Self-supervised deep network for automatic target recognition in sar
IGARSS 2023 - 2023 IEEE INTERNATIONAL GEOSCIENCE AND REMOTE SENSING SYMPOSIUM(2023)
摘要
In this paper, for the very first time, a self-supervised deep neural network (pretext & downstream) is devised for automatic target recognition (ATR) in synthetic aperture radar (SAR) images. We observe that the SAR employs side look image acquisition that makes the resultant images orientation sensitive. Using this fact, we propose orientation estimation as a novel pretext task to train for ATR downstream using MSTAR dataset. A 6-layer convolutional neural network (CNN) is designed to estimate the orientation of input SAR as probability vector. Thus obtained target orientation context knowledge is distilled to our downstream model where a 21-layer deep CNN is designed to perform ATR with a limited labelled dataset. This network consists of 4-pairs of convolution + maxpooling layers, followed by 9 dense layers with 2 residual connections. The network is designed to enhance ATR capability while avoiding shattering gradients. Experiments are conducted on real MSTAR images. Ablation study is performed with different number of training images vs. different pretext tasks integrated with ATR downstream. We found proposed network recognizes the targets with 98.90% accuracy (4-fold cross validated) with just one-third labelled dataset which makes it promising for on-board ATR.
更多查看译文
关键词
Automatic target recognition,Self-supervised deep learning,Synthetic aperture radar
AI 理解论文
溯源树
样例
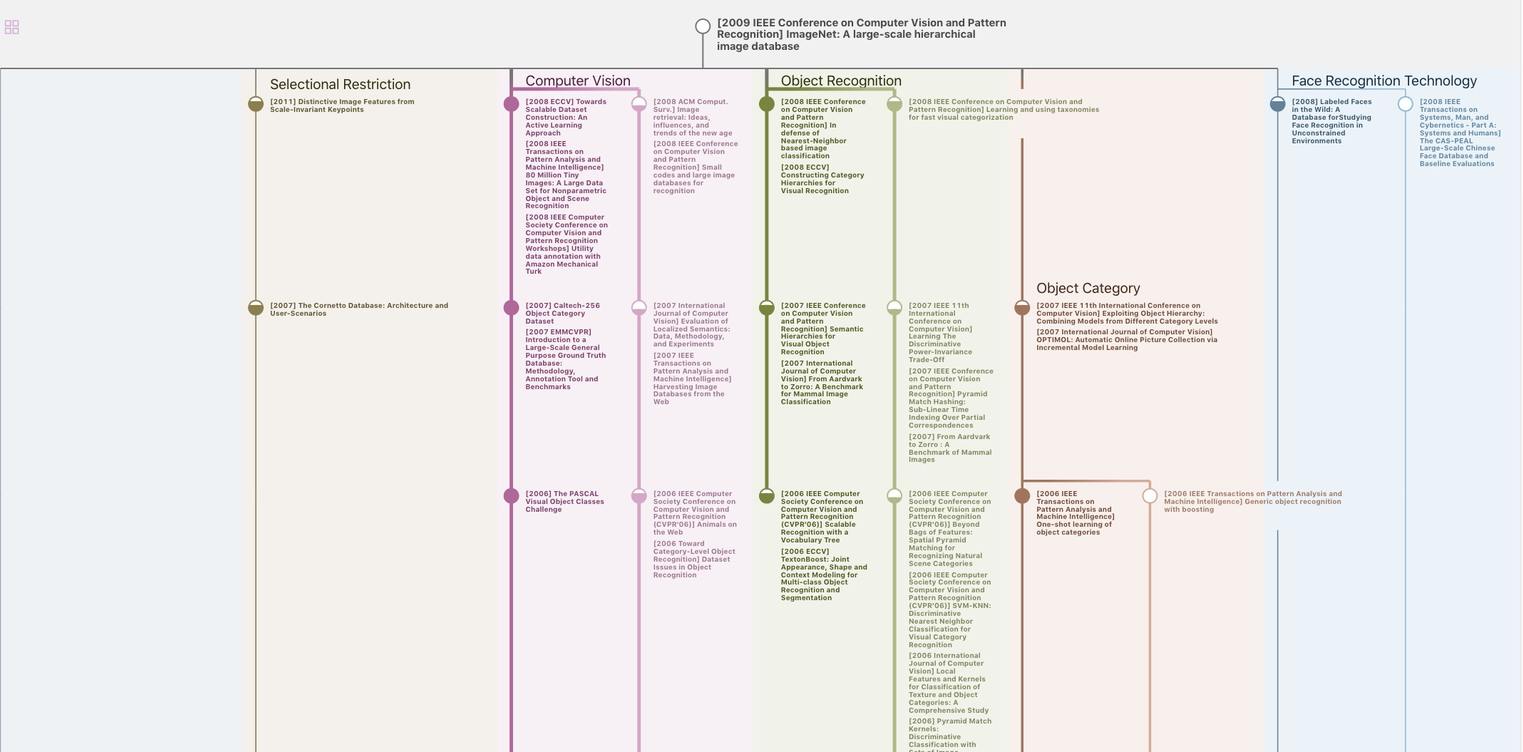
生成溯源树,研究论文发展脉络
Chat Paper
正在生成论文摘要