Federated Learning Support for Cybersecurity: Fundamentals, Applications, and Opportunities
2023 International Conference on Intelligent Computing, Communication, Networking and Services (ICCNS)(2023)
摘要
The term “Federated Learning” (FL) refers to a modern and advanced intelligence system that uses data storage that is not centralised. Most industrialists are hesitant to use the Internet of Everything (IoT) technology since cyberattacks are common and occur in numerous real-time applications around the globe. To deal with this problem, FL can be used to prevent such cyberattacks by offering better cybersecurity. This research intends to fill in some of the gaps between where federated AI is now and where it can be widely used by doing a thorough investigation of FL’s security and privacy features. We provide an informative description of techniques and different implementation styles, while also examining the current difficulties in FL, and we establish a complete evaluation of security and privacy problems that must be taken into account. Our research shows that the privacy risks connected with FL are lower than the security risks. While inference-based attacks pose the greatest risk to FL’s privacy, transmission difficulties, toxicity, and backdoor breaches pose the greatest risk to security. In the final section of the paper, we outline key areas for future study that will help FL adapt to real-world settings.
更多查看译文
关键词
cybersecurity,machine learning,federated learning
AI 理解论文
溯源树
样例
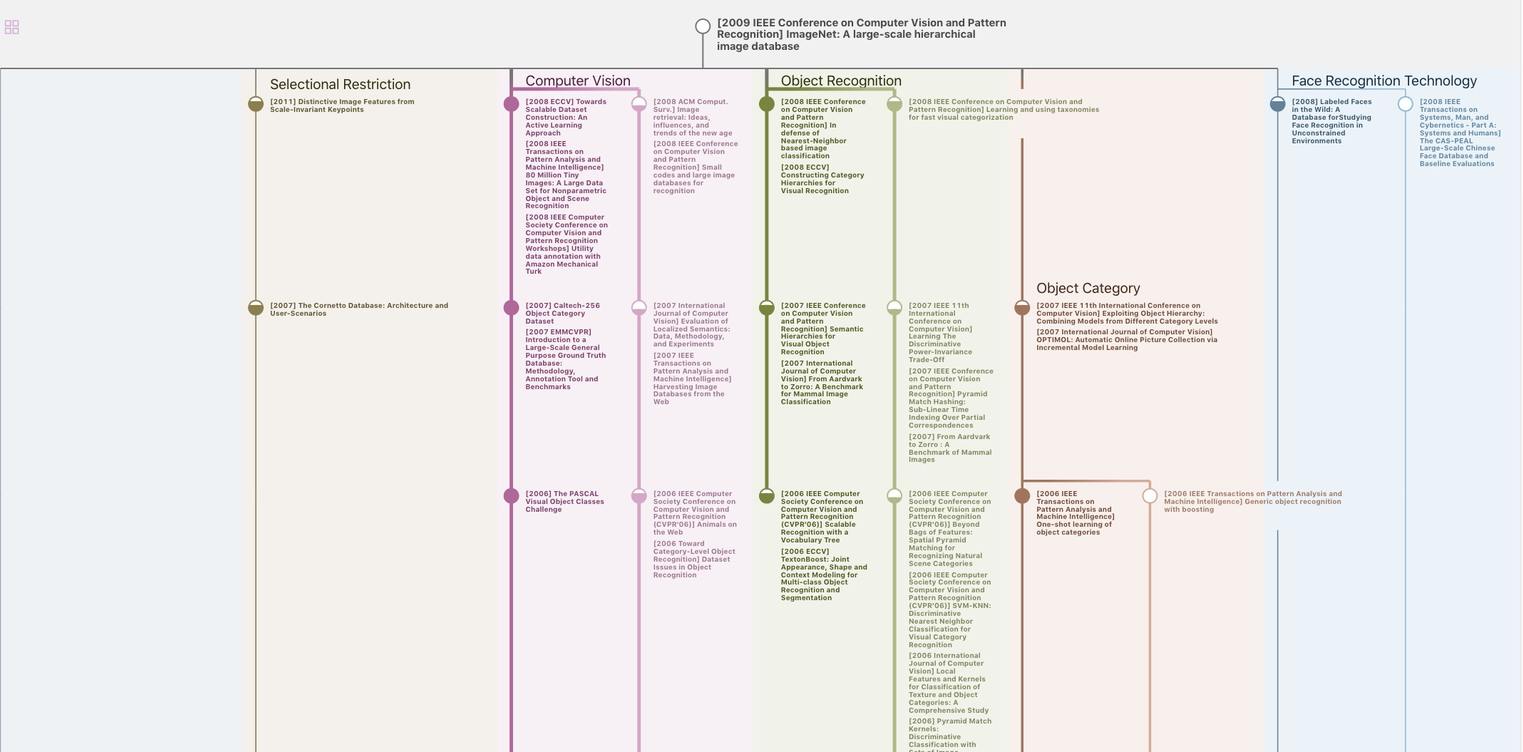
生成溯源树,研究论文发展脉络
Chat Paper
正在生成论文摘要