Performance comparison of multispectral channels for land use classification
IGARSS 2023 - 2023 IEEE INTERNATIONAL GEOSCIENCE AND REMOTE SENSING SYMPOSIUM(2023)
摘要
Land cover classification using satellite imagery plays a crucial role in monitoring changes on the earth's surface. This paper presents an analysis of the EuroSAT dataset using state-of-the-art deep learning models to benchmark the impact of additional bands on classification accuracy. The dataset consists of 27,000 images across 10 classes captured by the Sentinel-2 satellite, including RGB and multispectral bands. Performance evaluation was conducted using popular convolutional neural network models based on Resnet variants and Vision Transformer (ViT). The results show that the combination of all bands achieved the highest accuracy, with ResNet-152 achieving a validation accuracy of 96.63% on the multispectral dataset. Precision, recall, and F1 scores were also utilized to assess the models' performance. The findings highlight the significance of incorporating additional bands for improved classification accuracy in satellite image analysis.
更多查看译文
关键词
Land cover classification,Satellite imagery,EuroSAT dataset,Sentinel-2 satellite
AI 理解论文
溯源树
样例
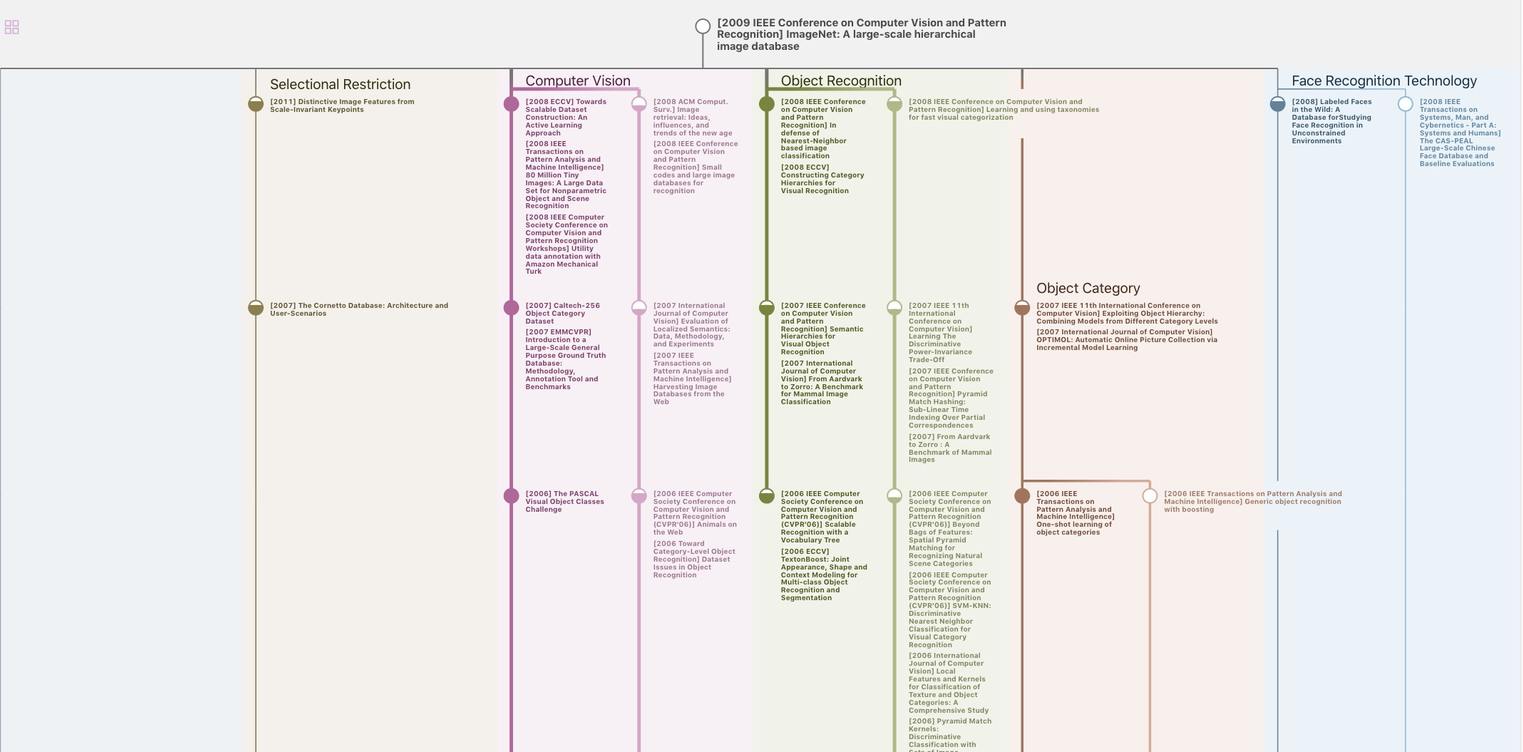
生成溯源树,研究论文发展脉络
Chat Paper
正在生成论文摘要