Homomorphic Encryption on Deep Learning in accurate prediction of Brain Tumour
2023 International Conference on Next-Generation Computing, IoT and Machine Learning (NCIM)(2023)
摘要
Data breach and data theft has become a repulsive problem in the digital world and medical data is the most vulnerable in this case as it contains numerous information of patients which can hinder a patient's personal life. Moreover, due to patients' rights and confidentiality, hospital's are unwilling to share the medical data to third parties. Also, Brain tumour is one of the most occurring disease as everyday, around the globe vast amount of MRI image are taken. Many existing models have been introduced throughout the decades to detect brain tumors, mostly based on Neural Network models. To secure the MRI images and protect patient rights, we have worked on a technique based on Partial Homomorphic Encryption (PHE) which produces encrypted images while keeping most of the features intact. As a result, existing NN models can produce a higher accuracy by extracting features from the encrypted images and also reduces the time complexity of established Paillier cryptosystem. We were able to achieve
$\max$
82% accuracy using a VGG-19 model. Using our proposed model we can safely and securely read encrypted medical image data via our PHE method while being efficient enough to be used on a mass scale in the medical industry.
更多查看译文
关键词
Deep Learning,Neural Networks,Homo-morphic encryption,VGG16,VGG19,Paillier Cryptosystem,ResNet50
AI 理解论文
溯源树
样例
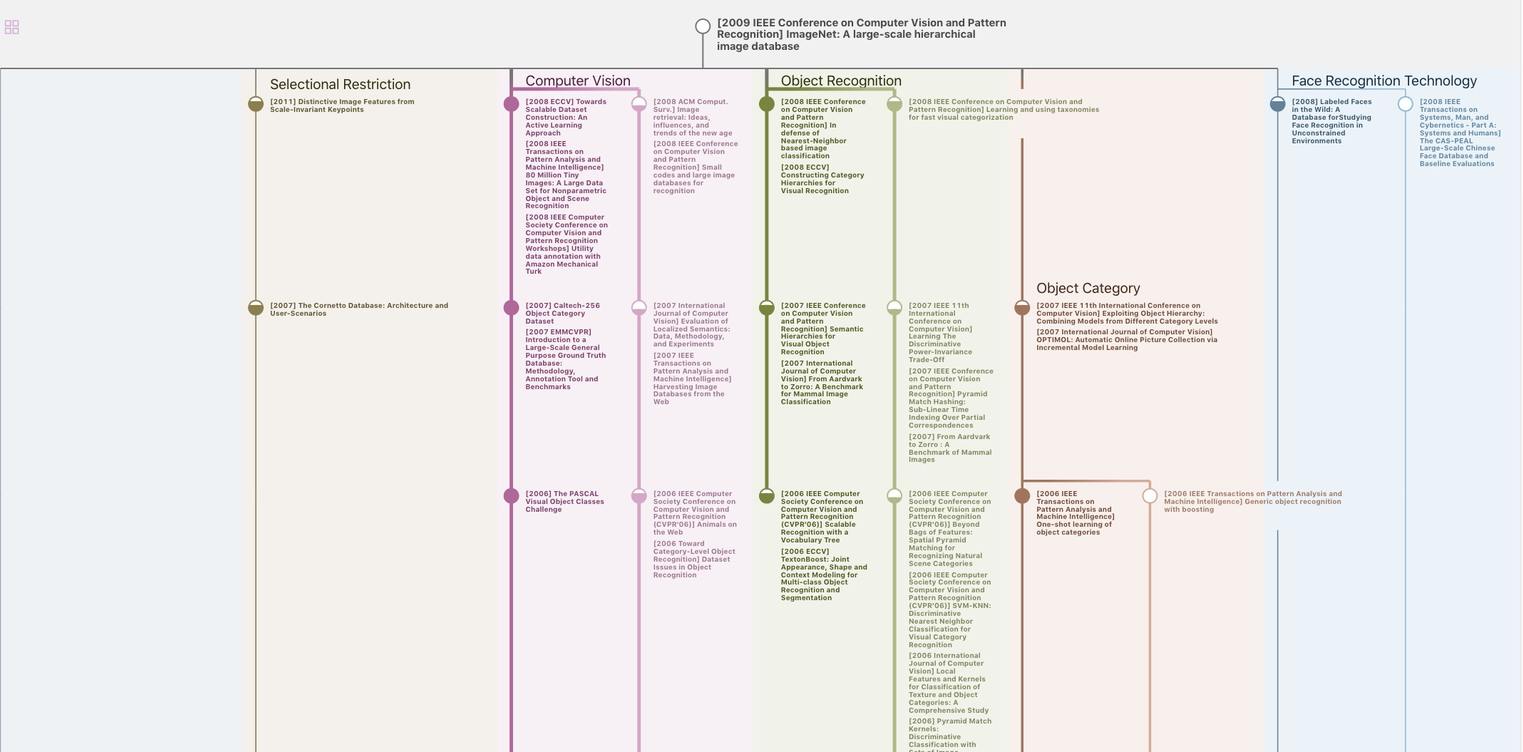
生成溯源树,研究论文发展脉络
Chat Paper
正在生成论文摘要