A Deep Learning Space Mapping Based Enhancement of Compact Models for Accurate Prediction of Trapping in GaN HEMTs from DC to mm-Wave Frequency
2023 IEEE/MTT-S International Microwave Symposium - IMS 2023(2023)
摘要
In this work, a deep learning space mapping technique has been developed to enable standard compact models to account for sporadic trapping effects in AlGaN/GaN high electron mobility transistors (HEMTs). In the proposed technique, an artificial neural network (ANN) is used to map the input feature space spanning the geometrical, material, bias, and trap-related parameters of a rigorous physics-based model (fine model) of the HEMT to the input feature space of a compact model (coarse model). Consequently, the space mapping augmented compact model is able to retain the high computational efficiency of the standard compact model while gaining the capacity to account for the effects of interface and bulk traps in the HEMT responses from DC to millimeter wave frequencies. In this work, the compact model considered is the industry standard advanced SPICE model for GaN HEMTs (ASM-HEMT).
更多查看译文
关键词
Admittance parameters,artificial neural networks,bulk and interface traps,compact model,coarse model,fine model,deep learning,space mapping
AI 理解论文
溯源树
样例
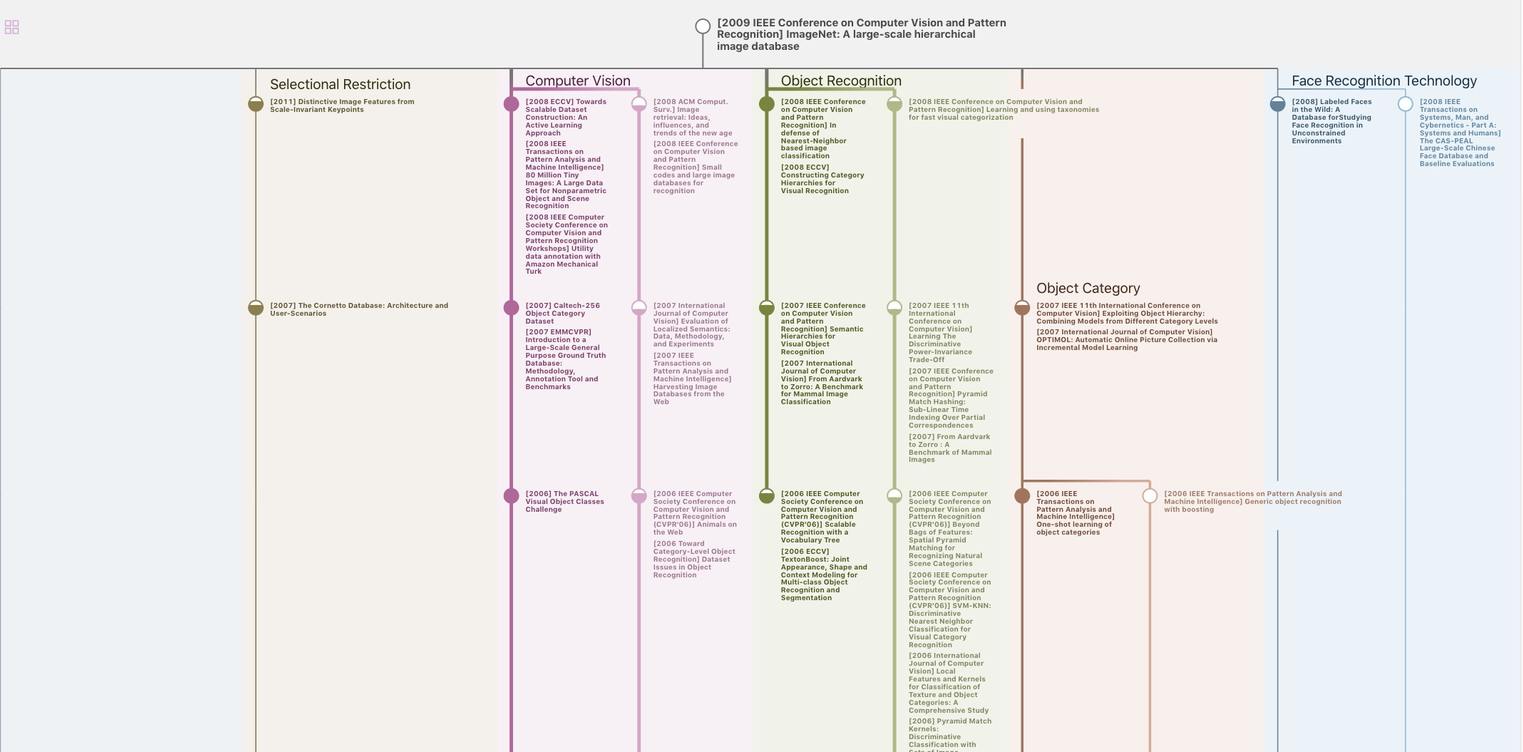
生成溯源树,研究论文发展脉络
Chat Paper
正在生成论文摘要