outperforms on LEVIR-CD dataset among other representative methods.
更多查看译文
关键词
Change detection (CD),dual decoder,dense fusion module (DFM),edge guidance module (EGM),remote sensing
AI 理解论文
溯源树
样例
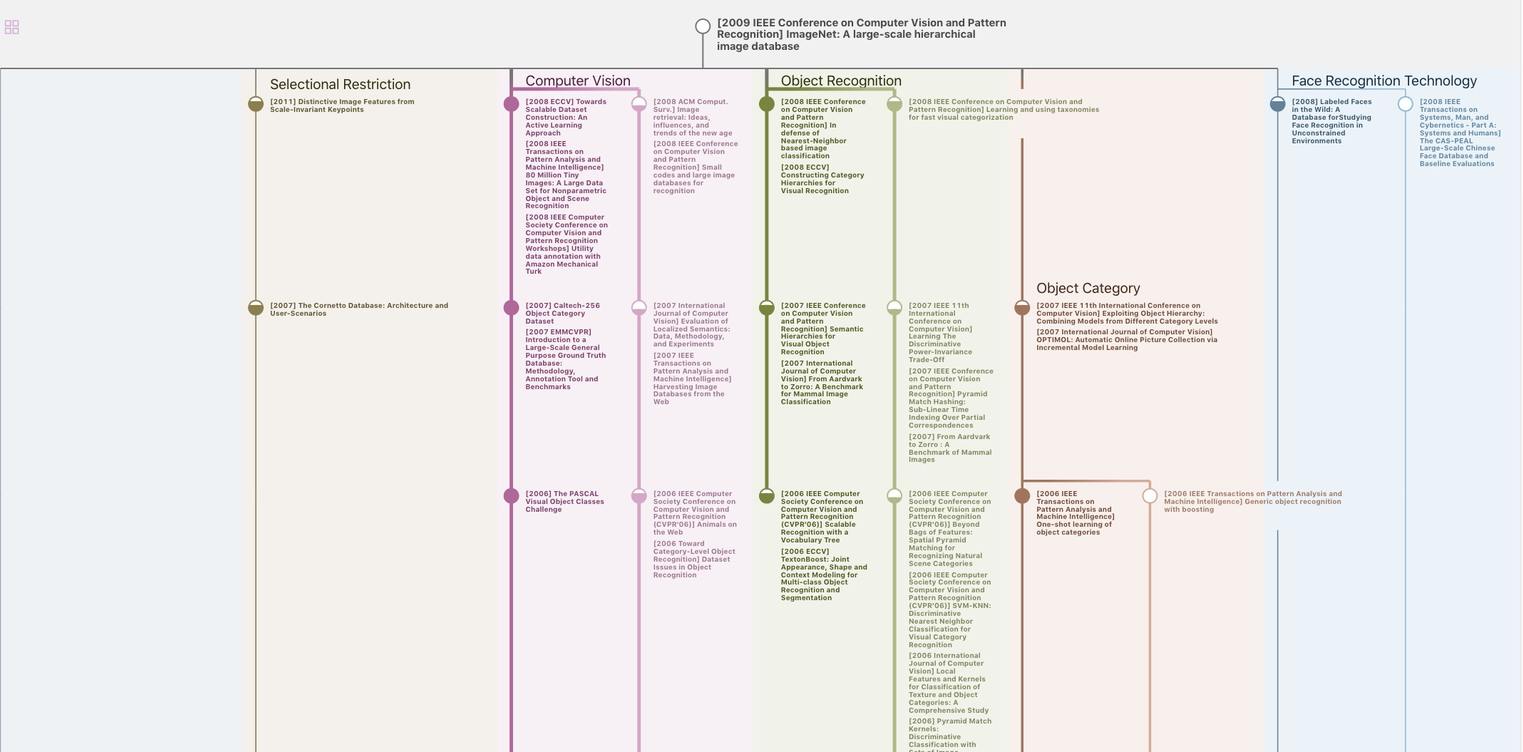
生成溯源树,研究论文发展脉络
Chat Paper
正在生成论文摘要