Local Weighted Neighborhood Rough Sets for Weakly Labeled Data
2023 International Conference on Machine Learning and Cybernetics (ICMLC)(2023)
摘要
Local rough set models are efficient rough data analysis methods for weakly labeled data in the rough set field. However, the existing local models do not distinguish the importance of different attributes to decisions when describing and defining binary relations, resulting in inaccurate information granules or classes, which affects the accuracy of concept approximation. In this paper, we choose local neighborhood rough sets as the representative model, and then propose Local Weighted Neighborhood Rough Sets (LWNRS). First, the importance of attributes to decisions is quantified by combining the supervised information of labeled data and the structural information of unlabeled data, and an attribute weighted method based on semi-supervised dynamic optimization is proposed. Meanwhile, the weighted neighborhood relation and neighborhood classes of objects are defined under weakly labeled data, and the WLNRS model is constructed. In addition, the weighted dependency is proposed to measure the data uncertainty, and the relationships among WLNRS and other relevant local and global models are deeply explored. Finally, the efficiency and accuracy of WLNRS in concept approximation are verified by comparison.
更多查看译文
关键词
Local neighborhood rough sets,Weighted attributes,Concept approximations,Weakly labeled data
AI 理解论文
溯源树
样例
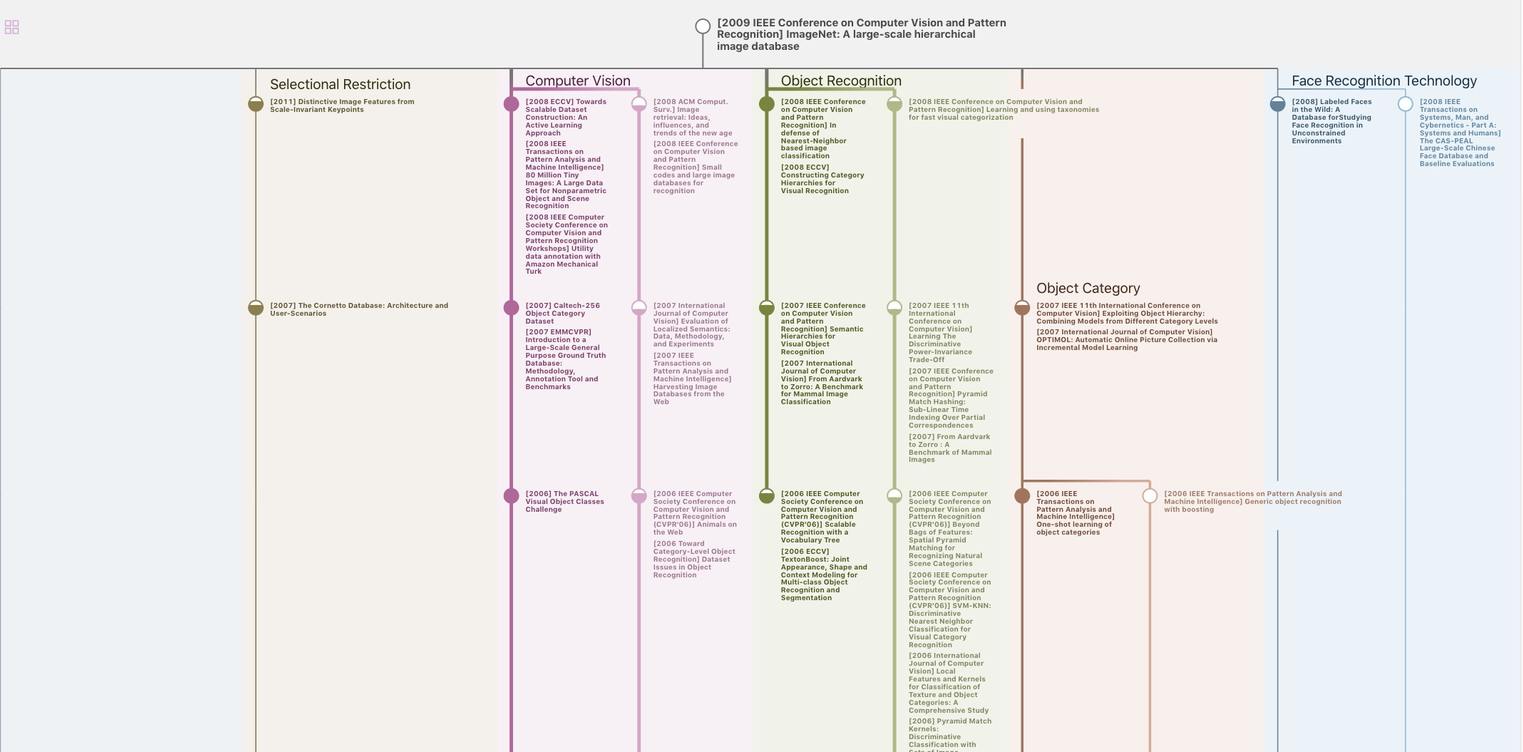
生成溯源树,研究论文发展脉络
Chat Paper
正在生成论文摘要