Data-driven FDI Attacks: A Stealthy Approach to Subvert SVM Detectors in Power System
2023 IEEE Kansas Power and Energy Conference (KPEC)(2023)
摘要
False Data Injection (FDI) attacks can mislead the grid operator’s state estimation without being detected by the Chi-2 detector in bad data detection. While machine learning methods have been used to detect FDI attacks, existing FDI construction methods only consider the Chi-2 detector and not machine learning (ML) detectors. This paper investigates the limitation of a support vector machine (SVM) detector in detecting FDI attacks. The study proposes a novel data-driven FDI (DFDI) attack that aims to remain undetected by the SVM detector and have a significant impact on the power system operation. The DFDI attack uses the minimum-area ellipse enclosing (MEE) method to obtain the boundary of the historical measurements’ cluster, which is used as a constraint to ensure that the compromised measurements are projected into the cluster. Numerical results on the IEEE 14-bus system demonstrate the limitations of the SVM detector and drawbacks of traditional FDI (TFDI) attacks, and further verify the stealthiness of the proposed attacks against the SVM detector.
更多查看译文
关键词
False data injection attacks,machine learning,SVM,data-driven attack
AI 理解论文
溯源树
样例
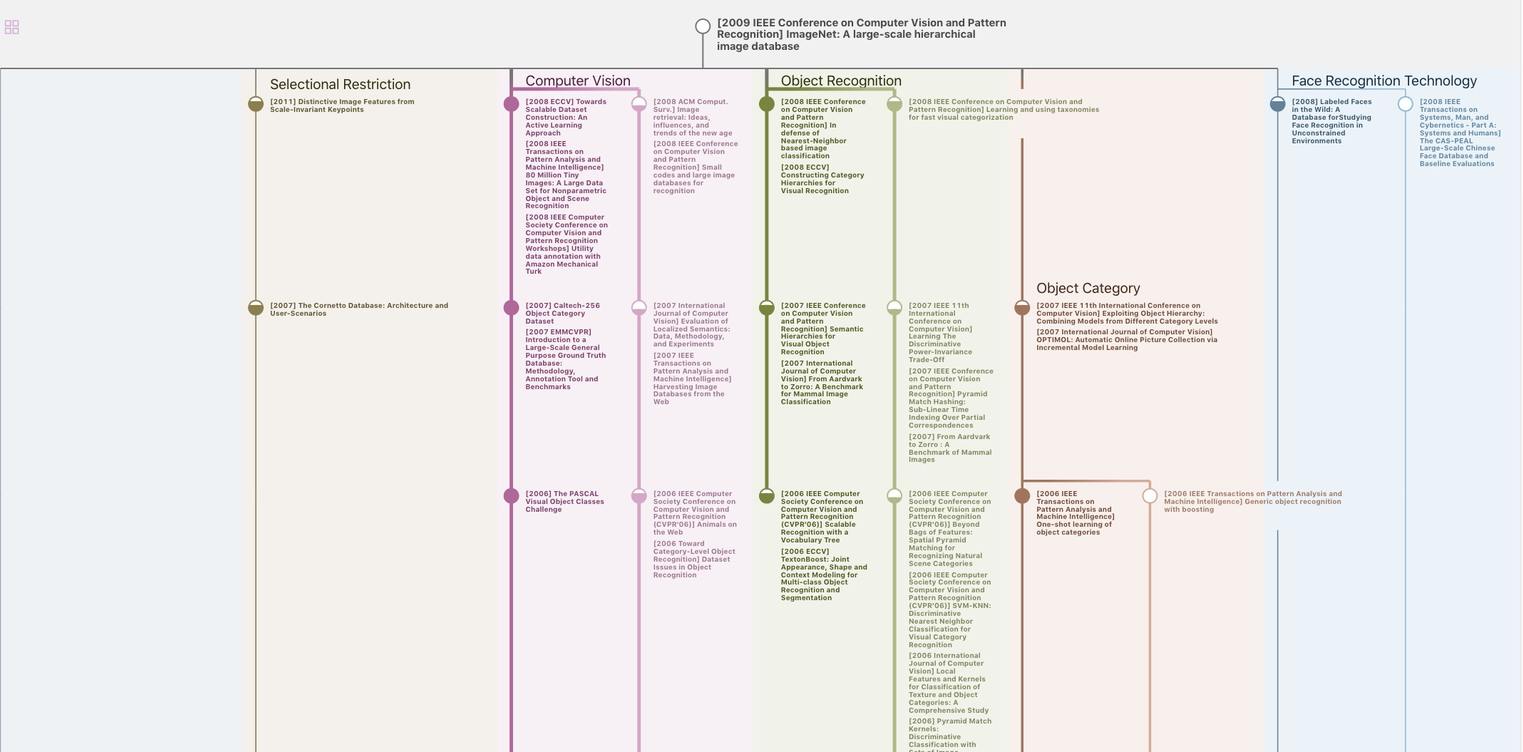
生成溯源树,研究论文发展脉络
Chat Paper
正在生成论文摘要