Surface Anomaly Detection Based on Multi-Scale Feature Propagation and Normalized Flow
2023 IEEE 16th International Conference on Electronic Measurement & Instruments (ICEMI)(2023)
摘要
Unsupervised learning is widely used for surface anomaly detection, due to the sparse and difficult description of anomaly samples. The goal of this paper is to improve the problems of low accuracy and slow speed in unsupervised anomaly detection algorithms. Starting off, the pre-trained convolutional neural network with large dataset is used for feature coarse extraction. Then, a multiscale feature propagation architecture is constructed to perform feature fusion for features at different spatial locations to enhance features coarse extraction of anomaly. Finally, the extracted high-dimensional feature embeddings are mapped to the latent space using normalized flow for distribution fitting, and the likelihood of anomaly existence is calculated using the latent distribution. As a result, our empirical study on the MVTec_AD dataset show that the detection accuracy of our method exceeds baselines, and our method has a faster inference speed. The ablation experiments also demonstrate the effectiveness of the multiscale feature propagation architecture, especially on enhancing the model's ability to detect tiny anomalies. In addition, our approach includes a faster inference speed and less space consumption.
更多查看译文
关键词
anomaly detection,unsupervised learning,pre-training network,multi-scale feature propagation,normalizing flow
AI 理解论文
溯源树
样例
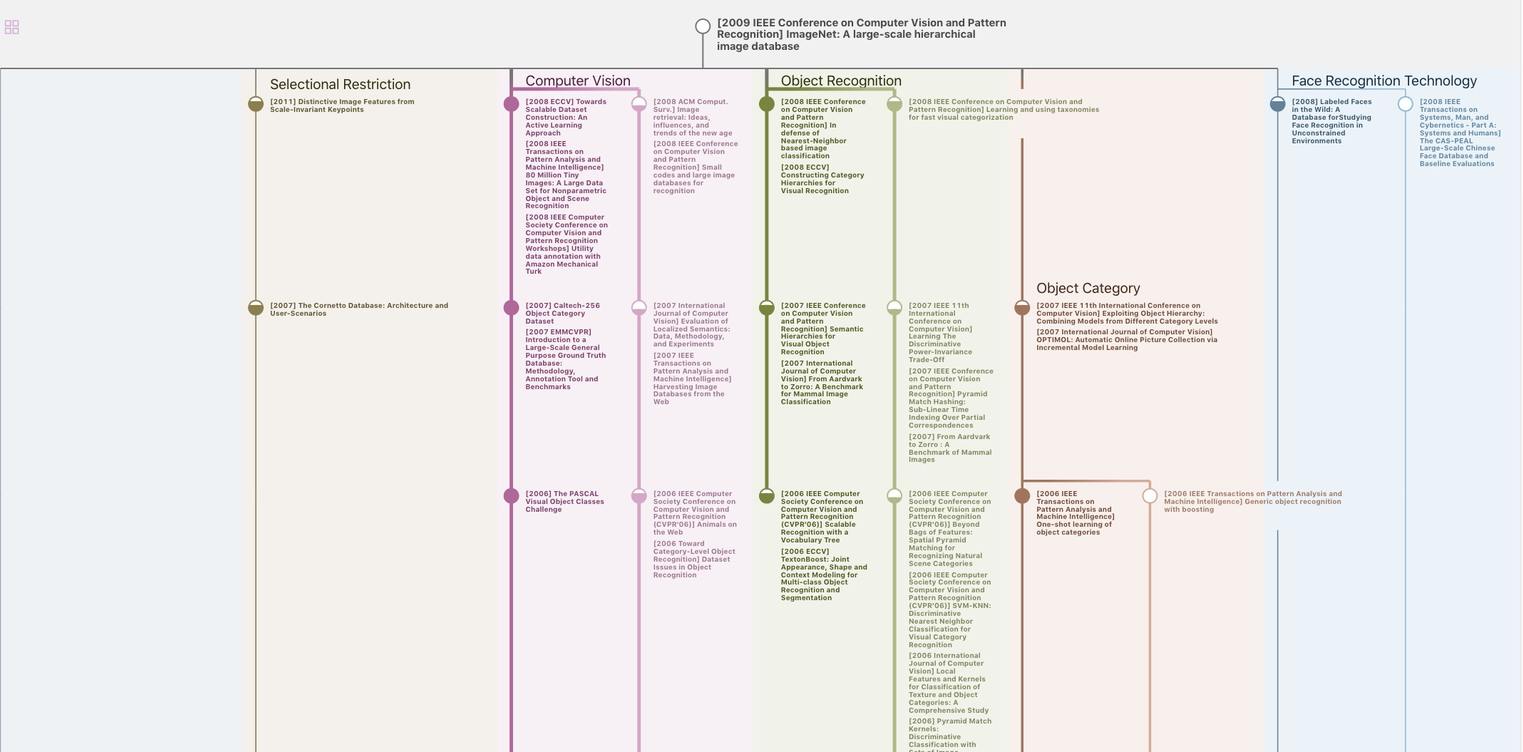
生成溯源树,研究论文发展脉络
Chat Paper
正在生成论文摘要