Autoencoder-Based iEEG Signal Classification for Accurate Focal and Non-focal Epilepsy Detection
2023 4th International Conference on Electronics and Sustainable Communication Systems (ICESC)(2023)
摘要
Intracranial Electroencephalography (iEEG) signals capture abnormal brain neuronal activity and are widely employed in epilepsy diagnoses. The neurologist must visually inspect the iEEG data to diagnose a patient using the traditional method, which takes time and has a high risk of inaccuracy. It is crucial to distinguish between the non-focal and focal classes to find the epileptogenic zone. To classify between focal and non-focal epileptic seizures, an automated method is therefore required. This study explores the potential for automatically learning features from raw iEEG data sufficiently representative for seizure detection. This study recommends classifying iEEG signals using a variety of classifiers like support vector machine (SVM), K Nearest Neighbors (KNN), multi-layer perceptron (MLP), random forest (RF) and Naïve Bayes (NB) with a classification accuracy of 93.3%, 92.66%, 93.33%, 95.99%, and 93.33% and an autoencoder. This method is used to help reduce the sample rate and improve detection effectiveness. In this proposed model, the highest accuracy achieved was 95.99%, with the developed autoencoder model using an RF classifier successfully distinguishing between the iEEG signals of epilepsy.
更多查看译文
关键词
Intracranial Electroencephalography signals,Autoencoders,Focal &,Non-focal,Classification
AI 理解论文
溯源树
样例
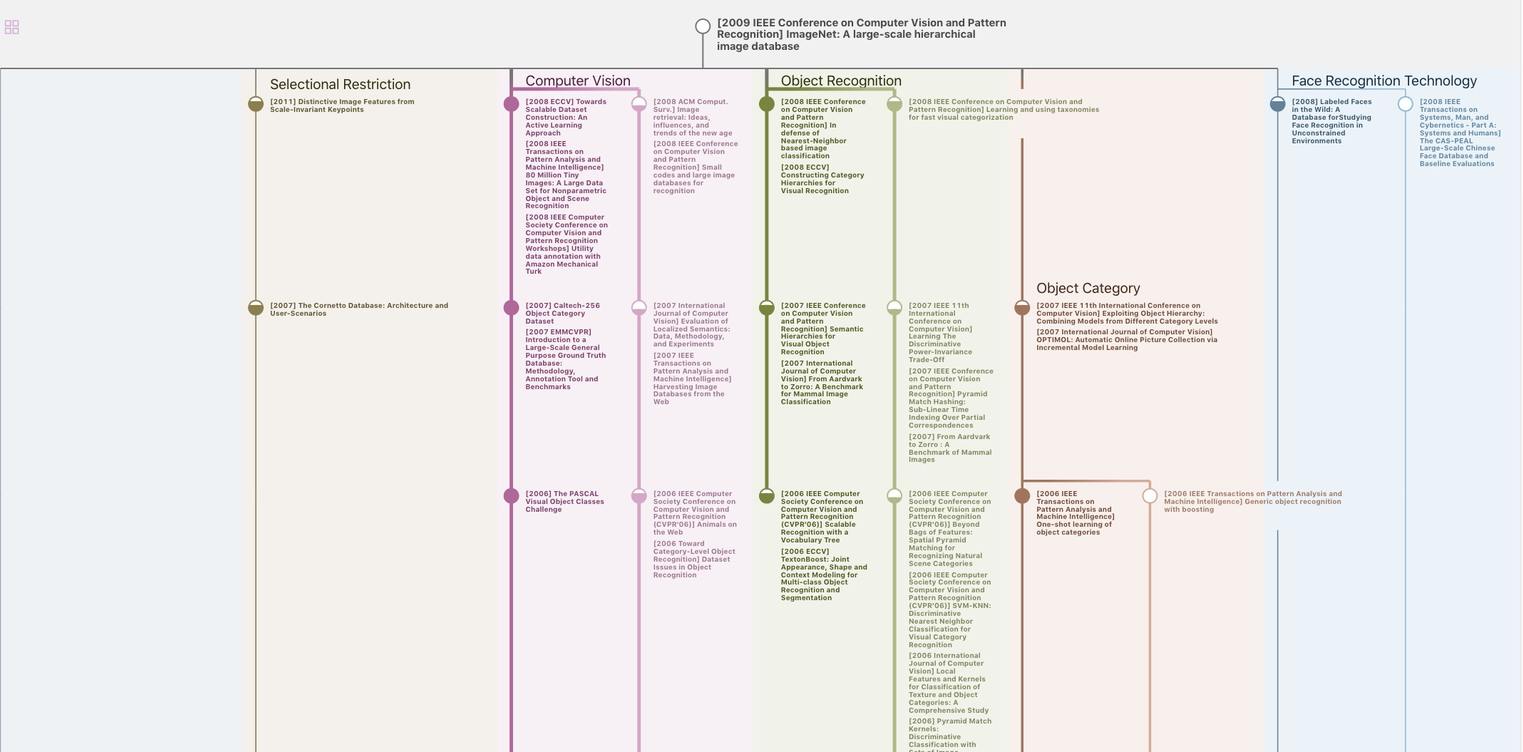
生成溯源树,研究论文发展脉络
Chat Paper
正在生成论文摘要