An Automatic Solution in Security Inspection
2023 7th International Conference on Machine Vision and Information Technology (CMVIT)(2023)
摘要
In this paper, we present a brand new dataset named cellphone buttery defects in X-ray(CBDx). CBDx consists of 300 X-ray images and 250 of them are anomaly free. We name them ‘good’. Others have some defects in the area of buttery. We name them ‘anomaly’, as Fig. 1. It raises a new task of detecting anomaly defects of cellphone butteries. But the challenge is how to distinguish the anomaly in the case of only training the ‘good’ cellphone. We define this task as an anomaly detection task. We propose an approach to deal with the task from the perspective of unbalanced classification. Specifically, we propose a data augmentation strategy that creates an anomaly sample mimic to the real defects. It helps the classifier to learn self-supervised deep representations and then make it an one-class classifier based on the representations. The classifier is well designed to discriminate the defect samples from the good ones. We evaluate different data augmentation strategies on CBDx. Our approach is more significant in this scenario with no defect training samples, which can be applied in real-world security inspection someday. Also, it can be used on the industrial texture anomaly detection, such as MVTec_AD.
更多查看译文
关键词
anomaly detection,data augmentation,self-supervised classification
AI 理解论文
溯源树
样例
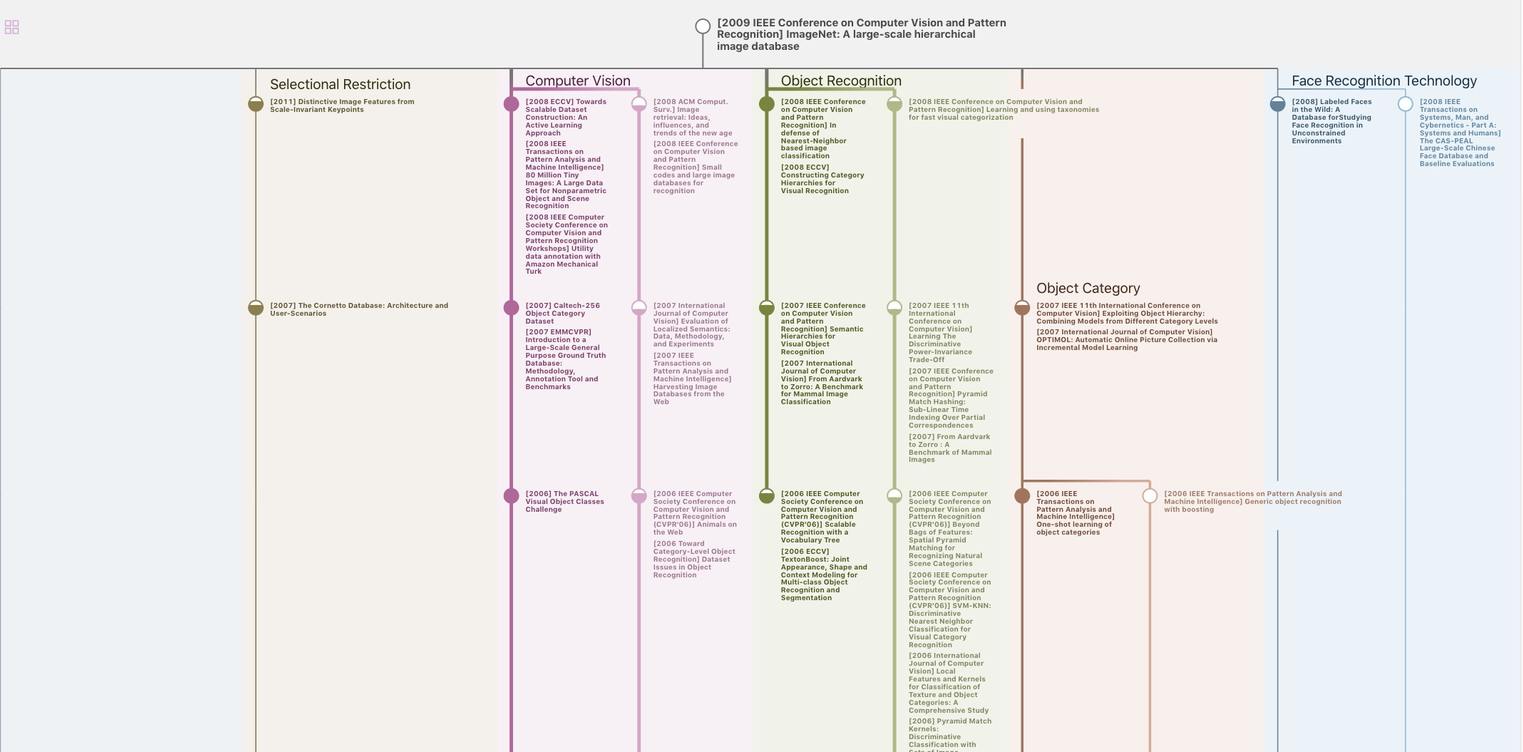
生成溯源树,研究论文发展脉络
Chat Paper
正在生成论文摘要