Bidirectional LSTM Based Sequence Classification Approach for Human Presence Detection Using Channel State Information
International Conference on Microwave, Optical and Communication Engineering(2023)
摘要
In the recent years, deep learning techniques have received a great attention in a wide range of practical problems, including the event detection, localization, classification, and prediction. In this paper, we present a real-time bidirectional long-short term memory (BiLSTM) based human presence sensing using the WiFi channel state information (CSI) feature for non-intrusive occupancy detection systems in indoor environments by selecting best hyper-parameters. In this study, we created real-time Wi-Fi enabled Raspberry Pi computing platform to collect CSI datasets for training and testing purposes by considering different room sizes, fixed objects and different activities. On the wide variety of CSI datasets, evaluation results showed that the proposed magnitude CSI-BiLSTM based human presence detection (HPD) method had an average sensitivity (SE) of 92.23 %, specificity (SP) of 91.18 % and accuracy (ACC) of 91.68% for the 20% test CSI segments taken from the same training dataset and had an average SE of 85.71 %, SP of 91.59% and ACC of 88.67% on the unseen CSI dataset collected under different room conditions. In real-time Raspberry Pi testing environment, the proposed method had an average SE of 99.35%, SP of 94.39 % and ACC of 97.00 %. The proposed magnitude CSI-BiLSTM based HPD method had an average computational time of 1.37 ms with a model size of 509.67 kB.
更多查看译文
关键词
WiFi Based Human Sensing,Bidirectional LSTM,Channel State Information,Device-Free Human Presence Sensing,Non-Intrusive Occupancy Detection
AI 理解论文
溯源树
样例
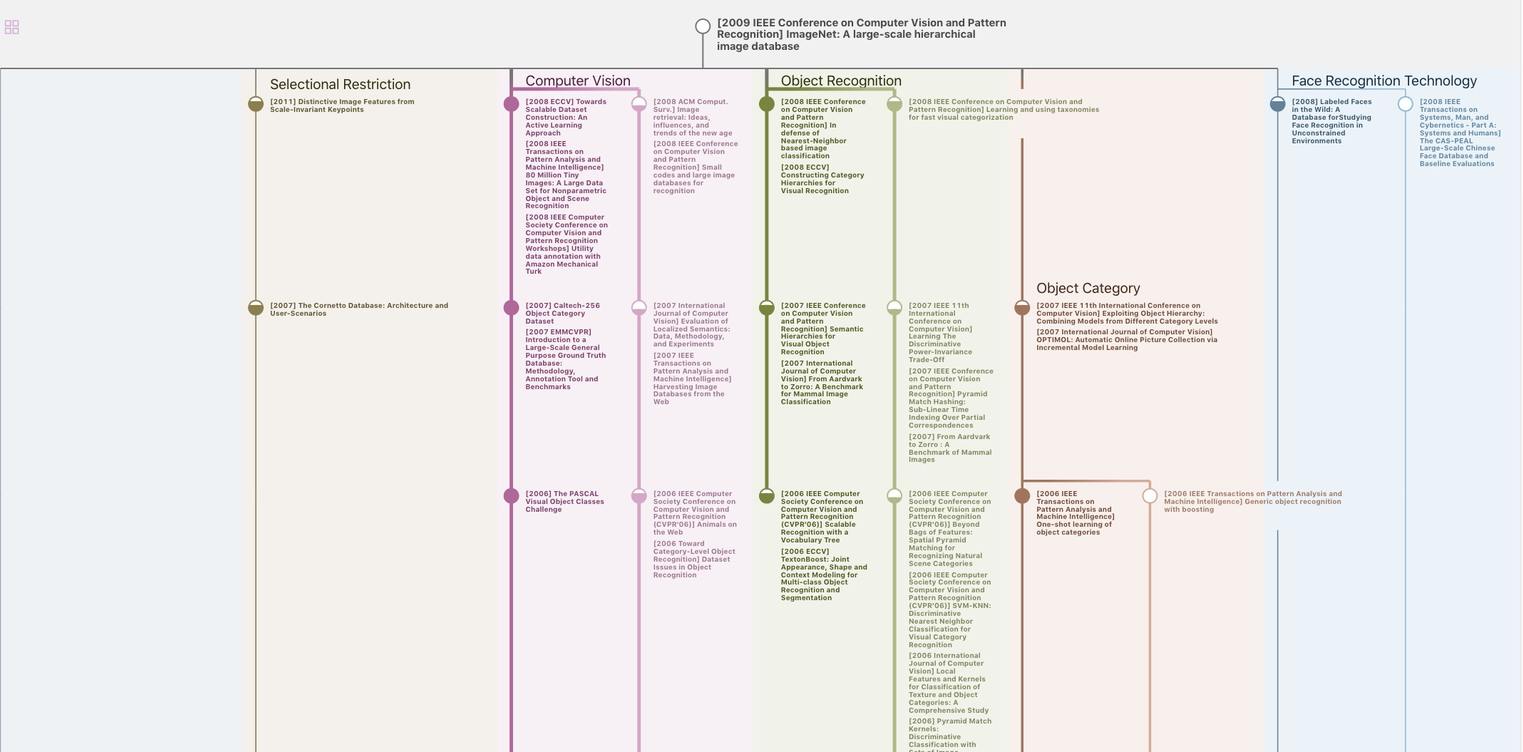
生成溯源树,研究论文发展脉络
Chat Paper
正在生成论文摘要