Predicting Player Performance in Tactical Games with the Lightgbm Algorithm.
2023 IEEE International Conference on Multimedia and Expo Workshops (ICMEW)(2023)
摘要
The accurate prediction of player performance in tactical games is crucial for game designers and developers to improve matchmaking, game balancing, and player engagement. This study presents an approach to predict player performance in Tactical Troops: Anthracite Shift game using the Light Gradient Boosting Machine (LightGBM) algorithm, a scalable and efficient gradient boosting framework. Following data preprocessing, we trained and optimized the LightGBM model using appropriate objective functions and hyperparameter tuning. The proposed approach demonstrated good results in predicting player performance. Our findings highlight the potential of using the LightGBM algorithm in predicting player performance in tactical games, providing valuable insights for game developers to enhance matchmaking, skill assessment, and overall gaming experiences.
更多查看译文
关键词
computer game prediction,gradient boosting decision tree,LightGBM,data mining competitions
AI 理解论文
溯源树
样例
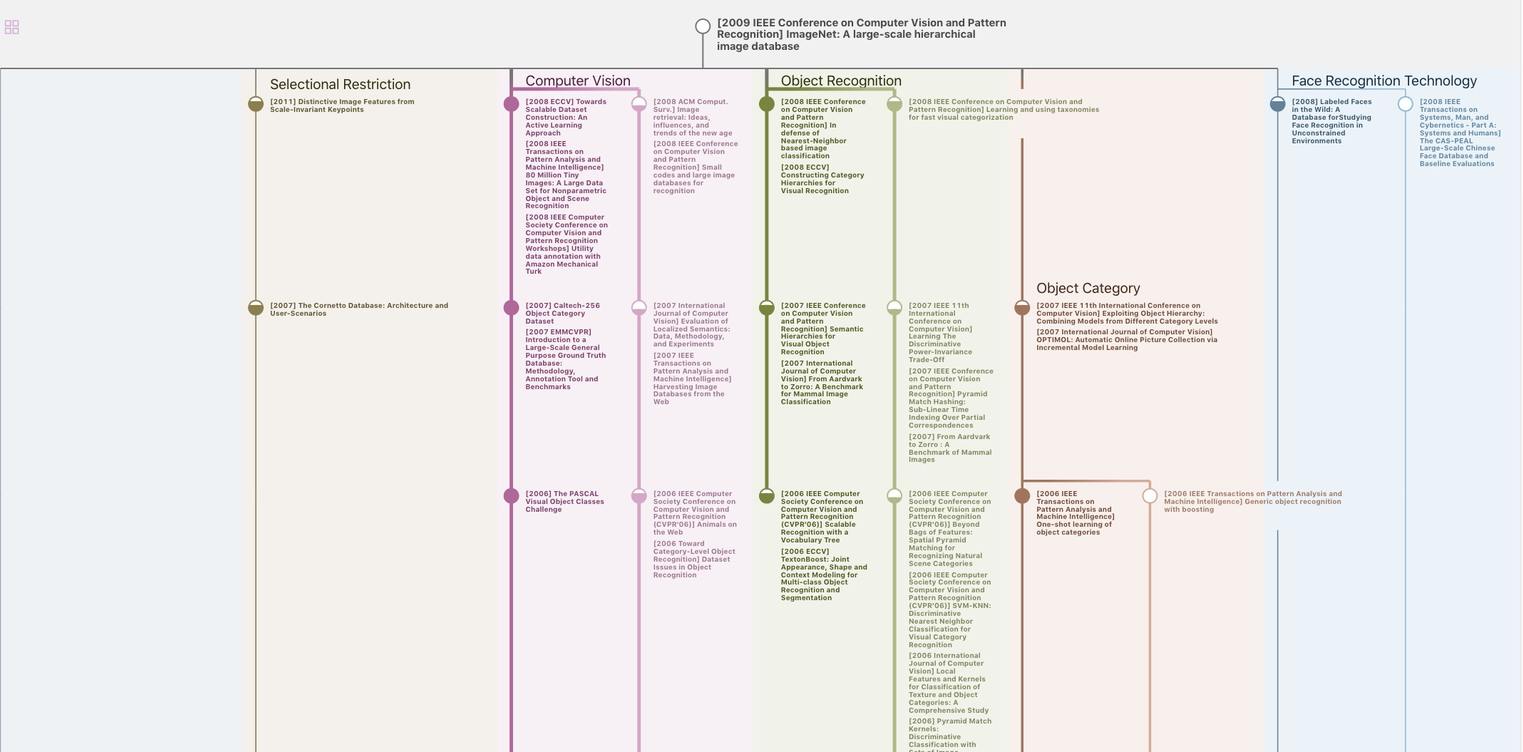
生成溯源树,研究论文发展脉络
Chat Paper
正在生成论文摘要