Deep Graph Representation Learning to Solve Vehicle Routing Problem
2023 International Conference on Machine Learning and Cybernetics (ICMLC)(2023)
摘要
The performance of a neural network relies on the depth of a model to learn the structural correlations of the features. Nevertheless, Graph Neural Networks (GNN) tends to lose its efficiency as the depth increases. In this paper we propose a technique to alleviate this problem by building on the existing GNN architecture. In effect, we installed a gating mechanism to overcome the propagation of noise information across the layers and trained the model using a proximal policy optimization (PPO), a policy gradient-based reinforcement learning algorithm. We trained the proposed model on a capacitated vehicle routing problem (CVRP) datasets generated on the fly. We used an encoder-decoder framework where the encoder learns the representation of the graph structured CVRP instance and the decoder learns to construct an optimal route based on the reward function designed. According to experiments using randomly generated test instances, the proposed model produces better results than the current deep reinforcement learning (DRL) methods to solve CVRP. To confirm the performance of our model, we also tested using locally generated real-world data parsed from digital maps. The results affirms that our model performs well in both random instance testing and real-world instance testing.
更多查看译文
关键词
Graph representation learning,Gating mechanism,Vehicle routing problem
AI 理解论文
溯源树
样例
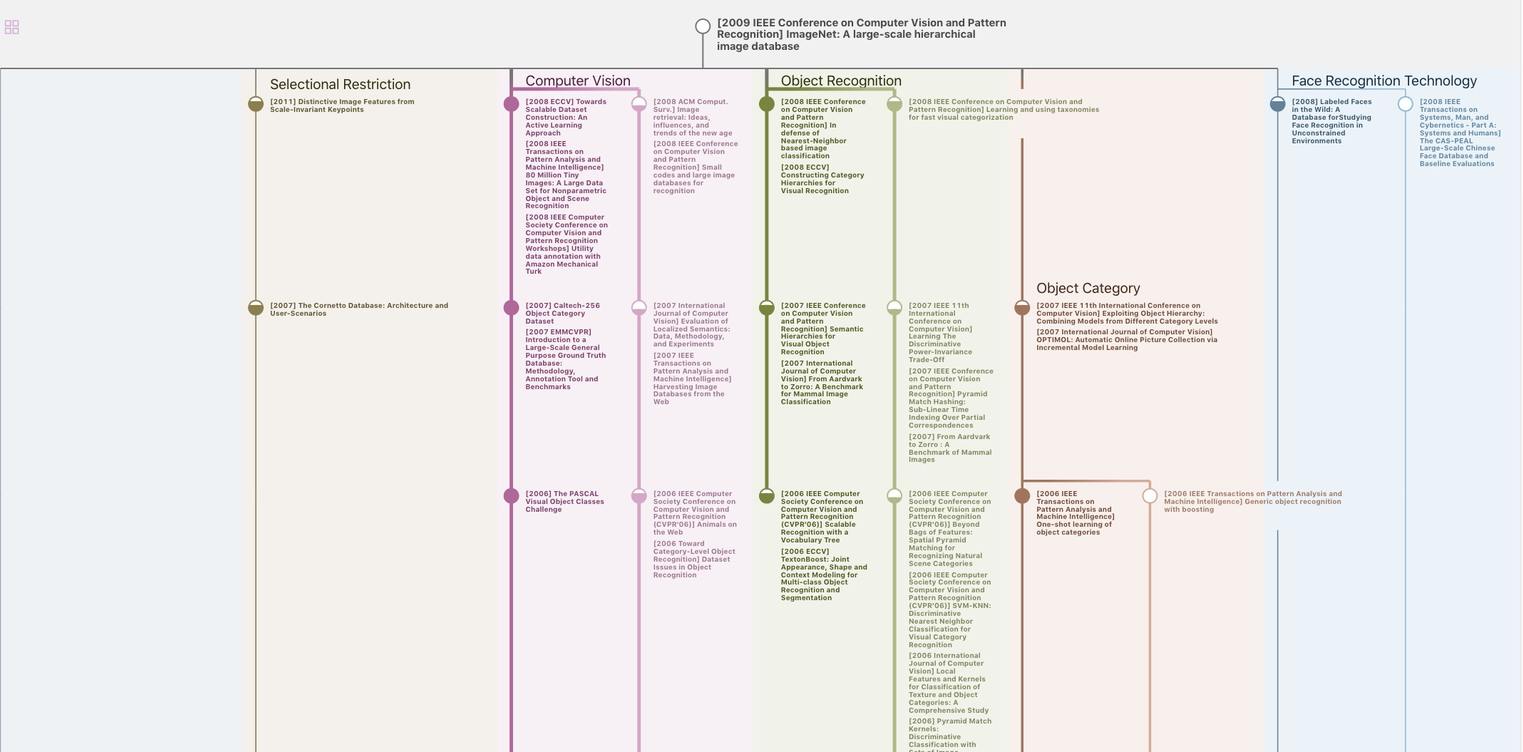
生成溯源树,研究论文发展脉络
Chat Paper
正在生成论文摘要